Flash and JavaScript are required for this feature.
Download the video from iTunes U or the Internet Archive.
Description: In this lecture, Prof. Jeff Gore finishes the discussion of the Lotka-Volterra competition model. He then moves on to the topic of non-transitive interactions, what he calls rock-paper-scissor interactions.
Instructor: Prof. Jeff Gore
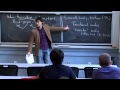
Dynamics of Populations in ...
The following content is provided under a Creative Commons license. Your support will help MIT OpenCourseWare continue to offer high quality, educational resources for free. To make a donation or view additional materials from hundreds of MIT courses, visit MIT OpenCourseWare at ocw.mit.edu.
PROFESSOR: Today, what we want to do is finish off our discussion of Lotka-Volterra competition models. So starting with this idea of the two species interacting competitively but then moving on to try to think about the general properties of Lotka-Volterra systems. In particular, when you have more species, the kinds of dynamics that you can get.
Also, we're going to talk about these non-transitive interactions, which are the rock-paper-scissors type interactions that may facilitate the maintenance of diversity in populations or ecosystems, in particular, in the presence of some sort of spatial structure.
And so we'll talk both about this demonstration of rock-paper-scissors type interactions in the context of male mating strategies in lizards, this paper that was by Curt Lively. And then we'll talk about another paper in the microbial realm, where they showed that there are rock-paper-scissors type interactions in the context of colicin production, toxin production in the context of bacteria.
Then, at the end, we'll talk about these population waves. There was a rather mathematical reading that I had originally proposed, but then we kind of switched things up a bit, so that you could, instead, read that rock-paper-scissors paper-- that is confusing-- that I think is maybe more fun.
But I'll tell you kind of this basic idea of the population waves, what happens, if you have a combination of some growth process together with some effective diffusion, you can get these population waves that correspond to this process of range expansion, where a population expands into new territory.
I just started by putting up what we had from the last class. So this is the two species Lotka-Volterra competition model. So what we found was that, for this to be competition, for the species to be kind of bad for each other, that that corresponds to the betas being positive.
Now, what we found is there are four cases in terms of the outcome of this two species interaction. And we wanted to, at least, try to get some sense of why that was and what the trajectories might look like. If you look at and N1 and N2, we can draw these nullclines and then get a sense of where the trajectories are going to go.
Now, the basic outcome of this two species Lotka-Volterra competition model are really exactly the same as the possible outcomes when we're thinking about frequency dependent selection, where we could get, in this case, that species 1 dominates or species 2 dominates, 1 or 2. But then we could also get coexistence or bi-stability.
And indeed, there is, in general, a mapping from the Lotka-Volterra kind of approach here and the approach that was kind of in Martin Nonwak's book of thinking about frequency dependent selection in this population. And so then it's not a surprise that you get the same four outcomes between these two situations.
And I think that this is also highlighting some very interesting and deep connections between evolution, which is changes in, say, allele frequency in a population of a single species, over time, and then some of these ecological kind of processes, where you're really thinking about these as different species.
Now, of course, in the case of the evolutionary dynamics that we analyze Martin's book, though, those were the evolution of different clonal populations in asexually reproducing populations. Do you guys remember what I'm talking about? All right.
So I just want to draw a couple of these sorts of diagrams. I'm not going to draw out all four of them, because it does take some time. But hopefully, we can reconstruct where we were.
The case that we were analyzing before, the N1 dot equals 0. We're going to have it as a dashed line. And N2 is going to be-- well, maybe we'll try to use thick chalk. Oh, wow, I missed. These are thick lines. So we'll draw these nullclines over here.
So the N1 dot-- and indeed, one of the comments is that it would be nice to draw where the nullclines are on the axes as well. And indeed, we can do that. We aim to please. So the dashed lines correspond to N1 dot being to 0.
So here's N1, N2. So N1 equal to 0 corresponds to this thing. And then we have this other guy that's a line, here. Now what we found is that, if N2 is equal to 0, this intersects at K1, whereas the intersection over here is at K1 divided by beta 12.
Now, we have our other nullclines that correspond to N2 dot equal to 0. Now one of those lines is indeed going to be along here. And the other line can fall-- there are four different possibilities for how we might draw it in relation to this N1 dot equal to 0 nullcline.
And depending on the orientation of that, we'll end up getting these four different possible outcomes of 1 dominating, irrespective of the initial conditions, 2 dominating, irrespective of initial conditions, or coexistence or bi-stability. So bi-stability is the only-- so if you start with a finite number of each of these two species, then bi-stability is the only case where the outcome depends on the starting condition.
So, of course, if you start out without one of the two species, then you won't get creation of those species, right? Because the only way to get creation of species 1 is to have some species 1 individual in this model.
So we're going to get another line, here, corresponding to N2 dot equal to 0. And I think that the one that we were trying to analyze was with the solid line underneath. Is that consistent with people's notes?
So we can draw some other line here. It doesn't have to the same slope. Now it's good to be clear about where these things are going to fall. So K2 is this point. And now K2 divided by beta 21 is over here.
Now recall that beta 12 is telling us about how much species 2 is reducing the growth of species 1. Whereas beta 21 is how much species 1 is reducing the growth of species 2.
Now, everything comes down to the relative ordering of these two quantities and these two quantities. And since there are two possibilities on each, that gives us the four possible outcomes. And broadly, the idea here is just that, if the species are weakly interfering with each other, then what should happen?
Yes, then you should get coexistence. Coexistence is when the betas are small. Of course, this is a concrete model, so you have to define what you mean by small. And indeed, small here ends up being relative to the ratios of these carrying capacities.
