Flash and JavaScript are required for this feature.
Download the video from iTunes U or the Internet Archive.
Description: This lecture by Prof. Jeff Gore is on the topic of evolution in finite populations. Several aspects are covered, including the Moran process, neutral and non-neutral evolution, and stochastic extinction of beneficial mutants.
Instructor: Prof. Jeff Gore
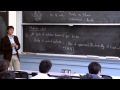
Evolution in Finite Populat...
The following content is provided under a Creative Commons license. Your support will help MIT OpenCourseWare continue to offer high quality educational resources for free. To make a donation or view additional materials from hundreds of MIT courses, visit MIT OpenCourseWare at ocw.mit.edu.
PROFESSOR: All right, why don't we go ahead and get started. So today what we want to do is start thinking a bit about evolution in finite populations. And of course, what we mean by that, is evolution in populations where we have to really think about stochastic dynamics.
And now in general, just like in the context of gene networks within cells, the situation where we have to worry about stochastic dynamics is in the small number kind of limit. What's perhaps surprising about evolution is that they're always-- the small numbers are always important. Even if you're in a large population, I'd say 10 to the 9 individuals, if you want to study evolution, then you're interested in cases where new mutants will arise in the population.
And kind of by definition, those new mutants start out as kind of a single member of the population. Which means that in the context of evolution, we always have to think about stochastic type dynamics.
Now the basic model that we're going to use in this class is the Moran process, which is a model that fixes population size. And then instead of having discrete generations, where all the individuals are reproducing at the same time-- which is what you might have seen in the Wright-Fisher process-- instead, we're going to think about the situation where it occurs more stepwise. In the sense that individuals reproduce one at a time. And then we contract the dynamics of the population.
So we're going to think about both the situation where we're trying to understand neutral dynamics, when we're tracking the composition of a population when the fitness of individuals is equal or nearly equal. But because in stochastic dynamics, there are interesting things that happen. But then we'll get into the question of non-neutral evolution. And really, we want to consider both halves of that.
All right, so in many cases, in the context of evolution, we're interested in, or focused on beneficial mutants. Now for those beneficial mutants, one of the basic things we're going to find is that even beneficial mutants will typically go extinct. It doesn't mean that they're not important over the long run. But it does mean that there is a very real sense that randomness is dominating the life of even beneficial mutants.
And then finally, if there's time, we will discuss this idea of Muller's ratchet, which is basically pointing out that if there are deleterious mutants or mutations in the population, those deleterious mutations can in some cases spread and fix in the population. And when that happens, you can have a decrease in the fitness of a population over time. And this is particularly a strong effect for small populations, because small populations, they're not as effective, what you might call filters, for selection.
And so what we want to do is start by thinking about this Moran process. And the key feature here is that we're going to have a constant population size, constant N. And that's not because we believe that real populations always have a fixed population size, but rather, we want to try to get some intuition in this simple model.
And then of course, it's reasonable to ask, well, which aspects of the mathematics or intuition we develop are going to change as a result of allowing fluctuations in the total population size? But I think there's a lot of value in starting out by analyzing the simplest model that you can.
So what we're going to think about is a situation where we have a population composed of N individuals. And for now we'll just consider two types, A and B. And this is going to be a model for asexually reproducing populations. Constant N, asexual. What that means is, in particular, that we're going to assume that an A individual can lead to two individuals. Similarity, a B individual can lead to two B individuals.
Right, so you can think about this as, for example, a model for how microbial populations may evolve. And for now, we will not consider any mutations. All right, so we're going to think about the process of assume that those mutations are already there. So A and B could be different. They could have, for example, different-- they could be different at some point mutation side of some gene that is relevant for growing a low glucose concentration, for example. OK?
So here we're going to-- so this is birth slash division, and in particular here we're going to, for now, assume no mutation. So we'll assume that A's always give birth to A's, and B's always give birth to B's.
