Flash and JavaScript are required for this feature.
Download the video from iTunes U or the Internet Archive.
Description: In this lecture, Prof. Jeff Gore asks why are some species abundant and others rare? Are there universal patterns at play? And what lead to these patterns?
Instructor: Prof. Jeff Gore
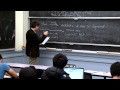
The Neutral Theory of Ecology
The following content is provided under a Creative Commons license. Your support will help MIT OpenCourseWare continue to offer high quality, educational resources for free. To make a donation or view additional materials from hundreds of MIT courses, visit MIT OpenCourseWare at ocw.mit.edu.
PROFESSOR: Today, what we want to do is talk about something at a much higher scale than what we've thought about through most of this semester. And that's probably by design. Over the course of the semester, we started with kind of enzyme kinetics or molecular binding kind of events, and we slowly built our way up the larger and larger scales.
Now there's always this question about whether we're claiming that we really understand how the higher levels of organization result from the lower level interactions. And I'd say, we definitely don't understand all of it. So you shouldn't come away with that as the notion.
But at least one thing that I think is fascinating about this area of systems biology is that much of the framework that we use to understand, let's say, molecular scale interactions or stochastic gene expression, so these dynamics at the smaller scale, much of those ideas and such certainly transport up to these higher scales or translate up to the higher scales, where, in this case, we're using kind of master equation type formulas to try to understand relative species abundance.
And so I think part of what I like about this topic of neutral theory versus niche theory and so forth in ecology is that you can just see how very, very similar ideas, that we applied for studying stochastic gene expression, can also be used to try to understand why it is that some species are more common than others when you go and you count them, in this case, on an island in Panama.
Now, the subject is, by its nature, less experimentally focused than much of what we've done over the course the semester. And this is really a topic the tends to be a combination of mathematical theory with kind of careful counting of species in some different areas and trying to understand what that means.
But it's an area that there have been a number of physicists involved in over the last 10 years. And I think that it's fascinating, because it does get to the heart of what we are looking for from a theory, what kind of evidence do we use to support a theory or to refute it.
So I think there are a lot of very basic issues about science that come up when we start thinking about this question of neutral theory in ecology. And since it's, for many of us, a totally new area that we don't know very much about, you can come to it with maybe fresh eyes. And you don't have the same preconceptions that you would have for many other models that you might be more familiar with in the context of molecular cell biology.
So the basic question that we're going to try to talk about today is just the question of why is it that, when you look out at the world, you see that there are some species that seem to be abundant and some that seem to be rare?
Are there other patterns that are somehow universal? And what kind of sort of lower scale processes might lead to the patterns that we observe?
And I think that this paper that we read is-- I mean, it's not that it's. Well, can somebody say what the actual scientific contribution of this paper was? Yes?
AUDIENCE: They did a calculation.
PROFESSOR: They did a calculation. But it's a little bit more specific than that. What is it?
AUDIENCE: They came up with the closed form equation?
PROFESSOR: That's right. Basically, there was a model of this neutral theory in ecology that we're going to explain or try to understand. You can simulate the model, but then there are possible issues associated with convergence or something of those. Although it's hard to believe that that's really such a concern. But you can simulate that model.
What they did is they just showed that you could get an analytic-y kind of expression for it. It's not a super analytic expression, but, at least, it's not a straight up simulation. You kind of numerically do something, integrate something, as compared to doing the stochastic simulation.
So it's not that that, in and of itself, is what you feel like-- it's not what we necessarily care so much about. But I think that it's still just a nice, short description of the model and the assumptions that go into it. And you get a little bit of a window into the debate that's going on between these two communities of kind of the neutral theory guys and the niche theory community.
So there's only one figure in this paper. And it's an example of the kind of data that we want to try to understand. So there's a particular pattern in terms of the relative species abundance. And we want to understand what kind of models might lead to that observed pattern.
But given that there's just one figure in the paper, we have to make sure that we understand exactly what is being plotted. And what I've found from experience-- and, actually, even the answer to the email question that was sent out, I think, was incorrect on one of these things. So we'll talk about that some more. So beware. We'll figure it out.
But I think it's actually surprisingly tricky to understand what this figure is saying. But first of all, can somebody describe not what the figure is saying but just what the data is supposed to be?
Where do they get the data? Anything that's useful?
AUDIENCE: They were on an island ecosystem.
PROFESSOR: There's an island. It's called BCI, Barro Colorado Island.
AUDIENCE: [INAUDIBLE].
PROFESSOR: So it's a 50 hectare plot. Does anybody know what a hectare is?
AUDIENCE: It's a lot more than a square meter.
PROFESSOR: It's a lot more than a square meter, yes, indeed. Yeah. Is this an English unit of measure? This is the kind of thing that I have to Google. But it's one hectare is equal to 10 to the 4 meters squared. That's a good thing to memorize. I
AUDIENCE: Exactly or approximate?
PROFESSOR: I think it's exact. I think I think it's an exact.
AUDIENCE: Then it's a metric unit.
PROFESSOR: Yeah, so apparently it is a metric unit. So the idea is that if you take a 100 meters by 100 meters, this is a hectare. And there's 50 of them. It's about like a half a square kilometer to give you a sense of what we're talking about.
And what do they do on this plot?
AUDIENCE: They count a certain number as canopy trees. So the trees that are, like, really big.
PROFESSOR: And how do they decide which trees to count? Did they count every tree?
AUDIENCE: No, just the ones that like formed the top layer.
PROFESSOR: I think that the way that they decide-- OK. Does anybody remember how many trees were counted?
AUDIENCE: [INAUDIBLE].
PROFESSOR: So there are 21,457 trees in this 50 hectare plot. They identify the species for each one of these 21,000 trees. And they assign them. And they found that there were 225 distinct species.
So this is really quite an amazing data set. Because I can tell you that I would not be able to do this. This was highly skilled biologists that can distinguish 225. If they can identify these 225, that means they have to be able to identify other ones as well. And they did it for 20,000 trees.