If the carrying capacities are just equal to, say, 1, then that's saying that the betas-- sorry, if the carrying capacities are the same, then the simple way to think about this is just whether the betas are larger or smaller than 1, whether each species interferes with the other species more than a member of that other species. So if carrying capacities are the same, that's what demarcates the different zones.
So what we want to do is take this sort of diagram and try to figure out where will the trajectories be on this diagram? Now, it's always good to locate the fixed points.
The fixed points of the system are? And somebody, words? How would we define fixed points in this system?
AUDIENCE: [INAUDIBLE].
PROFESSOR: What's that? Both lines intersect, right? So when the dashed line intersects the solid lines, right? So we have one such fixed point here. We have another fixed point here and another fixed point here.
Have we figured out the stability of those fixed points? No. What's the stability of this fixed point? It's unstable, right? Because we've already, previously assumed that these r's are greater than 0.
We're assuming that the species would be able to survive on their own. And that's actually true for both species. So this thing is unstable kind of in both directions, right? And what are the eigenvectors associated with this fixed point?
On the count of three, draw, use your arms like the hands of a clock to indicate the directions of the eigenvectors. All right, ready, three, two, one. All right, all right. There's no diagonals, right?
Of course, you could also. I was waiting for somebody to be kind of obnoxious and point in the other direction. A surprisingly not obnoxious class we have. So this is just saying that, if you start out just a little bit of one species, you'll just stay with that species. It makes sense.
Now, what do these lines tell us about the directions of the trajectories or the orientations of the trajectories? Why did I draw them? Yes?
AUDIENCE: These trajectories are-- these are lines are nonlines. And one of the derivatives [INAUDIBLE].
PROFESSOR: One of the derivatives, all right. And in particular, let's look at this line here. Are the trajectories? Again, we're going to do our arms to indicate the orientation of the trajectories. In particular, there's a trajectory right here. What direction will that trajectory be pointing? There's only going to be one arm. Ready, three, two, one.
All right, we got a lot of people not voting. All right, that means we need to turn our neighbors and discuss. If you didn't vote, it means, I think, not following what we're talking about. Turn to somebody. If your neighbor agrees with you about which direction you should be pointing your arm, then try to figure out whether we know which orientation the arrow should actually be in.
[SIDE DISCUSSIONS]
PROFESSOR: We'll figure this out.
[SIDE DISCUSSIONS]
PROFESSOR: Let's go ahead and reconvene, because it seems like some people are being quiet. But I'm not sure if that's because they think they know what's going on or they're just very much unhappy with the situation. Let me see a fresh voting. All right, ready, three, two, one.
Definitely, it's going up or down, right? Because the definition of this dashed line is that N1 dot is equal to 0. We don't know what N2 dot is. We'll figure that out in a moment. But what we know is that the trajectories we should be something, lines here, either up or down. And we're going to find that they're down but here.
Now, on this solid line, quickly, the orientation of trajectories. Ready, three, two, one. All right, perfect. So we know that N2 dot is 0 here.
Now, an actual direction of the trajectory, at this point, right here. Ready, three, two, one. OK, good. Because in the absence of N2, we know that species N1 should just come to carrying capacity K1.
Same thing over here, we should get arrows coming down. So indeed, what you can see is that the arrows are coming down here. That means they actually do have to come down immediately to the right of them.
Whereas over here, the trajectory is point right here, so we can kind of figure out that-- so they're coming here. And from far away, they're coming here. And you can see that they have to come across here. And then they're going to come into this point.
So this is going to be our stable fixed point. I'll color it in to indicate that. From here, they're going to curve around though. So if you start out right here, you kind of do this business. From here, we come in.
Are these lines allowed to cross each other? No. Now, indeed, you could actually see here, what is the direction of the other eigenvector at this point? Using a hand, arm, ready, three, two, one. Right, it's kind of something in there.
Because there's all these trajectories are coming here, and then they approach this fixed point from this point. Because here, the other eigenvector is still horizontal, right? Because we know, if we don't have N2, then we just have N1.
But this other eigenvector is not purely straight up. The other one is along here, because the trajectories are coming in along that. Does that make sense? So in this case, we should just be clear.
We are in a situation where K1 over beta 12 is greater than K2. Another way of writing that is that beta 12 is less than K1 over K2. So that means species 2 does not strongly harm species 1.
Yet, we know that K1 is greater than K2 divided by beta 21. So that means that beta 21 is greater than K2 over K1. This is telling us that species 1 is strongly harming species 2. And that makes sense.
In that case, species 1 wins. Does that outcome change if we change the r values, the division rates? A is yes. B is no. I'm going to give you 10 seconds. What I just said, if I change r's does that change the outcome? Ready, three, two, one.
So we've got a majority of B. So the answer is no. This statement that, in this situation, species 1 dominates, that's independent of the r's. And what you see is the conditions, here, only depend on the what's in here.
So the actual shape of those trajectories will depend upon the r's. So if it's the case that species 2 is just a faster grower than species 1, then you might end up with a situation where, if you start with a little bit of each, you might come way up here-- well, no. I guess you might come close to this fixed point. So you might think that it really looks like species 2 is about to win. But eventually, they'll curve over and come back.
And indeed, in the Strogatz book, one of the chapters that I recommended, he has an example of sheeps and rabbits, the idea is that they are competing species, maybe eating similar foods. I don't know if that's true. But the rabbits can divide more rapidly.
So here, the idea would be, well, if the sheep can really displace the rabbits, because it's just bigger and push them aside, then what can happen is that the rabbits first divide rapidly. It looks like they're going to take over, but, over time, eventually, the sheep population kind of grow up, and they start displacing rabbits. And you end up excluding the rabbits.