We'll follow the nomenclature from the reading that you guys did last night, Martin Nowak's book, chapter six, where we're going to think about-- we're going to assume that there are initially i A individuals, and therefore, N minus i B individuals. Now we'll assume that the basic process for the-- in this Moran process is that you have reproduction, or birth, that's proportional to fitness.
And then the resulting kind of what you might call a daughter cell replaces one member of the population at random. So there's birth and then replacement. And indeed we'll assume that replacement, that the daughter cell, for example, could even replace the mother cell, if we want. So this is just the birth. So A is going to lead-- there's going to be two As, and this new A will have to replace one of the other individuals in the population, to keep constant population size.
All right. Are there any questions about the basic model? OK. So that, in principle, here we can use this model to try to understand both neutral and non-neutral evolution. But let's start out by thinking about the neutral case.
So in particular, the fitness, rA is equal to rB. Now what I want to do is, given the rules we just kind of laid out for you, let's assume that i over n is equal to one third. So for now, we'll say, OK a third of the population is A, 2/3 is then B.
And we can think about these probabilities of going from i to i plus 1, as compared to going from i to i minus 1. So these are the probabilities that in one cycle of birth replacement, the number of A's goes up one or goes down one. Can you ever go up two or three or four in the Moran process in one step? No. Because each step is always one birth and one replacement.
So you can move, at most, one. Do you always move-- does i change always? No. And what we want to know is the probability of going from i to i plus 1, as compared to the probability of going from i to i minus one. The ratio of these probabilities is equal to what?
We're considering a case where the A's and B's have the same fitness, so they're somehow equal per capita probability of being chosen to reproduce. But there's-- but we're not in a symmetric population distribution, right? So 1/3 of the population is A, 2/3 is B. So I'll give you 20 seconds to think about this.
All right, do you need more time? Everybody nod or shake. Do you need more time? OK. I'll give you another 10 seconds, because it's--
Let's go ahead and see where we are. Ready? Three, two, one. All right. We have a wide range of different answers here. OK, perfect. This is exactly the situation that we hope for. So turn to your neighbor. You should certainly be able to find somebody that disagrees with you. So if the first person you turn to agrees with you, try to find somebody else talk to.
All right, why don't we go ahead and reconvene. I know that there was quite a lot of disagreement, so that means that you guys will probably not be able to converge in this one minute time frame. But let me just see, let me see if anybody's mind was changed by their neighbors.
All right, let's re-vote. Ready, three, two, one. OK, all right, so it's pretty much the same as where we started, maybe. All right. OK I would say-- does anybody want to volunteer what their neighbor said? I know what your neighbors said. So tell us.
AUDIENCE: OK, so if you-- so I would say it's E. And the reason is that-- so there are two cases. In the first case, both the number A and B stay the same. Right, for example, A gets born and A dies. So you first decide, is the pop-- is the number of A and B going to change, or is it not going to change?
PROFESSOR: OK.
AUDIENCE: Once you've decided that it's not going to change-- I'm sorry. Once you've decided that it is going to change--
PROFESSOR: Right.
AUDIENCE: --then you just want to know, OK, then what's the probability that you just choose A to change [INAUDIBLE].
PROFESSOR: OK. Yeah.
AUDIENCE: And then the probability that A-- you choose--
PROFESSOR: OK. But you haven't said anything about replacement yet. So I'm-- replacement should be-- because certainly, we're talking about the ratio of the probability that the number of A goes up, as compared to the probability that the number of A goes down. Right? So we've already, in some ways, excluded the cases where the number of A individuals doesn't change.
And in your-- what you just told us, you're asking about the probability that individuals are going to be chosen to reproduce.
AUDIENCE: Yeah, because [INAUDIBLE].
PROFESSOR: OK. Yeah, but I guess all I'm saying is that there're going to be two halves to this, right? So you have to think about the probability that an individual is being chosen to reproduce, and also the probability that a particular type of individual will be chosen to get replaced. So it's the-- there's somehow a balance of those two.