And indeed, Barro Colorado Island is one of the major Smithsonian research institutes, where they've been tracking. They do this like every five years or so, where they do a census, where they count all of the trees. And they're also tracking many other-- it's not just trees. They're doing everything there.
AUDIENCE: Is there only plants?
PROFESSOR: What's that?
AUDIENCE: Is it only plants?
PROFESSOR: No. So actually, I visited BCI, and it seemed like they were studying all sorts of things. And there were nice looking birds there.
AUDIENCE: No, I mean in this census.
PROFESSOR: In this census, it's only trees. And the way that they decide which of the trees to do, it's the ones that are more than 10 centimeters DBH. Anybody can guess what DBH might mean?
It's actually diameter at breast height. So what they do is they walk up to the tree with a ruler, and then, if it's larger than 10 centimeters, then they count it. You need to have some threshold at the lower end, otherwise you're in trouble, right? And there were plenty of trees that satisfied this requirement here.
Then what they do, for all of these trees, it's assigned to some species. The basic goal of this branch of biology or ecology is to try to understand the pattern, from this sort of data, where it comes from. Or first describe it, and then once you have a description of it, then you can try to understand what microscale processes might lead to the pattern.
And the pattern is what's plotted in figure 1. It's the only figure in the paper. I have reconstructed a rough version of it, here, for you on the board. But if you want a more accurate version, you can look at your paper.
Now, we want to make sure that we understand what the figure is saying. So we will ask the following question. What is the most common number of individuals for a species in this data set? The most common/frequent number of individuals for a species to have in this data set.
Now, it's maybe worth just saying something a little bit more. So you notice that they were not trying to count the total number of species, altogether. And in general, all of this field of relative species abundance, to try to understand them, what you do is typically take one trophic level.
So some of the classic studies were of beetles in the Thames River. The idea is that it's some set of species that you think are going to be interacting, maybe competing, with each other, in some way, in the sense that they're maybe eating related things and being eaten by related things.
And so in this case, these are the trees in Barro Colorado Island. And you can imagine that this is useful. The fact that it's trees instead of something else means that you can actually track the individuals over time. And when you go to the island what you see is that all the trees, they're wrapped by some tag. And presumably, they have some system to tell you which species that is so that they keep records of everything.
But the question is, what's the most common number of individuals for species in the data set? Do you understand what I'm trying to ask? And we're going do approximate, so we'll say. Or this, can't determine.
We want to know, what is the mode of this distribution of the number of individuals for each of these species? Do you understand the question? I'm going to give you 20 seconds to look at this.
AUDIENCE: Should we just hold a blank piece of paper?
PROFESSOR: Oh, we don't have our-- ah.
AUDIENCE: [INAUDIBLE]?
PROFESSOR: You know, the TA always lets me down. All right, yeah. So you can do A, B, C, D, E. Are we ready?
AUDIENCE: [INAUDIBLE]?
PROFESSOR: You can just do this if you're not. But given this was the only figure in the paper, and that this is a basic property of the distribution, I'm sure that you figured that out last night, anyways, right? Especially since it was one of the questions in the [INAUDIBLE]. So you presumably already thought about this question, right?
OK. Yes?
AUDIENCE: Yes.
PROFESSOR: Ready, three, two, one. I'd say we got a lot of B's. So it seems like B is the most. So this, we'll put a question mark here. Can somebody verbally say why their neighbor said that the mode of the distribution is around 30? Yeah?
AUDIENCE: The tallest bar.
PROFESSOR: The tallest bar there is around 30. That's a very practical definition. So that's normally what we mean by the mode. There is a slight problem in all of this, which is that this thing is plotted in a very kind of funny way.
So if you look at the figure, what you'll see is that it's number of individuals. And down here, it says, log2 scale. Now, when we say the mode, what we're wondering about is that, if you just take the most typical kind of species of tree that's there, how many individuals do we think there should be there?
Of course, typical is hard to define. We can talk about mode, median, mean, et cetera. But the most common number of individuals for a species of the data set ends up not being 30. It ends up being 1. And we will try to reconstruct this right now.
Because you have to do a little bit of digging to figure out what is being plotted here. But it's not the raw data. The problem here is that this is on this log scale, where the bins here are growing kind of geometrically or exponentially, whatever, as you move to the right.
So over here, this thing only contains one real bin. And actually, we're about to find it's half a bin, which is even weirder. Whereas out here, this is maybe 30 bins. So the number of species that we're going to put in this bin is everything between around 20 something up to 50 or so. The number of kind of true bins that end up in each of these plotted bins is going to grow geometrically as we move to the right.
So this is a very funny transform of the data. And indeed, I think it's always nice to just, in life, you always plot the raw data first. And then what you can do is then you can do funny. There's a reason to plot it this way.
Because this is where they get this idea that this might described as described as a log normal. The idea is, if you take a log of the data, then you get something that looks like a normal. But you always plot the raw data first.
So let's try to figure out what the raw data looked like. And now what we're going to do is we're going to have real scalings, honest to goodness numbers. Now the number of species you get still. So this is asking, how many different species do we see with one member or with two members or with three, four, et cetera?
And I don't know how far we're actually going to be able to get. But in this one figure, in our paper, they tell us what the histogram means. So the first histogram bar represents what they call phi 1 divided by 2. Phi 1 was the number of species observed with one member, which means that even this first plot bar is not the number of species observed with a single individual. It's half of that.
You can argue about the consistency of how these things should be, but that's what this thing's plotted. And it looks like it was nine, here, so this should be 18. So I'm going to put up here, here's a 20 and here's a 10. Right, so here is an 18.
Now, what do they say? This bin represents phi 1 divided by 2 plus phi 2 divided by 2. So they took the number of species where they saw just a single individual plus the number of species where they saw two individuals, and they added those and they divided by 2. That's this number.