This is this phenomenon of competitive exclusion. And depending upon the context-- OK, that's an e-- this is either more or less maybe formally phrased. But the idea is that, if there are two species that are too similar, and in particular, if they're somehow perfect competitors, they're really just trying to eat the same thing, then you should only end up with one of the two species surviving.
And that's the kind of idea here. Although, I think, you can argue about the mapping I think. So this is one of the four outcomes. And of course, it takes 15 minutes to go through each of these examples. So we're not going to go through all of them.
But you should be able to, for a given a combination of betas and K's, be able to figure out, using some combination of algebra, derivatives, fixed point stability analyses, and drawing of things, well, you should be able to do all of the above.
Are there any questions about where we are here?
AUDIENCE: I guess that none of this holds in the stochastic [INAUDIBLE].
PROFESSOR: Yeah, that's an interesting question.
AUDIENCE: For example, in that case, if r2 is much bigger, then you're going get almost, very close to like K2, and then maybe just the last individual [INAUDIBLE] and then you just add that fixed point.
PROFESSOR: Right. So it's certainly the case that, once you have stochastic extinction-- the thing is that, you would probably be most susceptible to stochastic extinction. In the case you were talking about, you would be most susceptible to stochastic extinction when you're around here, actually. These trajectories are still always moving up in N1 space.
I think I know what you're saying. We're going to maybe zoom in onto this N1, N2. Because we have this unstable fixed point here. And the claim was that, if you start out over here, then the trajectory might look something like this.
And you'd say, oh, well, you might go extinct here.
AUDIENCE: The idea was just the statement that [INAUDIBLE] r1, r2 are very dynamical [INAUDIBLE].
PROFESSOR: It's true that, I guess, things change. There are a number of things you might want to say. First of all, this is a purely continuous and deterministic description of the setting. It allows for fractional individuals. There's no shocks or perturbations that you have to worry about.
I guess the only thing I wanted to say, in regards to your question, is that I think the stochastic extinction will not be dominated. We're talking about stochastic extinction of species 1. It will not be dominated due to a stochastic extinction here but stochastic extinction at the beginning.
I haven't drawn this very, very well, but, in this case, I think these trajectories are always are going up in numbers of the 1 species, which means that you're most likely to experience stochastic extinction at the beginning. Yeah?
AUDIENCE: r1 and r2 should still really mess with stochastic extinction a lot, right? Because the larger r1 and r2 are the quicker we get out of the regime of stochastic extinction.
PROFESSOR: That's all true. We have to be careful about many of these things. In particular, if you go and you do a stochastic simulation of this, so let's say you plug this thing into a Gillespie simulation, can you get stochastic extinction? A yes, or B no, ready, three, two, one.
No. So the answer is no but why?
AUDIENCE: It depends. If you take r combination [INAUDIBLE].
PROFESSOR: Exactly. So right now, as written, there's no death. Although, I guess you could say that this a death. There's a question of how you partition things. So in principle, this is the difference between the growth and the death rate.
But the most straightforward way of doing such a simulation is that you put this whole thing in here as a rate for birth. Somebody is going to say, ah, that's not how I was going to do the simulation, right? Well, that's probably how you were going to do it.
AUDIENCE: That's a terrible way.
PROFESSOR: But if you did that, if there's only birth, then you can't get stochastic extinction, obviously. But in general-- and this is one of things we spend our time a lot time thinking about in this semester-- there are multiple ways of doing kind of a stochastic simulation from a deterministic equation.
And this thing, you could be more explicit and say, oh, this thing is really a B2 minus a D2, so a birth rate minus a death rate for example. And form the standpoint of a differential equation, it doesn't make any difference.
But if you do the Fokker-Planck approximation or you do a simulation or whatnot, then these lead to different things. In particular, the rate of, say, stochastic extinction here increases as B and D increase, because that leads to more of these fluctuations.
So there are many things that are different once you include the stochastic dynamics. But it's always good to get a base sense of the dynamics from the standpoint of just deterministic differential equations before you think too much about the stochastic dynamics, because otherwise you get overwhelmed quickly.
Any other questions about that?
What I want to do is just spend a little bit of time to think about the more generalized case of more species. And in particular, we could convert this set of equations. We can normalize by each of their carrying capacities. And we can convert a set of equations to look something like this.
So now we just have Xi dot is equal to-- there's some ri Xi, 1 minus. And what we can actually do is normalize everything so that it's just written like this. And normally, what we assume is that we've done things such that alpha i i is equal to 1 for all i.
So this is just saying that this is the normalization such that each species inhibits itself in a way that it's just give a simple logistic growth. And it's going to be logistic growth with a carrying capacity equal to 1.
And then once you've done that, then a species inhibits itself with alpha i i 1. And then everything comes down to what this alpha matrix is. And I would say that, as always, it's really very, very important that you can go back and forth between the non-dimensionalized versions of equations and the base version.
This was something that, on exam number two, there were quite a lot of problems where we asked about, how does the parameter change when you change the strength of expression or this or that, right? So this is something that I think is very important. Because this comes up a lot.
So in this case, the alpha matrix tells you kind of everything. And then there are a number of things that, well, the mathematicians have proven about these sorts of equations. And I just want to point you towards some of those things to think about.
So first, I'm considering a case where, again, alpha i j is greater than 0, again, for all i and ji, or greater than or equal to 0. So some of them can be 0. But the interactions, when they exist, are competitive.
So first, if you start out in the region where all of the species start, between 0 and 1, then you stay in that region. If this is true initially, then it will be true forever. That's good. Negative abundances, you maybe were not so worried about.
But it's not as obvious that you can't get above one, because there's nothing saying that, in principle, you couldn't have started there, right? Or in principle, you couldn't have gotten there?
And certainly, it's physical to think about starting outside of that region, because we often talk about carrying capacities as something that's like. If you think about, this is an Xi as a function of time.