AUDIENCE: [INAUDIBLE]
PROFESSOR: Right, because in this case--
AUDIENCE: [INAUDIBLE] replace--
PROFESSOR: --replace, this is-- right, death, slash-- right. And I should maybe just highlight-- if you want, you could call-- replacement-- I mean, this is just a nice way of saying death, right? Death--
AUDIENCE: Yeah, you can. I mean, [? the point ?] [? is ?] once you've ruled out-- once you say, OK, the populations are going to change, then if you choose an A to reproduce, a B has to die.
PROFESSOR: Oh, OK, once you've already--
AUDIENCE: If an A is chosen to reproduce, and an A is chosen to die, then--
PROFESSOR: OK, yeah, right. But, OK, I think I understand what you're saying. But we-- you still have to keep track of there are the two sides. There's the replace-- there's the birth, and the replacements. And we have to figure out how the relative probabilities or rates that those two things happen.
Does somebody want to make an argument for something else? I mean, we'll see how this plays out in a moment.
AUDIENCE: I want to argue for C.
PROFESSOR: OK.
AUDIENCE: So take the numerator.
PROFESSOR: Yeah.
AUDIENCE: In order to go from i to i plus 1-- so we're going to take two individuals from the population. We need one of them to be type A, that's the one that's going to reproduce.
PROFESSOR: Yep.
AUDIENCE: And the other to be type B, the one that's going to die.
PROFESSOR: Yeah.
AUDIENCE: So we get the product of those--
PROFESSOR: Perfect. OK. And we can actually just be more-- be explicit about this. OK, so the probability-- in one cycle, the probability that you go from i to i plus one. That requires that two things happen. One is that you choose an A individual to reproduce. And what's the probability that you choose an A individual to reproduce?
AUDIENCE: It's going to be i over N.
PROFESSOR: i over N. So we have i over N. Right, so this the probability that A reproduces. And then for i to go from-- to increase by one, requires not only that an A individual is chosen to reproduce, but that a B individual is chosen for replacement, or death. And what's the probability that that's going to happen?
AUDIENCE: That's N minus i all over N.
PROFESSOR: N minus i, all over N. OK. So this is the probability that in one cycle you're going to go from i to i plus 1. Now of course it's not-- we haven't said what the probability of staying in i is, but this is the probability that i will increase by one. Do we agree?
And indeed, where is it that we've assumed-- where is it that we've assumed neutrality in this calculation? That A and B have equal fitness? Yep?
AUDIENCE: Just take the probability of reproducing to be about-- or, the--
PROFESSOR: That's right. That's right. So indeed, we've-- this probability that A reproduces, we've assumed that it's just simply i over N. Whereas, if it were non-neutral we'd have to write something else. Maybe we'll figure out what that's going to be in a moment. But it's in here that we've assumed that.
Incidentally, you could write down a reasonable model similar to the Moran process, where differences in fitness show up instead of here, in the probability of reproduction, you can have it as a difference in probability of death, or being replaced. But this is the most maybe intuitive way of thinking about it.
And this is very similar to, for example, what happens in a, what you might call, a turbidostat, where you keep constant population size. And as the cells divide other cells are randomly sucked out. So I'd say that this Moran process is really a theoretical kind of implementation of what you could do experimentally, is this turbidostat. Which is like a chemostat, instead of keeping constant dilution rate, you fix population size. Yes.
AUDIENCE: So do we care about the step of, OK, first A reproduces. Then, from the pool of new individuals-- because you're going to have N plus 1, so--
PROFESSOR: OK, so all right. I think maybe I wasn't totally clear on this. OK so, you have N individuals here. What you're going to do is you're going to choose one of them randomly, maybe proportional to fitness for reproduction. And then, but then, from this original N, you choose one of them for death.
So it's not-- you're not, yes. It's not-- so the daughter cell is not allowed to--
AUDIENCE: Die.
PROFESSOR: Right. The daughter cell always replaces somebody, but it could've been the mother cell. If we're thinking about this in the context of cells. So we haven't yet figured out which answer is which, right? But we can go ahead. OK, this is the probability that A reproduces, and over here, this is the probability that a B individual is replaced. Right?