We're not going to go through this whole process, because it's a little bit tiresome. But I've already done it for you. So I'm going to plot a few of things to get you there.
And so I calculated it was 19, 13, 9, 6. It becomes ill-determined once you get out here, in the sense that we don't have enough. It's not uniquely specified going from that to that as it has to be.
But I calculated it. It's around 5, in here, for a few. And somewhere in here, it's going to go into 4. And then this might go down to 3, and then deh, deh, deh.
Now, if you look at this and the rapid rapid fall-off, do you think that you're going to find any species that have more than 20 individuals? We're going to vote. So you see this falling-off?
So let's say that I've just showed you this, and I haven't yet calculated the rest, do we think that there's going to be any species with more than 20 individuals? Greater than 20 individuals, question mark? 1 is yes. 2 is no.
It's going to be yes, no. Ready, three, two, one. So we got some 2s. So I'd say that most people are saying, no. Look at this fall-off. They're not going to be any species with more than 20 individuals.
Although we already know that there are many species with more than 20 individuals. So this plot is useful for something. You can see that there are. And we know exactly the number of species that have more than 20 individuals, roughly. So those ones are all in these.
So you can see that there are hundreds of species with more than 20 individuals. And indeed, it looks like there were two or three species that had more than 1,000 individuals or 1,500 or whatever the cutoff there was.
So this distribution starts out rather high but then falls quickly. And out here, it's going to be very, very sparse. So there's going to be a bunch of numbers in here where there's not any species in the histogram. And then out there, there's going to be one, right?
And indeed, you have to go really far out. Because there's one species out there that has a couple thousand. And indeed, the mean number of individuals per species has to be around 100. We know how to calculate a mean. This divided by this is just short of 100.
So the mean number of individuals in a species is around 100. The mode is one. And the median? Well, ready? We decided this was the mode. Where is the median going to be? Is it going to be A, B, C, D? Ready, three, two, one.
Indeed, this tells you pretty clear where the median is. This thing is indeed around the median. Because you can say, oh, it's about the same numbers to either side. So the median is around here. And I told you where the mean was, again.
You guys remember? Ready, three, two, one. Mean, uno. Mean. So this is a very, very funny distribution. I guess I want to highlight that. And I think it's not at all what you would have expected somehow.
At least, if you had described this measurement process to me, if you told me that you went to this island and you counted 20,000 trees, I don't know how many species I would have guessed. But OK, 220, it's reasonable.
Well, I would have guessed it would have looked something like this on a linear scale, maybe, right? You know, that there would be a bunch of them around 50 to 100 and some would go couple hundred, some of them. So I guess I would have thought that the mean, mode, median would all be kind of a more similar thing.
But this is just not the way the world is. It's not just on BCI. People, for hundreds of years, have been studying these distributions. And things that look like this, with extremely long tails, this is what people see.
Now you can argue about exactly how fast it falls off and whether it's different on a mainland or an island. But this basic feature, that rare species are common, this seems to be just that's what you always see. This is the thing that you have to remember, rare species are common.
And I think that this is the basic, surprising thing in this whole field. And the ironic thing is that even after spending all this time reading about theories to describe these distributions, it's still very possible-- and I would say, based on the statistics, this year and past years, it's not just possible, but it is the standard outcome-- is that after reading this paper, you do not realize that the distribution looks like this.
You somehow still think that it looks-- you kind of still think it's like a linear scale, where the typical species has this, where the mean, median, mode are all about the same thing. So I guess always plot the raw data in an untransformed way. There are theoretical reasons why it might be nice to plot it like this. But be very careful about what you're doing. Because then you're left with a mental image of a histogram that looks like this. And that's very, very dangerous. Yeah?
AUDIENCE: Why does it matter [INAUDIBLE]? [INAUDIBLE] the aggregate data in bins like that. And I mean, sure, exactly one species is the mode, but do you really want the--?
PROFESSOR: I understand what you're saying. It's just that there's a qualitative aspect to the data, which is that most species are very rare. And this is something that I think is surprising. I think it's deep. And it's something that you do not get realized.
AUDIENCE: Most species have more than 16. I mean, it depends what you mean by rare.
PROFESSOR: Yeah.
AUDIENCE: Look at the way that the distribution is away from trend.
AUDIENCE: That's a good point. But the species density is clustered around the low numbers.
PROFESSOR: Right.
AUDIENCE: But actually most species have more than 30.
PROFESSOR: Maybe the surprising thing is that just if you take-- the mean is 100. And so I would've thought that, if you plot number of species as a function of the number of individuals, given those numbers, I would have guessed, OK, here's 100.
I would have guessed-- here's 50, so just to highlight that this is 150. So linear scale, I would have guessed it would look something like that, maybe larger than Rudin or something.
AUDIENCE: What would that look like in a log2 scale? It would look like It's like the log of [INAUDIBLE]? So it goes up really fast and then--
PROFESSOR: So this thing would be kind of like shoom. I mean all the weight would be in. It would be like all here plus a little bit on each of these.
AUDIENCE: But yeah. I don't think it's actually that different. The only thing that's different is the tail on the left.
PROFESSOR: And the tail on the right.
AUDIENCE: Yeah, it's a little bit longer.
PROFESSOR: No, it's lot longer, right? Because this thing, all of the weight is between 50 and 150, which means that all of the counts are basically going to be these two, basically. Because this thing comes out either way.
So in this case, if you take that histogram put it on this kind of scale, you end up with two bars up high, nothing outside. So it's a very different distribution.
And it's not to say that this is a ridiculous thing to do. It's just that. But the problem is that your mental image of what the distribution looks like ends up being incorrect, in the sense that you have a qualitatively different sense of what's of what's going on. And if you go up to 10 species, here, and 10 is way down here.
If this is what it looked like, there would be essentially no species with fewer than 10 individuals. But if you come over here and you add it up here. It's like a mean of 6 times 10 is 60 out of 200. A quarter or a third of the species on this plot of land have fewer than 10 individuals. And 10 is really a very small number.