So in these situations, it's not crazy to think about something above the carrying capacity. But this mathematical statement about the Latka-Volterra framework is that, if you start out with everything below its carrying capacity, then everything will always stay there.
And all the dynamics occur-- this is for i equal to 1 to N Do I want to use a big N or little n? Does it matter? So this is big N, different species. The dynamics occur on an N minus one manifold. If we have many mathematicians, they can explain what this technically means.
But basically, what it's saying is that there's going to be some N minus 1 dimensional surface or volume or whatnot where all the dynamics are going end up being on. And what that means is that, in particular, a limit cycle requires two dimensions, requires 2D, which means that to get a limit cycle requires-- so then N has to be greater than or equal to 3 to get a limit cycle.
Can you see what I'm saying? Whereas chaos requires 3D, and that means that N has to be greater than or equal to 4. And indeed, you can get limit cycles with N equal to 3. And you get chaos with N equal to 4.
There's another theorem that says that any dynamics are possible for N equal to 5 or larger. And chaos seems as much as I would want to ask for. But there's, apparently, a 4D torus, something that is different. This is something to think about in your spare time. Well, you know, like a lot of things.
And this is worth spending a moment talking about. First of all, so here's a two dimensional thing. The special thing, as you might remember, about continuous dynamics is that these trajectories are not allowed to cross each other, right?
Which means that you just can't draw a chaotic trajectory, because you're going to have to cross yourself again. And that's also why a limit cycle requires two, because we originally tried to draw a limit cycle in one dimension, and it didn't work. Do you remember this?
And the same thing with-- you can imagine, here's a nice limit cycle. And that could be stable. Oh, and incidentally, you were right about. I was getting confused about the Poincare-Bendixson theorem. Because I think there are these funny things. It wasn't. OK, well, you know, all these people that complain about what I say.
Because if you have the trajectories coming in, then what I said was that, if you had an unstable fixed point coming out, then you could draw this region, then you could be guaranteed that there was a limit cycle in there. But you cannot, just based on what I had said, say that, if it's a stable fixed point, then you won't get a limit cycle.
I mean in some other cases you can prove that it doesn't work. Particularly, like in the Latka-Volterra, there in one of the crossings, it's now going to be a stable fixed point. In that situation, you can prove, mathematically, that you can never get a limit cycle oscillation because of some divergence condition of some function and so forth.
But in the example that I was telling you about, in the predator-prey, it was true in that particular case that, when that thing is stable, you don't get oscillations. And when it's unstable, you do. But both directions do not follow from the Poincare-Bendixson.
This is a limit cycle. Now, in a chaotic situation, you have to be able to do something where you kind of come around and then, every now and then, you kind go over here. And then you go around and around. Something crazy happens.
But you can see that this situation doesn't work if it's only 2D, right? Do you see why I'm saying that?
AUDIENCE: Yeah.
PROFESSOR: You don't? You don't agree. It's just, if it's 2D, then these lines cannot cross. So you need to a third dimension, so that they can just shoot above and below each other.
AUDIENCE: And what's with the definition? Because you can come up with trajectories, like you can have trajectories that asymptotically approach the limit cycle but that never cross themselves, right?
PROFESSOR: Yeah.
AUDIENCE: Those are obviously not chaotic, but the y--
PROFESSOR: Right. So this is not actually class in non-linear dynamics, so we're not. So normally, you characterize this Lyapunov exponent, which tells you about how the phase space is kind of growing or shrinking. In the case that you were just talking about, that's a case where all the trajectories come together.
Because in this case where this trajectory comes into the limit cycle, if you draw like a blob of phase space, it's going to come together over time. Whereas in a chaotic system, if you have a blob of phase space, it's going to diverge and fold and do all the craziness. Yes?
AUDIENCE: So you said [INAUDIBLE] essentially you need n greater than or equal to 3. Is that just for this--
PROFESSOR: Yeah. Yes. So this is in this Latka-Volterra. Because, in general, you can get a limit cycle with two equations. And that's with the 2D. And in general, you get a chaos with three equations or three variables. But in the Latka-Volterra model, it requires three and four, respectively.
That doesn't mean that every four species Latka-Volterra will have chaos. But it means that it's possible to get one. And I think one thing that's just rather striking is that this really is kind of the simplest, possible model you can ever write down describing how species might interact or variables might interact or whatnot.
And so it's really kind of incredible to me that you get all these crazy dynamics. Yes?
AUDIENCE: I was going to ask about the n minus 1 thing. This dynamic [INAUDIBLE] n minus 1 [INAUDIBLE].
PROFESSOR: Yeah.
AUDIENCE: Over here it just looks to me like-- we have n equals 2 and the dynamics are occuring on a plane, on a two-dimensional plane.
PROFESSOR: Yeah. The transience or whatever can occur. It requires the full N dimensions to describe. Because you can start-- to describe all of the trajectories, clearly requires all dimensions, right? Because anywhere you start, you have to have specify it by five dimensions.
But the dynamics, as far as like-- and here, there aren't even any dynamics. I think that you go to a point.
AUDIENCE: What do you mean by dynamics? [INAUDIBLE].
PROFESSOR: Yeah, I agree that there is a-- so in the case of the limit cycles and so forth, this is really like, at steady state, it's doing something. So here, steady state, it only goes to the fixed points. But if you have a three dimensional system, then you can have it steady state, the trajectories on like a plane. Yeah?
AUDIENCE: Is it because there's like some concept of [INAUDIBLE] that can be [INAUDIBLE] for the system?
PROFESSOR: I don't think that that-- I'm not aware of that being the case. But I'm hesitant to say that it's not true.
I want to switch gears a little bit and think about three species interactions and, in particular the three species interactions when they're non-transitive. Because this is thought to be potentially a significant stabilizer for diversity in populations.