What we can do is, we can ask, well what's the probability that we go from i to i minus 1? Well it's the exact kind of same calculation, except now what we want to know is, we want to know the probability that a B is chosen for reproduction. And what is that going to be? Somebody? N minus i, right, the number of B individuals divided by the total number of individuals.
So this is the probability that a B reproduces. And then what's the probability that A-- that an A type individual will be chosen for replacement or death? That's just i over N. The number of A individuals divided by the total population size.
All right, does everybody agree with the two calculations that we just did? Let's re-vote. All right, ready, three, two, one. All right, see, you know, if we do the calculation, we can convince you. So indeed, these are equal, these two probabilities.
Right, and this is funny. Because on the one hand it's like blindingly obvious, but then the other hand, you get yourself all tied up in knots thinking about it. So I don't understand why or how those two statements can be true at the same time, but they are. So this is indeed a random walk in i space, number of A individuals.
And it sort of has to be, because these things are neutral. The fact that i over N is not equal to a half doesn't matter, because these two terms kind of cancel. But indeed, all of the things that you know have to be true based on the fact that A and B have equal fitness, they're going to not work if this thing were not equal to 1, if these two probabilities were not equal.
So any of these other answers would lead to things that you would clearly agree are going to be nonsensical, if you think through the consequence of this. And we're going to do one right now. All right, let's imagine that we start-- so here's the number of A individuals, i. I apologize that that's the nomenclature we have for a number of A individuals, but we want to be consistent with Martin's book.
Now let's say this is N and let's say we start out at some i here. The question is, what's the probability that B fixes? I want to make sure I write down some reasonable options.
So what we want to know is the probability that B fixes, and that means that it takes over eventually. That B, we'll say eventually. In the Moran process with neutral dynamics.
AUDIENCE: I's the number of A, right?
PROFESSOR: That's right. i is the number of A individuals. I'm going to give you seven more seconds. All right, ready, three, two, one.
All right, so we have-- it's kind of mostly split between C's and D's. Although I'd say a majority of the group is going to say-- is saying that it's going to be D. All right, can-- all right, and this is the distinction between the probability that B fixes and that A fixes.
I'm not trying to be super tricky, but I just want to make sure that you keep track of A's and B's. And in particular, as i increases, the probability that A fixes should go up or down? Verbally, three, two, one.
AUDIENCE: Up.
PROFESSOR: Up. This here-- over here is a bunch of A's, here is a bunch of B's. So if you have a larger here, than you should be more likely to fix the A individuals, vice versa. So in particular, this-- the probability that B eventually fixes is going to be this, whereas the probability that A will fix eventually is just going to be 1 minus that, it's i over N.
So this is indeed what was pointed out in the book. All right, and can somebody give an argument, verbally, for why the-- I mean, this is a result, that if you think about in the right way, you can just verbally say why it has to be this. Rather than writing down all the equations that-- so why is it that the probability that A will eventually fix has to be equal to i over N? Yeah.
AUDIENCE: [INAUDIBLE] book.
PROFESSOR: Yeah, perfect.
AUDIENCE: So at this given time, there are N individuals--
PROFESSOR: Yep. And there will always be N individuals, because we're keeping it--
AUDIENCE: OK, yeah, right. Their descendents, at some point, the descendents of one of them is going to take over the whole population. That's a given.
PROFESSOR: That's right. And that's fine. It's at first glance kind of surprising but, it's just the nature of-- if you imagine that they all were individually tagged, right, so it wasn't just that we had two types, A and B. But if they were all color coded using rainbow colors, then you could keep track of them. And one of the individuals will eventually fix. Now, OK, and then what's next?
AUDIENCE: So there are i individuals that are type A, so the probability that this one individual [INAUDIBLE] will fix.
PROFESSOR: And then there is one ingredient in that argument that you didn't say, but I'm sure is in your mind. Which is, how is it that the probability-- so among these N individuals, right? One of them will eventually fix. And what's the probability that each one will be the lucky ancestor for all of the population?