Well, rare species are common. I think it's a true description of the observed distribution here and elsewhere. And it's not something that you appreciate or realize when you plot it in that way.
AUDIENCE: But you can get this information from that plot.
PROFESSOR: No, I agree. You can get it. You can get it. But it was only 10% of the group got it. Right, the fact that you can get it-- right, it's possible. But you don't get it. That is a practical statement. Yeah, I'm not dead set against this distribution. It's just that it makes everybody think something that's not true.
So if you think that that's OK, then I can't help you. It's OK, but it's just you have to be careful is my only statement. And I very much want you to take away. Because I this is an accurate description of the data. Rare species are common.
And one of the readings-- I think it was in this paper, maybe it was a different one that I was reading. Even Darwin, when talking about this, commented on this fact that rarity of species is somehow a typical event.
AUDIENCE: And common species are rare.
PROFESSOR: And common species are rare, that's right. This distribution is hugely, hugely skewed. These are the measurements. It's good to look at them in both of these ways. Because you can't even plot the data on a linear scale. So that's a good reason for doing it. But I think it's good to have both of these pictures in mind.
What we want to do is to talk about two classes of models that give something that's essentially this log normal distribution. So on a log scale it looks normally distributed, approximately. And those two models are going to be kind of a niche-based model and a neutral model.
Can somebody, in words, explain what they maybe see as the difference between this niche and a neutral kind of approach? Yeah?
AUDIENCE: [INAUDIBLE].
PROFESSOR: Every species is--?
AUDIENCE: [INAUDIBLE].
PROFESSOR: In which one?
AUDIENCE: In niche.
PROFESSOR: In the niche theory, the species are different. So it seems like a ridiculous statement. Do you believe that species are different? We can vote, yes or no. Ready, three, two, one. Yeah. Well, somebody's been convinced by the neutral theory.
It's clear that species are different. And the question is which patterns in the data do you need to invoke differences in order to explain?
And I think that one, maybe, theme that's come out of this relative species abundance literature and the debates between the neutral and the niche guys is just that this distribution is less informative of the micro scale or individual kind of interactions then you might have thought.
Because multiple models can adequately explain such a pattern. In all areas, we have to remember that you make an observation, and you write down a model that explains that observation. So what you do is you write down a model.
And writing down a model, what that means is that you make some set of assumptions. And then you look to see what happens in that model. And if the model is consistent with the data, that's good. But it doesn't prove that the assumptions that went into the model are correct.
And this is a trivial statement. And I've said it before. You have to tell yourself this or remind yourself of this kind of once a month. Because it's just such an easy thing to forget about.
Now, the niche models indeed assume that the species are different. And that's reasonable. Because we think it's true. But then, of course, there are many different ways of capturing those differences. And then you have to decide whether the assumptions there are reasonable or whether they're necessary, essential.
In the context of the niche models, we're going to think about the so-called broken stick models. So basically, you get log normal distributions when there's some sort of multiplicative-type random process that's being added together.
You get normal distributions when you have sums of random things going together. This is the central limit theorem. But when you have multiplicative kind of errors or random processes coming together, you get log normal distributions.
And I want to highlight that that does not necessarily have to tell you so much about the biology of it. Because a classic situation where you get log normal distributions is if you take a stone and you crush it.
You can do this experiment at home. And then you measure the mass distribution of the resulting fragments. And the distribution of mass is log normal. Just take a stone, grind it under your boot or hammer it, just kind rub it right in. You'll get you'll get some distribution of fragments.
For each of the fragments, measure the mass, and, indeed, you end up getting a log normal distribution. Because there's some sense that what's happening is that you take a larger mass, you break it up randomly, and then the resulting fragments, at some rate, each of them you break up randomly. and the small ones are maybe kind of less likely to get broken up as the big ones, so then the small ones can still get even smaller.
But then there's going to be, at some rate, some very large ones. So such a process ends up-- I mean it's not biology. This is just something about the nature of the breaking up of this physical object. And indeed, the basic idea behind many of the niche models that give you a log normal distribution is equivalent to crushing a stone and measuring the resulting distribution.
I'll describe what I mean by that. Typically, the broken stick models, they say there's some resource axis. This is a resource axis. And this could be, for example, where you're getting food from.
Now, we're going to have to divide up this resource access among some number of different species. And what we're going to assume is that the number of individuals in the species is proportional to the length of the resource axis that it's able to capture. And I want to make sure I find my notes.
I want to highlight this. This comes from MacArthur in the 1950s. MacArthur and it's 1957. So we imagine there's this homogeneous resource axis. We're going to break it up into N segments. And the abundances are proportional to the length.
And the idea is that, if you just break this up randomly, so let's say you just draw N minus 1 lines randomly, or N minus 1 points randomly here. Now you have N species with N different abundances.
The question is does that give a log normal? We'll say N minus 1 random points. Do you understand what I mean. You sample uniformly once, sample uniformly twice. You do that N minus 1 times, and now you have N and deh deh.
And then we say, OK, the first species has this many individuals. The second has this one. The third is this one, et cetera. The question is does random points, does that lead to a log normal?
Yes and no. Let's think about this for 10 seconds. N minus 1 random points, log normal distribution, ready, three, two, one. So I'd say that we have a majority are saying no. Can somebody say why that is?
AUDIENCE: [INAUDIBLE].
PROFESSOR: Because it's something else. That's fair. But can you say qualitatively why it is that this is not going to work?
AUDIENCE: You can't have very long gaps.
PROFESSOR: Right. That is it's going to be very unusual that you get a very long gap. What about the other end?
AUDIENCE: Also a very long tail.
PROFESSOR: Now I'm a little bit worried. I think that that's true, right? Well, I'm going to say that you're not going to get this super long ones. I think that the distribution might still be peaked at short values. No?
AUDIENCE: No.