So this is non-transitive interactions. And we often just say rock-paper-scissors. Is everybody from a cultural that plays rock-paper-scissors? Yes?
AUDIENCE: Yeah.
PROFESSOR: So this is a true, human universal. Although I think that what we call it does vary and so forth. You know how sometimes the linguists try to find this ur-language that our ancestors spoke 50,000 years ago or whatever? I think that you probably do something similar with rock-paper-scissors, because it seems to be a pretty common theme.
But the idea here is that you have-- wait, which direction does it go? So paper beats rock, scissors beats paper, but rock beats the scissors. So you can imagine that this kind of dynamic can be captured in the Latka-Volterra type framework.
So you just have to set up these betas or alphas so that this is true. And again, the way to think about this would be-- the simplest thing is to think about it as dominance. So if you have the rock species and the scissor species together, then the rock species will drive the scissor species extinct and so forth.
Now, this is the kind of situation that, in principle, can lead to very complicated dynamics in multi-species ecosystems that, at least in many models, can stabilize the coexistence of multiple species via some of these complex, crazy dynamics that we were just talking about.
I would say that, as a mechanism for the stabilization of diversity, I don't know how convincing that is in terms of being what explains why it is there's so much diversity outside, when you look outside the window. But, at least, it's in principle true.
And one of the topics of these papers-- certainly the one you just read-- is that rock-paper-scissors, i.e. non-transitive interactions on their own, may not be sufficient, but, in the presence of spatial structure, maybe it does allow for long-term coexistence of these species or strategies and so forth.
And again, it's not always clear in these situations whether you're thinking about ecology, where these are different species, or you're thinking about evolution, where these are different genotypes. And indeed, in the Kerr paper that you read, these are all E. coli. So it's all one species, it's just that they have different mutations. So that's really rock-paper-scissors in the context of evolution.
Whereas in the male mating strategies paper by Curt Lively, that's more of an ecological context, but it still is evolution within the species. Because these mating strategies are heritable.
I did tell you the base idea of this lizard mating strategy business? Or did I never say anything about that? Not really? OK. For some reason, I thought that I'd alluded to it. Well, let me explain it to you.
I think it's kind of an incredible paper. So this is a paper by my Curt Lively. So it's Sinervo and Lively. It was Nature in '96. And it's called "The Rock-Paper-Scissors Game and the Evolution of Alternative Male Strategies."
So what was known is that there are many examples of alternative mating strategies in males. In particular, it's rather common that there are, what you might call, territorial males and what they often call sneaker males.
This is observed in fish and various land animals. And in many of the cases, these sneaker males really do look phenotypically like females. And this has been measured using various kinds of observational, experimental approaches.
There's often what you call negative frequency dependent selection between these strategies. The sneakers can often, when rare, spread in population of the territorial and vice versa. But this was, at the least the first case I'm aware of where these ideas had been demonstrated, that there were really three strategies. And the three strategies implemented, one of these rock-paper-scissors interactions.
I want to maybe make a little more space. If you guys are available after class, I encourage you to come up and look at the paper, because they actually have pictures of them, so you can identify the sneaker males. Because they actually look different based on the coloring of their throat.
So these are lizards that live in the mountains up outside of the Bay Area in California, in Merced County. They're side-blotched lizards. I don't know anything about that.
And what they showed was that there's these guys with orange throats that are kind of aggressive. And they defend a very large territory with a large number of females. And they fight off any males that come.
Then there are the dark blue. Sorry, I should have put that over here. So there are other lizards here that are dark blue throats. And these guys are less aggressive with smaller territories.
So you can guess, if it were just the orange-throated guys and the dark-- and these are genetically encoded strategies, in the sense that they do seem to be passed on, and it's determined by the genes that the male inherits-- less aggressive and small territory.
Can you guess which one wins between these two, against each other? What's that?
AUDIENCE: The two aggressive ones
PROFESSOR: Yeah, but if you just have the two aggressive ones?
AUDIENCE: Yeah.
PROFESSOR: This guy's going to beat this one, right? That's just because this aggressive male has a larger territory, and they pass on more genes than the less aggressive ones.
However, what they found is that there's a third mating strategy here, which are these sneaker males. And they indeed look like the females. They have these yellow stripes on their throats.
They look like the female, and they have no territory. Sneaker males, so they don't defend any territory. Instead, they just sneak into the territory of the other males and try to mate with the females in that territory.
And what they show is that, over the course of seven years, in the mountains of Merced, they see that the frequency of these things goes through an entire oscillation. So they see just over one period of this oscillation.
And their argument is that, although it's true that the aggressive males can outcompete the less aggressive ones, the sneakers actually outcompete these aggressive ones, basically, because these aggressive males, it's just too large of a territory for them to effectively defend it.
So the sneakers can actually outcompete the aggressive males. Yet the less aggressive ones can outcompete the sneakers, because they are trying to defend a smaller territory. So they basically measured the frequency of these strategies and also the number of females in the different territories, from 1990 to '96 or so, and saw that this thing kind of went around in some circle in this frequency space of alternative male strategies.
Kind of an incredible paper. So the idea here is this is a situation where it is non-transitive. So there's this rock-paper-scissors type dynamic. And it's also spatial, because these lizards are in some particular place, they have some territory and so forth.
And in this other paper, by Benjamin Kerr, what he wanted to do was try to understand something about what the role of that spatial structure might be in maintaining the diversity. So in this case, the argument was that, if you go out and you look at these lizards, the reason that you see all three of these strategies is because of this rock-paper-scissors dynamic.
If one of the strategies becomes more rare, it's going to have an advantage relative to the others. It's going to spread. And what Benjamin Kerr wanted to explore is whether that statement could be made-- somehow you can distinguish between whether the spatial component is important or not.