AUDIENCE: So it's equally distributed.
PROFESSOR: Yeah. It's just 1 over N, right? So the idea is there are N individuals in the population, they're all identical. We know that eventually one of them is going to take over the population, just due to random stochastic dynamics. What that means is that each individual has a probability of 1 over N of taking over the population.
And this is very important. So each individual has a 1 over N probability of fixing. And that's assuming that everybody in the population has the same fitness. And that's just by symmetry. But then of course, you can also say, well, you know if the probability of each individual is 1 over N, then the probability that one of these i individuals takes over is going to be i over N.
AUDIENCE: The generalization [INAUDIBLE] reproducing organisms is that [INAUDIBLE] organisms every individual is probably the ancestor of [INAUDIBLE]. Like ever individual takes [INAUDIBLE].
PROFESSOR: OK, all right. OK, now you want to allow for recombination. Is that--
AUDIENCE: I mean, yeah.
PROFESSOR: Right, OK. So yes, there are several important aspects of sex. But one of the major ones is the recombination. And so if you have enough recombination, then everybody will contribute-- well, everybody. Then there will be-- then many, many individuals will contribute to the [? lineage. ?]
What you often will hear people talk about is the ancestral Adam and the ancestral Eve. And that-- and what are people referring to about that? Yes, in the back.
AUDIENCE: An individual with the-- an individual that [INAUDIBLE] early on [INAUDIBLE].
PROFESSOR: Yes, right. So there's this idea-- OK, so I don't want to get too much into the sexual-- sexually reproducing populations because that's covered more in other classes. And it's a totally different models you would typically use.
But I think the simplest way to think about some of this is just that there's some part the genome that does not have recombination in the same way. So it's simpler. What part of the genome is that in us?
AUDIENCE: Y chromosome.
PROFESSOR: Right. So the Y chromosome, and that means that in principle you could track the dynamics along the male lineages. So there are all these studies, whatever, Genghis Khan, maybe lots of us are descendants of. Right, because he had lots of wives, or something like that. So his Y chromosome supposedly occupies a non negligible fraction of the population.
OK, so but then what about-- what's the other, yeah, so on the female side? What would be the equivalent?
AUDIENCE: Mitochondria.
PROFESSOR: Mitochondria. Right. So in principle, you can-- so I think for an awful lot of these studies you can-- the genetics are much simpler for those two lineages. Because you don't have the recombination.
AUDIENCE: So why is the mitochondria-- I mean, you say that it's the most obvious thing.
PROFESSOR: OK, yeah--
AUDIENCE: I've never heard that.
PROFESSOR: Yeah, OK, OK, right. So--
AUDIENCE: I mean, we don't have to--
PROFESSOR: OK, well, you're right. So basically the situation is that we have cells, and most of the genome is in the nucleus. But then, but the mitochondria actually have their own mitochondrial DNA.
And then the issue is, OK, well, what happens? You know, here's the birds and the bees talk for you guys, all right? Right, so the sperm comes, fertilizes the egg. And the vast majority of the mitochondria come from, or were in the egg, as compared to the mitochondria from the sperm.
And I don't-- does anybody know if any of the sperm mitochondria actually contribute? Are they selectively-- does something happen to them?
AUDIENCE: They don't have any.
PROFESSOR: Oh, they just don't have any? All right, whoo. All right, well, OK. OK, well that solves that problem. OK.
AUDIENCE: Wait.
PROFESSOR: Is that not your-- all right, well-- all right, this is the kind of thing that somebody could maybe Wikipedia this while we're going. But that's the basic idea, though.
All right, does anybody have any questions about these two statements? Probability that A fixes, probability that B fixes? Incidentally, you should be able to draw-- these are random. From this point moving forward, am I more likely-- OK, so given where i is here, am I more likely to fix B or A? Ready, three, two, one.
AUDIENCE: B.
PROFESSOR: B. Does that mean that my first step is more likely to be in the direction of B than in A? Yes or no. Ready, three, two, one.