PROFESSOR: Random? If we were just traveling along this resource axis, at a rate that's kind of exponentially distributed, like Poisson rate, we just dropped points, that's something very similar to this random--
AUDIENCE: It said we're limited in the number--
PROFESSOR: No. Is that not true?
AUDIENCE: Your sample [INAUDIBLE].
PROFESSOR: I'm a little bit worried that I might be-- now, I'm not 100% confident. Depending on how I look at this, I get different distributions. Yeah?
AUDIENCE: But I think the first thing that he said, where you just say, I'm going to pick N minus 1 points--
PROFESSOR: Yes.
AUDIENCE: --is a different thing than going along the axis and exponentially dropping ones along.
PROFESSOR: I agree it's different.
AUDIENCE: I don't think that would be the idea simulated, because you would be very likely to just get this giant thing at the end when you're finished.
AUDIENCE: What you could do, you could go on to draft N plus 2 points.
PROFESSOR: No, I think--
AUDIENCE: These scales that are your two end points [? are doubled. ?]
PROFESSOR: Because I think that the probability distribution does grow. I think that I'm going to side with you. So we've decided that there are not going to be as many short sticks, and there's not going to be as long sticks as compared to a log normal. Do we agree with that?
At least we agree that it's not going to be a log normal. So you're not going to get this huge variation of some very long sticks and some very short ones. Now, the question is how would you change this sort of model in order to generate a log normal?
And the answer is that what you have to do is you have to what is called some niche hierarchy or so some hierarchical breaking. Just like what led to the stone giving you a log normal is that you have to have some successive process of breaking things. So this is what they call some hierarchy model.
And then they key thing is that it's sequential. You have your resource axis. First, you have some rule for breaking it up. It could be that you just sample uniformly or some other probability distribution.
And the way that you might think about this is via-- just everything up on the board is so nice and useful. I feel bad getting rid of it. This thing is not true, so I don't mind erasing it.
So let's imagine some bird community in the forest. And we're going to think about where is it that the birds are getting their grub or their food to eat.
First, well, now the axis is somehow vertical. You could divide them up into the ground foragers as compared to the tree foragers in terms of where they're getting their food.
And you say, oh, well, how much of the food is on each side? Oh, well, we'll say 30% is on the ground, 70% is on the tree. This is along the stick. You cut the stick in some way, or you break the stick in some way.
But then within the tree foragers, you'd say, well, the resources might be separated. And this is really like speciation, a species is in the niche, the species are focusing on different niches. So you'd say, oh, some are going to focus on the trunk, some will focus on branches.
And again, this part of the stick is now broken or divided among different resource locations with some amount. But then also, you're going to get speciation in different directions here, because there's both the surface-- I don't know if you guys have ever eaten grubs-- but there's the surface grubs, and then there's also the sub-bark grubs.
And so you kind of do this process multiple times, where you kind of pick different branches and break them to divide up the niche. And then you end up with a log normal type distribution.
And this is a similar process to the crushing of the stone, because the idea is that there's sequential breaks of the stone. So the stone first breaks into maybe simply two or it could be three. First, there's one breaking. And then one of them is broken more. So given this process, you end up getting a log normal distribution. Yeah.
AUDIENCE: But you also have a distribution of like how far. Because I guess there are two questions. Like when you break your stick, you assume, somehow, that you uniformly break it.
PROFESSOR: Yeah. A lot of work has gone into the question of how it is you should break the stick. Given that you have this tree foraging stick. On a practical level, what they do is they ask, well, what probability distribution gives you the best agreement with the data? Is it uniform? Or is it, oh, it's broken like this?
And in some cases people say, well, it's actually tilted on one side. Well, in the context of a succession and some other environments, there's an idea that, if a species first gets somewhere, they can kind of monopolize a larger fraction of the resources then if it's divided kind of an equally at the beginning.
And that's going to effect where this probability distribution is going to break each one. But there's always this question about how constrained are the notions and so forth. And I'm agnostic on that point.
AUDIENCE: But you also need distribution for how many times it breaks [INAUDIBLE].
PROFESSOR: Yes. It's just that, if you do this process, it's like a central limit theorem type result. So you have to do it enough times so that you get to some limiting distribution. And then you could keep on doing it. In the end, we always say that species abundance is proportional to the size.
So we're going to scale, ultimately, to get the correct number of individuals. It's just that you have to do it some reasonable number of times so that the randomness kind of washes out, and you end up approaching that limiting behavior. Does that make sense?
And indeed I just want to mention a major result in this field. These niche type models successfully explained or predicted another pattern that had been observed, which is the so-called species area relationships.
So this is just saying that, here, we looked at 50 hectares, and we asked how many species where there. 225 species in 50 hectares. Now, the question is, if instead of looking at 50 hectares, we instead looked at 500, do you think of that the number of species we observed would have gone up, stayed the same, or gone down? Up, same, down, ready, three, two, one.
Up. Up. If you look at a larger area, you expect to see more species in a larger area. And people really do this. They look in some area, going from, say, they take a meter, and they count all the species. And then they go and here is 100 meters, and they count all the species.
And they ask, how many species do you see as a function of the area? And what people have found is that the number of species you observe it is proportional to the area to some power, where Z is around a 1/4. And of course, the area goes as some r squared. If you wanted to, you could say it goes as the square root of the radius, whatever.
But the number species in some area, it grows, but it grows in a manner that is less than linear. Does that make sense? It definitely makes sense that's less the linear.
Because linear would be that you sample a bunch of species here, and then you look at another identical plot, you get some other species. And they were saying that, oh, that you really don't expect any of those species overlap. That would be a weird world.
So it very much make sense that this is less than 1. Of course, it didn't have to be this power law. But one thing that has been discovered, around the world, is that power laws are very interesting. But once again, many different microscopic processes can lead to power laws.