Of course, it's hard to do that in the case of the lizards, but he was able to implement this in the case some chemical warfare behavior in bacteria. So in this paper by Kerr, and it's also a Nature paper from 2002.
So this paper is called "Local Dispersal of Promotes Biodiversity in a Real Life Game of Rock-Paper-Scissors." So you guys read it. So what were the three strategies?
AUDIENCE: [INAUDIBLE].
PROFESSOR: So C is the colicin producers. Incidentally, just this colicin production is kind of an incredible phenomenon already. So these are proteins that are produced that bind to other bacteria and can often make pores in the membrane and kill them.
But the amazing thing about the colicin production is that in E. coli and other gram negative bacteria, the only way that these colicins are released is by cell lysis. So it's not just that the cell is engaging in some costly behavior in order to make this protein that will kill other cells.
But the only way that the toxin is released is by the cell actually bursting open. So it's clear then that this has to be supported by some kind of group level or kin-selection kind of argument. This can never be good for the individual, because the individual has had to spill its guts in order to harm other cells.
So the only way that this can be supported is by inhibiting the growth of competitors and allowing your kind of kin mates or other cells that also have this plasmid, and, therefore, also the immunity protein, allowing them to grow better. So this is a very neat example of an altruistic, kind of warlike behavior.
So this is one of the strategies. What was the other two.
AUDIENCE: [INAUDIBLE].
PROFESSOR: Resistant. So there's R, which is resistant. And between the C and R, who wins?
AUDIENCE: R.
PROFESSOR: R, that's right. There might be some costs associated with being resistant, but the cost is not as large as actually bursting open. What's the last one?
AUDIENCE: [INAUDIBLE] sensitive.
PROFESSOR: Sensitive, perfect. So this is just the normal bacteria. And the argument is that there's often a cost to be resistant, which means that sensitive bacteria will outcompete resistant. Yet, if it's just the sensitive and the colicinogenic strains, then this strain can beat this strain.
So this is the idea of the rock-paper-scissors game in this system. They do say that, in some situations, the fitnesses are such that it's like this. And it's good to take these sentences seriously, because, if they thought that every time that you isolate a resistant bacterium, that it would satisfy this, they would have said, when you do this, this is what you see.
Their phrasing tells you that actually, depending upon which strain you get here or there, you may or may not see this. So you have to be careful. Just because there's a nice paper that's written about this doesn't mean that, if you go out and you find particular strains that have these properties, that it will always yield this particular outcome.
So they argue that these strains, just because they have a non-transitive interaction, does not necessarily mean that they will be able to coexist in a well mixed environment in particular.
And in their simulations and the experiments, where they did experiments in a test tube, which strain died first? The sensitive strain. Does that make sense?
Yeah, right? And the other thing to remember is that these strains, there's no reason that they should be accurately described by a Latka-Volterra type formulation. And in particular, it could just be the case that, if you have enough producers and they make enough of the colicin, then the sensitive cells are just all dead.
And that will, in general, be hard to capture in this sort of framework. So the idea is that if you start with a bunch of N's, N for each of these three, then first you see that the sensitive cells die. And once the sensitive cells have died, then you're really just playing an interaction between these two. In that case, you get the colcinogenic strain dying, and you're left with just the resistant strain.
One thing I want to caution you about, though, is that, just because in this experiment they saw that coexistence of three rock-paper-scissors type strains was not possible in a well mixed environment, it does not mean that it will always be the case.
This is a very well known paper in the field. And the thing is that it's easy to forget what a paper shows and what it doesn't show. So what this shows is that there is maybe a set of these three strains that have a rock-paper-scissors type interaction.
And in those particular three strains, that are interacting in this particular way, via colicin killing da-duh, then they don't coexist in this particular well mixed and maybe other well mixed environments as well. But this does not necessarily show that any rock-paper-scissors interaction in a well mixed environment will not support coexistence.
And in particular, you might remember, in Martin Nowak's book, there are very reasonable equations that display rock-paper-scissors type interactions that can lead to coexistence. So you can kind of spiral in that space to a state of coexistence. So it's possible. But in this situation, it doesn't happen.
Can somebody remind us how they implemented the spatially structured environment?
AUDIENCE: [INAUDIBLE].
PROFESSOR: Yeah. So they used agar plates. They did this thing where they took this plate, and they kind of used a hexagonal grid of some sort. Is it actually hexagonal? Yeah. I don't know how they decided on the original order, but they said, OK, here is a sensitive, sensitive, resistant, colicinogenic, resistant. I don't know, whatever.
So they filled it up. They put maybe 20 different kind of patches there. And then they basically, each day, they just used one of these velvets, that we often use to do replica plating. And then they just kind of took off some of the cells and put it on a fresh plate. And then they did this for a week or so.
And then they saw that there was some sense that there was a spatial dynamic taking place, where the spatial structure was kind of reminiscent of this. The sensitive kind of moved into the resistant. The resistant kind of moved into the colicinogenic. Colicinogenic kind of moved into the sensitive.
But they couldn't actually see all of those, because two of the strains, they said, grew to similar density. That was between the sensitive and the resistant, right? So visually, they can only distinguish the colicinogenic strain relative to the others.
But what they found, though, is that, over a similar amount of time, they got coexistence of all three. So R, C, S, kind of all-- the number is just a function of time-- stuck around in this spatially structured environment.
So their argument is that, in some cases maybe, this non-transitive interaction is not sufficient to maintain diversity in a population, whether it's genetic diversity or species diversity. But it may be that it's very important to have this spatial component.
If you're curious about these things, there's also a nice computational study done by Erwin Frey, who studied these rock-paper-scissors dynamics as a function of the mobility of the individual agents.