AUDIENCE: No.
PROFESSOR: No. OK, so this is a random lock. All right, it doesn't always take steps, but sometimes it goes up and then down. All right, so it's going to- now I'm-- you know, I understand it's not to-- you know, whatever. OK.
But the idea is that once it hits 0 or 1 here in terms of the fraction, then you stay where you are. These are absorbing boundaries. But every now and then it's going to hit there.
Before we get going too much more in this, I want to mention something about time in this model. Because time is a little bit of a funny entity here. So here's the question. How long-- and long is funny-- but how long does one-- I don't know. Do we want to call this an iteration or a cycle? On iteration of the model?
And what I mean by that is that in units of something that would be like real time, you know what I mean? Is it a second, a generation time-- so this would be like a cell generation time. Or don't know, something.
OK, I'll give you 15 seconds. Can you guys all read this? Seconds, generation time, N times generation time, or 1 over N times generation time.
AUDIENCE: What do we want [INAUDIBLE]?
PROFESSOR: Yeah, well let's say that I-- let's just imagine that I was using this to model the dynamics of the neutral drift dynamics of some bacteria in my test tube in the lab. Right, so let's say I have one of these tubridostats. So I-- question is, how long does this last in the units of-- right. Or equivalent, how many iterations do I have to go to get through some period of time in the lab.
So I grow my bacteria in my turbidostat, say. And I do it for 100 hours. Now your advisor says, OK, go do a simulation, so you get something. And your advisor goes, all right, do a simulation, use the Moran process. You guys are going to be doing this, so this is not entirely hypothetical.
But right, so your advisor says, go simulate this process. Right? So the question is, how many iterations do you have to do to make it equivalent to that 100 hours that you did in the lab? How do you-- how do you make a connection between a model, well, this model, and something that actually happens in your laboratory?
Ready? Three, two, one. OK, all right, so we got a majority of the group agreeing that it's going to be D. Can somebody just say why this is?
AUDIENCE: [INAUDIBLE] one cell [INAUDIBLE].
PROFESSOR: Right, so each iteration, there's only one cell out of N that actually divide, right? And that means that if you want, like for example, everybody to have had a chance, roughly, to divide, you need to go N iterations. And it also makes sense, if you ask-- let's imagine you have a test tube with a million bacteria. Now it's going to take some time before one of them divides. Now the question is, if you had 10 million bacteria in your test tube, you have to wait 1/10 as long before the first one divides. So the amount of real time that elapses in each one of these iterations goes as 1 over N, where N is the population size. So I got some unhappy looks, so that means that I expect an unhappy question. Maybe. OK, well, if you don't ask the question, then in the teaching evaluations you're not allowed to write that you did not like the explanation of time in the Moran process.
AUDIENCE: [INAUDIBLE]
[LAUGHTER]
PROFESSOR: Well, that worked. A little bit too well.
AUDIENCE: [INAUDIBLE] question, just a clarification.
PROFESSOR: Yeah.
AUDIENCE: So an iteration is when one cell or thing increases?
PROFESSOR: Right. OK, an iteration in this model is both of these things. So it's a birth, and a death, or a replacement. So it's one duration here, another-- so each iteration involves one birth and one replacement. Yeah.
AUDIENCE: What is one generation time? Is that when the population [INAUDIBLE]?
PROFESSOR: Right, so the generation time is the typical time that it takes for one of these individuals to give birth to another individual. So in the case of the cells, it might be half an hour. I'm going to try to leave this up just so that you guys can continue to look a bit.
So I want to say just something about this idea of a molecular clock while we're here. All right, so now that we've said something about how much time is actually elapsing here, we can think a little bit about the rate-- now we want to allow mutations. So let's assume that there is a mutation rate or probability. Mu is the probability of a mutation.
And we're going to say this is a neutral mutation. And this is per division, or per birth. So the idea is that when-- all right, we might start out with just all A individuals in the population.