The niche models have successfully predicted or explained why it might have this scaling. But it turns out that neutral models can also predict it. And may just be that lots of spatially explicit models will give you some power law type scaling that looks kind of like this.
So once again, it's a question of how convinced you should be about microscopic processes based on being able to explain some data. And I think the best cure for this danger, of assuming that the microscopic assumptions are correct, because the model is able to explain something, is that, if you find some other very different set of microscopic assumptions that also explain the patterns, then it becomes clear that you have to take everything with a grain of salt.
And that's I think part of what's been very valuable about the neutral theory contribution to this field.
AUDIENCE: Does this just come from-- you assume that all the individuals are uniformly distributed and then [INAUDIBLE]?
PROFESSOR: There are multiple derivations of this, so it's a little bit confusing. The neutral models, that I have seen, that lead to these patterns, they basically have the individuals randomly, either with sex or without sex, kind of diffusing around, and then they divide, deh-deh. And then you can explicitly just do the different spaces and see that you get a scaling.
It seems to be a surprisingly emergent feature of many of these models. And once again, it may be something that tells us less about biology than it does about math or something.
Any other questions about this, the base notion of this niche hierarchy type models? So I want to spend some time talking about this neutral theory in ecology. The math, in particular the derivation of this particular closed form solution, is not really so interesting or relevant. But I think it's very important to understand what the assumptions are in the model and maybe also something about the circumstances in which we think that it should apply.
So the basic idea is that we have, what we hope, is some metacommunity that is large. And then we have an island. So this has to do with this theory of island biogeography. We have an island over here.
And in the context of the nomenclature of this paper, they are some community size, size j here. This tells us about the number of individuals. And they're distributed across some number of species.
Now, the neutral theory, the key thing is that we assume that all individuals are identical. And once again, it's not that the neutral theorists believe that this is true. It's that they think that it may be sufficient to explain the patterns that are observed.
And when we say that all individuals are identical, what we mean is that the demographic parameters are the same, birth, death rates. And it's even a stronger assumption, in some ways, than that. It's assuming that the individuals are the same, the species are the same, and that there are no interactions within the species as well.
So there's no Alley effect, or no specific competition. So the birth, death rates are going to be independent of everything, which is an amazingly parsimonious model. And it's kind of amazing you can get anything out of it.
And then we have a migration rate m. It's either a rate or a probability, depending on how you think about it. Rate or probability m. And can somebody remind us how we handle that?
AUDIENCE: Both just in a community?
PROFESSOR: Yeah.
AUDIENCE: At some probability that is proportional to the distribution of the species in the metacommunity?
PROFESSOR: Yeah, that's right.
AUDIENCE: --transfer an individual from the metacommunity to the island.
PROFESSOR: Perfect.
AUDIENCE: We do stick to the island to make sure that number of individuals.
PROFESSOR: Right. So what we're going to do is we're basically going to pick a random individual, here, each cycle. This is kind of like a Moran process. We're going to pick an individual here. And we're going to kill him.
And then what we're going to do is, with probability m, replace that individual with one member of the metacommunity at random. So the rate coming from here will be proportional to the species abundance in the metacommunity. And with a probability of 1 minus m, what we're going to do is we're going to replace that individual with another individual in the island.
Now, the math kind of gets hairy and complicated. But the basic notion is really quite simple. You have a metacommunity distribution, which is going to end up being the so-called Fisher log series in this model. This describes the species abundance on the metacommunity.
But then on the island, we're just going to assume that there's birth, death that occurs over here at some rate. But we don't even have to hardly think about that. From the standpoint of, say, a simulation or model, we just run multiple cycles of this, where we have j individuals.
And we always have j individuals, because it's like the Moran process. At every time point, we kill one individual, and we replace it, with somebody either from the same community or from the island.
And you can imagine that in the limit of m going to zero, what's going to happen on the island? Yeah, so you'll end up just one species, just because this is just random, like genetic drift. It's ecological drift where one species will take over. Whereas if m is large, then somehow it's more of a reflection of the metacommunity.
Are there any questions about what this model is looking like for now?
AUDIENCE: Could we talk about the Fisher log series?
PROFESSOR: Yeah.
AUDIENCE: So we would put it on the same axis as the [INAUDIBLE]?
PROFESSOR: Yes, this is a very, very good question. So we'll do this in just a moment. Because this is very important. I want to say just a couple things about this model. So when I read this paper, what I imagined is that it really looked like this.
This was Panama, and that, 30 kilometers off the coast, there was this island, BCI, Barro Colorado Island. But that's not maybe an accurate description of what the real system looks like. Does anybody know where BCI is?
AUDIENCE: It's in Panama.
PROFESSOR: Hm?
AUDIENCE: Panama.
PROFESSOR: So it is in Panama. But it's not off the coast of Panama. I guess that was my original.
AUDIENCE: It's in the canal.
PROFESSOR: Yeah, it's in the canal. So it's an island that was created when they made the Panama Canal. So this thing was not always an island. It's been an island for 100 years. And it's in the middle of a canal. And they actually have cougars that swim back and forth from the mainland.
But it does make you wonder whether this is-- it's much more strongly coupled to the mainland then I imagined when I read this paper at first. I don't know what that means for all this. But certainly, you expect this to be a more or less appropriate model depending on this.
Because, of course, if you went and you sampled 50 hectares here, you wouldn't believe that it should have the same distribution. You'd believe it should be more like the Fisher log series. And there's some evidence that things are tilted in a way that you would expect. And we'll talk about that.
It's tricky. And of course, you have to decide in all this stuff, oh, what do you mean by free parameters? And actually, it seems like people can't count. And we'll talk about this in a moment, too.
Because, of course, constructing the model, there's some sense of free parameters that you have there. Because we could have said, oh, it's just going to be the Fisher log series, or we could have said, oh, it's going to be island. Or we could have said, oh, there's another island out here. And then that would be another distribution.