And in that study, he found that there was sort of a critical level of mobility in which you kind of switch from a spatially structured environment with coexistence to, above some mobility, you end up losing the diversity, because it kind of goes into this well mixed regime.
So if you're curious about these things, you can look up Erwin's paper. Are there any questions about what they did here, what you think it means? Yeah?
AUDIENCE: You said you couldn't use [INAUDIBLE]. How did they know if it was [INAUDIBLE]?
PROFESSOR: They can just scrape off everything and plate.
AUDIENCE: And then you can tell?
PROFESSOR: Right. Because, well, then you can ask, oh, we're those guys sensitive to colicin or not?
AUDIENCE: Why not actually just inject them with colicin?
PROFESSOR: Yeah. Or plate them on something with colicin. This is a system that has been very influential, but it's really not yet or has not been a domesticated kind of model system in the sense that they are not nice, fluorescent proteins. This is not on a nice cloning plasmid. It was a natural plasmid.
And this resistant strain, the way that they get it is, basically, they take some sensitive cells, and they add colicin. Let's say they supernatant from here, and they ask, which cells grow> and then that's a resistant cell. And it's genetically resistant. But then each one's going to different.
They have done sequencing. It's a surface receptor that the colicin maybe uses to go in or other things. But indeed, we actually did some experiments with some of these strains. And it's a little bit messy. And I think that there's a sense that the field maybe needs to just make some nice plasmids, with nice colors, so that we can really distinguish things.
Because I think that this system, despite 500 citations or so, almost none of those citations are experimental papers really exploring this thing. Because I think that it still is just a little bit messy. And I think that it's also a very pretty system to explore these ideas. Yeah?
AUDIENCE: [INAUDIBLE]?
PROFESSOR: So the question is, why can't you distinguish these? And they say it's because they're similar densities. I don't even know if that's even. Of course, well, if you added sensitive cells here and sensitive cells here and they grew up, it's likely you're not going to see a boundary.
And just more generally, if you take similar strains-- and these are rather similar strains, this just has some mutation relative to this-- then you won't, often, see a boundary when the colonies kind of grow up.
AUDIENCE: So they just didn't see boundaries. It's not like they didn't see--
PROFESSOR: So they saw there were cells there, but they couldn't necessarily say that it was true that the sensitive cells were kind of spreading into the resistant cell region, because they couldn't see the boundary.
So in the last 20 minutes here, 15, 20, I just want to say a few things about these population waves. For many, many purposes, there are strong arguments to be made that, in the context of evolution or ecology, spatial dynamics matter.
And the way that we often think about the spatial dynamics is via some effective diffusive process. And that's convenient, because we know a lot about how to model it. But it's also maybe a reasonable description of the motion of animals and other living things over length scales that are large compared to the movements of the animals.
So what we do is we take some equation, such as, well, we often have, say, dN/dt. So this is going to be about population waves. So we take our standard thing where we say, oh, here's r N 1 minus N/K.
And then we just want to add some spatial dynamic. So what we're going to do is we're going to say, now, the derivative, now it's a density. So we're going to use a little n, just for fun. And we might still use a K just for simple. But then we have to add some diffusive term.
So there still is going to be a local carrying capacity. Now this is in terms of density of the organism. And we're going to assume that there is some diffusive type motion of the organism. Now this is this is, of course, going over the life-scale over which the animals are actually doing things over shorter time scales.
So it could be that different organisms have very different modes of motility. In some cases they walk or swim, in some cases they just get picked up by a passing deer. So there are a wide range of ways in which organisms move around.
But if you look at it over kind of longer length time scales, then maybe it doesn't matter. And certainly, if it's an unbiased kind of motion, with motility being well-behaved, i.e., if the probability distribution of these steps, as long as it's not long-tailed.
Similarly, Oskar Hallatschek, over at Berkeley, has been doing a lot of fun work looking at epidemic spread in cases where this kernel, the kind of step size distribution, has long tails. It leads to qualitatively different behaviors. So if you're curious about such things, check out Oskar's work.
But for normal kind of the step size distributions, then the central limit theorem type considerations just tell you that you can maybe just look at it like this over time spatial scales that are bigger. Yeah?
AUDIENCE: But is it a unique correlation in your step? Because the central limit theorem, as long as the variance-- I guess, you're saying?
PROFESSOR: That's why I'm saying long-tailed.
AUDIENCE: If the variance is infinite?
PROFESSOR: Exactly Yeah, so that's what I was saying. And indeed, people argue, in the case of disease spread, with modern air travel and so forth, that the probability distribution of kind of step sizes for infected individuals, over the next week, is long-tailed, right?
Because there's a fair chance that you're just going to stay around your local neighborhood, but there's a smaller chance you're going to go to the other side of town. But you might go to a business trip over in DC. At some small right, though, you also fly to South Africa and go to a conference.
So all of these things have reasonable probabilities, and so there are arguments that this is kind of some power law type distribution. And in those cases, you don't necessarily have finite variance, and so then you can't just put everything under the rug.
But we first want to understand what happens here, and then we can-- well, we're not going to-- but then other people can think more deeply about what happens in fancier situations.
It is worth saying, though, that this approach, I mean it looks very physics-y, in the sense that physicists like simple equations where we add diffusion and so forth. But this is not what a physicist came up with.
These are classic ideas in evolution and ecology. The solution to this was originally done by Fisher. So this was in the 1920s or so. So a long time ago, originally to try to understand not the spread of a population but the spread of a beneficial allele in a spatial population.
And once again, this highlights the deep connections between evolution and ecology. You can have a genetic wave in space of a of a beneficial mutant spreading, or you can have a population wave of an invasive species or whatnot, and you end up getting very similar dynamics.