And not all of these things introduce more free parameters, necessarily, because you could say, oh, this is the same migration rate, or you could do something. But they are going to lead to different distributions, and you have that freedom when you're trying to explain the data. There are a lot of judgment calls in this business.
But let's talk about Fisher log series, because this is relevant. So the model is very similar to what we did for the master equation in the context of gene expression and the number of mRNA. So was the equilibrium or steady state distribution of mRNA in a cell, was that a Fisher log series? Yes or no, five seconds? Was the mRNA steady state probability distribution a Fisher log series? Ready, three, two, one.
No. No. What was it? It was a Poisson. And you guys should review what all these distributions are, when you get them, and so forth. So what was the Difference why is it that we have some probability, P0, P1, P2? This could be mRNA or it could be number of individuals in some species with some birth and death rates.
What was the key difference between the mRNA model, which led to this distribution becoming Poisson, and the model that we just studied here, where it became a Fisher log series? And I should maybe write down what the Fisher log series is.
So this is the expected number of species with n individuals on the metacommunity. Here is the Fisher log species. There was some theta X to the n divided by n. So what's the key difference? Yeah.
AUDIENCE: I think that the birth and death rates are both proportional [INAUDIBLE].
PROFESSOR: Right, the birth and death rates are both proportional.
AUDIENCE: In the Fisher log series.
PROFESSOR: In the Fisher log series. So what we have is that b0-- and what should we call b0 in this model?
AUDIENCE: [INAUDIBLE].
PROFESSOR: Well, right now, we're thinking about the metacommunity.
AUDIENCE: Speciation.
PROFESSOR: Speciation. b0 is speciation, which we're going to assume is going to be constant. In this model, do we have speciation on the island? No. The assumption is that the island is small enough that the rate of speciation is just negligible. So speciation plays a role in forming the metacommunity distribution, but it doesn't play a role in the model.
So this is speciation. But then what we assume is that b1, here, is equal to some fundamental rate b times n, but it's b times, in this case, 1. So more broadly, bn is equal to some birth rate times n. This is saying that the individuals can give birth to other individuals.
Now, we're not assuming anything about sexual reproduction necessarily or not. We're just saying that the kind of rates are proportional to the numbers. So if you have twice as many individuals, the birth rate will be twice as large. This is reasonable.
This is Pn and this is Pn plus 1. So this is d of n plus 1 is equal to some death rate times n plus 1. So each individual just has some rate of dying. It's exponentially distributed. This again makes sense.
What was the key difference between our mRNA model, from before that gave the Poisson, and this model that gives the Fisher log series?
AUDIENCE: So with the mRNA, it's with a standard like a chemical equation where there's some fixed external input. But then the degradation is according to the amount that you have. So death is proportionate [INAUDIBLE].
PROFESSOR: Perfect. In both cases, the death rate is proportional to the number of either mRNA or individuals. However, in the mRNA model, what we assume is there some just constant rate of transcription, so a constant rate, per unit time, of making more mRNA. So just because there's more mRNA doesn't mean that you're going to get more mRNA.
But here, we assume that the birth rate is proportional to the number. So that's what leads to the difference. And so this is one of the few other cases that you can simply solve the master equation and get an equilibrium distribution.
And it's the same thing we do from just always, where we say, at steady state, the probability fluxes or whatever are equal. So you get that P1 should be equal to P0. and then we have a b0 divided by d1. And more broadly, we just cycle through. The probability of being in the nth state, it's going to be some P0. And then basically, it's going to b0 divided by d1, b1 divided by d2, b2, d3, dot, dot, dot, up to bn minus 1 dn.
And indeed, if we just plug in what these things are equal to, we end up getting-- there's P0, the fundamental birth over death to the nth power. And then we just are left with a 1 over n. Because we're going to have a 2 here and a 2 here, and those cancel. A 2 here and 3 here, and those cancel. And we're just left with the n at the end, finally.
So this x, over there, is then, in this model, the ratio of the birth and death rates. So which one is larger? Is it A slash 1? Is it b is greater than d? Or is it b slash 2, that b is less than d? Think about this for five seconds. Do you think that birth rates should be larger than death rates or death rates should be larger than birth rates or do they have to equal? Ready, three, two, one.
So we got a number of-- it's kind of distributed, 1 and 2's. Well, it's maybe not that deep, not deep enough. Can somebody say why their neighbor thinks it's one or the other? People are actually turning to their neighbor. A justification for one or the other.
AUDIENCE: So if this problem where b over d is greater than 1, then this distribution is not normalized.
PROFESSOR: Right. So if b over d is greater than 1, so if x is greater than 1, then this distribution blows up. Then it gets more and more likely to have all these larger numbers. But then if b is less than d, shouldn't everybody be extinct? No.
Can somebody else say why it is that it's OK for b to be less than d? If birth rates are less than death rates, shouldn't everyone be extinct?
AUDIENCE: Because there's a rate b0.
PROFESSOR: Because there's a rate b0, exactly. So there's a finite rate of speciation. So it's true that every species will go extinct. But because we have a constant influx of new species, we end up with this distribution that's this Fisher log series.
Now, if you plot the Fisher log series, it looks a bit like this. But let's think about it a little bit. Does the Fisher log series, does it fall off, A, faster or slower than this? Fisher falls, A, faster-- this is in this direction-- or, B, slower?
AUDIENCE: Faster or slower than what?
PROFESSOR: Than the island distribution. Because you can see that this falls off pretty rapidly. Ready, maybe? Three, two, one. I saw a fair number of people that don't want to make a guess.
Indeed, it's going to be faster. Can somebody say why? Yeah.
AUDIENCE: [INAUDIBLE].
PROFESSOR: Is it going to be because of the 1 over n? I mean the 1 over n is certainly relevant. Without the one over n, then we just have sort of a geometric series. And the log normal is not just a geometric series either.
AUDIENCE: [INAUDIBLE] Whereas this has a very long tail.