So the basic idea, here, is that, if you look at the density as a function of position. If start with, there's one individual. What's going to happen? It's going to start dividing, right? So we kind of get up. And it's going to come up. And eventually it's going to saturate at this carrying capacity, K.
And then you end up getting these spreading population waves that look like this. And the reason we're calling it a wave is because the shape of this front is the same over time. So it can really to be described as some function x minus vt.
And we are going to, maybe, typically assume that we're in a situation where we don't have to think about the left and the right, because it's just too complicated. So we'll just imagine it being that it's at saturation here, and then we're looking at some front that's moving to the right.
Now, by dimensional analysis, we should be able to figure out what the velocity is going to be. Remember how much we liked dimensional analysis in this class? Yes. So what we're going to do is I'll give you some characters that you're going to be able to use in your quest.
So we can use r, K, D. I'll give you a square root in case you find it useful. And you can raise something to the second power as well. So what you can do is you're going to set up your cards so that when I look at it, from the left to the right, it will describe the velocity of this wave.
I'll give you 30 seconds. So this is dimensional analysis for this wave velocity.
All right, do you need more time? Yes? OK, that's fine.
All right, let's go ahead and vote. Construct your answer, remember, from me, from left to right. Ready, three, two, one. All right. We have trouble. A key skill is being able to imagine yourself in someone else's shoes. So if I'm viewing-- but that's OK.
So we have, here, this is kind of units of 1 over time. This is what length squared over time. Whereas this is a density. If we want something that is a length over time, then we're going to end up having to take r times D and take a square root.
So this should be DAC, which is a square root of-- of course, it depends on how you're entering it into your calculator, if you have scientific calculator or something else, maybe. And indeed, it ends up, there's a 2 here. So this is the velocity. Yes?
AUDIENCE: [INAUDIBLE].
PROFESSOR: I see what you're saying. But it ends up not being true. These derivative signs don't have any units. So this still has units of a density divided by a length squared. So for unit purposes, you just look at this thing and at this. This squared does mean there's a length squared in the denominator, but this squared doesn't do anything. So D is still a length squared over time.
So this is the famous Fisher velocity. There are some mathematical subtleties to all of this that we're not really going to get into. I don't know. I'm having trouble-- velocity. There are a few features to highlight.
If the organism grows faster, the wave is going to spread faster. That make sense? If it has a larger mobility, it also moves faster. That makes sense. Of course, the velocity is given by both of those things. So this wave coming out really is a population level property.
Because it's not just growth. It's not just motion. It's a result of the coupled division and diffusion that leads to this population wave spreading. Importantly, to first order, it doesn't depend on the carrying capacity, at least within the deterministic regime.
AUDIENCE: It's interesting that, if the reproductive rate goes to 0, suddenly the population stops spreading.
PROFESSOR: So first of all. You'd say, oh, it would be sort of surprising if it really kept on spreading in the absence of growth. But what you're pointing out is that, if you just, at one moment, turn off division, then there will still be diffusion. It'll still keep on going.
But this is the velocity of a wave when it's a wave. When it's described by a function like this. So it's true that you could turn off division, and it'll still diffuse. But then the shape is changing as well.
I'm going to draw a few lines describing possible populations. Now, let's assume that they have the same motion, diffusion. I want to know, which one has the largest velocity? Is it A, B, C, D? It's the same diffusion. Per capita growth rate as a function of the density for three different organisms.
Ready, three, two, one. All right, so I'd say we have a fair number of B's, D's. It seems like it's B versus D. Now, this is tricky because D, we have not explicitly considered here.
But it turns out that the answer is B. Certainly, between these three, these are all really logistic growth functions. And so from the standpoint of here, it's just this r. And r is the division rate at 0 cell density. The per capita growth rate at 0 cell density is what determines the velocity in a Fisher wave.
And indeed, that's true even if there's no decrease in the growth up right until you get to some carrying capacity. And indeed, all of these cases, the division rate and the growth rate that's relevant for the velocity is when it hits this axis.
And indeed, all of these waves are described as Fisher or pulled waves. Because there's a sense that the entire wave is determined by the front of the wave. So we drew this profile. I didn't do that very well.
Here, this is an exponential. And the exponential actually is what's pulling this wave. There ends up being a characteristic length scale here that is the square root of D/r. So this is the length scale of the exponential.
And the velocity and the length scale are only functions of the division rate in the limit below cell density or low density of organism. The shape of what goes on here changes, indeed, the bulk properties of the wave, but doesn't change the velocity.
And I just want to make one comparison of all this to-- because there's another qualitatively different kind of wave, which is a so-called pushed wave. And that's what happens if you have an Allee effect, particularly like a strong Allee effect. If this thing looks-- like this is certainly possible. This is an Allee effect.
Now, if you just said, oh, the only thing that matters is the growth rate at low cell density, you would say, oh, this thing cannot possibly expand. Although it turns out that it still is possible. And in this situation, it would be called a push wave, where your profile somehow maybe looks kind of similar.
But instead of it being the front of the wave that's pulling the wave, it's diffusion around the bulk. Because the bulk is the part that is actually happily growing. Because the front, here, in this case, is dying. Yet it still is possible to have a positive velocity.
And so this is, then, a qualitatively different kind of population expansion. So cooperatively growing populations expand very differently from logistically growing populations. And one of things that the reading in Physics Today talked about is these different rates of loss of heterozygocity and so forth in different populations.
And as you might expect, the pulled waves have a smaller effective population size than the pushed waves, because, here, the relevant population is at the front if it's a low density. Whereas here, the relevant population is the bulk that's at high density.
With that, I think we should quit. But I will see you on Tuesday. And we'll talk about this neutral theory in ecology. Thanks.