PROFESSOR: That's right. So this falls off. This would be kind of exponentially, and this is faster than exponentially. And indeed, this make sense based on the model. Because this community, the reason that it has some very, very abundant species is partly because it gets migration from the abundant species here.
This falls off pretty quickly. But those frequent species still can play a pretty important role in the island community, because the migration rate is influenced by large numbers. And the other thing is, of course, that the rare species are going to often go extinct.
I mean the distribution on the island is some complicated process of the dynamics going here, plus sampling from here. But there's a sense that it's biased towards-- it's not just a reflection of the metacommunity, because the migration rate is sampled towards the abundant species.
So the migration of these species ends up playing a major role in pushing the distribution to the right. So you have much more frequent, abundant species on the island as compared to the mainland.
AUDIENCE: [INAUDIBLE]?
PROFESSOR: Yeah.
AUDIENCE: [INAUDIBLE] measurement of the distribution on the--
PROFESSOR: Well, I'm sure they have. I think the statement that there's a faster fall off on mainlands than on the islands I think is borne out by the data. But I don't know if trees on the Panama side of the canal are actually better described by a Fisher log series as compared to this, though.
AUDIENCE: I guess my question was the abundant species that we see on the island, is it just the result of diffusive drift?
PROFESSOR: Well, this also has the diffusive drift.
AUDIENCE: But in the sense that what really pushes.
PROFESSOR: Well, I mean I think you need both, the diffusive drift and the migration. But I think that the fact that the migration is from the mainland, and it's biased towards those abundant things, I think is necessary or important.
AUDIENCE: I guess just in terms of distinguishing between the niche and the neutral models, as applied to the mainland, does the niche model predict also a log normal? Because it seemed like, in the discussion earlier, the neutral also predicted log normal [INAUDIBLE].
PROFESSOR: That's a good question. In this whole area, I mean it's a little bit empirical. The fact that the niche model kind of predicts this, or this broken stick thing predicts a log normal, they didn't say anything about islands there, right?
I guess even Fisher's original log series, he used it to describe-- I think maybe that was the beetles on the Thames. But his original data set, where the Fisher log series was supposed to described it, as it was sampled better and better, it eventually started looking more and more like a log normal anyways.
I mean it's easy to see the frequent species, because you see them. This tail can actually be very hard to see, because you have to find the individuals. It's a good question of to what degree each of the models really predicts one thing on one place and another.
There's always tweaks of each model that adjust things. So I think it's a bit muddy. But the one thing that I want to highlight. So there's a lot of debates, then, between these different models. And each of the models have some fit.
They have red and black. There's one that kind of goes like this. And another one that kind of goes like that. And they're not labeled, because they look the same. And you can argue about chi squareds and everything, but I think it's irrelevant. They both fit the data fine.
And the other thing, just the sampling of kind root n sampling, if you expect to see 10 species, then if you go and you actually do sampling, you expect to have kind of a root n on each one. I mean the error bars, I think, around this are consistent with both models.
So I'd say that the exercise of trying to distinguish those models based on fit to such a data set I think is hopeless from the beginning. And then you can talk about the number of parameters. And if you read these two papers, they both say that they have fewer number of free parameters.
And it is hard to believe that there could be a disagreement about this. But then, you know, it's like, oh, well, what do you call a free parameter?
And then what they say, any given RSA data set contains information about the local community size j. So they say, given that, it's not a free parameter, because you put that in. That's the number of individuals. And then outcome is your distribution, right?
And you say, OK, well, all right, that's fine if you don't want to call that a free parameter. But then when you fit the log normal to this distribution, the overall amplitude is also to give you the number of individuals in the metacommunity.
So if you don't call j a free parameter in this model, then you can't call the amplitude a free parameter when you fit the log normal, at least in my opinion. I think that they both have three.
Because if you fit a log normal to this, you have the overall amplitude. That's the number of individuals. And then you have the mean and the standard deviation or whatever. From that standpoint, I think they're the same. Yeah.
AUDIENCE: But I mean how do you fit the log normal when you don't impose? Do they impose the amplitude? I mean it's still a parameter.
PROFESSOR: No, that's what I was saying. It's a parameter. I mean the normalized log normal, you integrate, and it goes to 1. But then you have some measured number of individuals in your sample, and then you have to multiply by that to give you.
AUDIENCE: But is that what they do when they do their fit?
PROFESSOR: Yeah.
AUDIENCE: Or do they keep that amplitude as also a parameter?
PROFESSOR: I think that you can argue whether this is a free parameter or not. But I think that you can just put it as the number of individuals, and it's not going to affect anything. You could actually have it be a free. But this gets into this question about what constitutes a free parameter or not.
And actually, there is some subtlety to this. But I think, at the end of the day, the log normal is not going to look like this. You have to. You basically put in the number of individuals that you measured.
AUDIENCE: So when you calculate [INAUDIBLE]?
PROFESSOR: Huge numbers of pages of has been written about comparing these things. At some point, it comes down to this philosophical question about what you think constitutes a null model. And this gets to be much more subtle.
And I think reasonable people can disagree about whether the null model that you need to reject should be this neutral model or if it should be a niche-based model. Or maybe it's just that there's some multiplicative type process that's going on and gives you distributions that look like this, and you need other kinds of information to try to distinguish those things.
And in particular, I'd say that it's really the dynamic information in which these models have strikingly different predictions, and then you can reject neutral-type models. Because that neutral models predict that these species that are abundant are just transiently abundant, and they should go way.
Whereas the niche-based models would say, oh, they're really fixed. And indeed, in many cases, the abundant species kind of stick around longer than you would expect from a neutral model. Of course, the neutral model is not true in the sense that different individuals are different. But it's important to highlight that even such a minimal model can give you striking patterns that are similar to what you observe in nature.
And so I think we're out of time. So with that, I think we'll quit. But it's been a pleasure having you guys for this semester. And if you have any questions about any systems biology things in the future, please, email me. I'm happy to meet up. Good luck on the final.