But then an A individual will give-- OK, here is the mother cell, the original A. And the mother cell, for now, we'll assume just doesn't ever mutate. But that the daughter cell has a probability, mu, of being a new type, say B. And we often call this a mutation rate, but it's a probability per birth.
So what we want to know is what is the what we want to calculate is what's the rate at which new neutral mutants both appear and then fix in the population. So what we're asking about is, from the standpoint of us as scientists, we do sequencing of different lineages, say humans and chimpanzees. And we're looking at the accumulation of these, what we think are neutral mutations.
Question is, how many neutral mutations do we expect to see? So we need to know the rate that these things happen. So this is the rate of fixation of neutral mutations, and so this is somehow the rate of neutral evolution. There are two steps in here. What are the two things that have to happen?
AUDIENCE: [INAUDIBLE]
PROFESSOR: Right, needs to appear. So we'll call this the rate of appearance. And then what else do we need to know?
AUDIENCE: [INAUDIBLE]
PROFESSOR: I'm sorry, what's that?
AUDIENCE: Population size.
PROFESSOR: Right, so the population size. And why are you saying that? Or what's-- I mean, the population size is certainly going to be relevant, but I guess the question is, will the rate of neutral evolution, the rate in which you see neutral mutants in a lineage, will that be just equal to this, or do we need to multiply it by something else? Yeah.
AUDIENCE: [INAUDIBLE]
PROFESSOR: Right. And it's a rate of fixation. We'll say it's really kind of a probability of fixation. Because there's some rate per unit time. Maybe even like real time in terms of number of generations in real time. But we need to know the probability that it fixes.
Great. So let's-- all right, we're going to do-- we're going to do a very detailed calculation here. Yes.
AUDIENCE: So I'm just curious, [INAUDIBLE] for this probability [INAUDIBLE] time is completely left out of the picture, so in principle--
PROFESSOR: That's right.
AUDIENCE: --it could take a very long time.
PROFESSOR: That's right.
AUDIENCE: But we don't take this into account because--
PROFESSOR: That's right. So for now, let's just assume that the rate of appearance of these is small, so that you don't have to worry about different mutants competing against each other. We're going to spend a lot of time on Thursday talking about this phenomenon of clonal interference, when multiple mutant lineages are coexisting and perhaps competing in a population.
But for simplicity for now, what we're just going to assume is that there's a separation of time scales. Right? Which means that the rate at which these neutral mutants appear in the population is very small compared to the 1 over the time that it takes for the fixation to occur.
So what we want is what's the rate of appearance here in units of real time. What are the things that are going to appear here?
AUDIENCE: Rate of mutation.
PROFESSOR: All right, rate of mutation, mu, times population size. And that's just because a larger population will experience a larger rate of these mutants appearing in the population. And it's a linear. And that's actually just-- that is, indeed, the rate of appearance.
And the probability of fixation of each one?
AUDIENCE: [? Excuse me, ?] but it doesn't have the unit of rate.
PROFESSOR: Oh, yes. OK, so this is-- OK so we have to actually--
AUDIENCE: [INAUDIBLE] times is that per iteration?
PROFESSOR: Yes. So this is per, this is per-- so this is a rate per generation. So I guess I'd define mu as the probability-- so this is all in units of per generation, basically. Because mu is a per.
AUDIENCE: Generation or iteration?
PROFESSOR: OK, let's make sure that I-- mu-- this is a generation. Because if we have-- right. So let's say that, for example, there's 10 to the 6 individuals here, and the mutation rate is-- yeah.
All right, probability of fixation was what?
AUDIENCE: 1 over N.
PROFESSOR: 1 over N. So this is great. Because this is saying that the rate at which you expect neutral mutants to actually appear in the population, in terms of like, in terms of fixing, if you were to sequence along a lineage, that it is independent of the population size. And it's given by the rate of mutation. But what you expect is it's on-- it should be on a per generation basis.
So this thing is perhaps useful in several different ways. And there are some subtleties, like always, to this. If you go out and you measure the rates of fixation of neutral mutants, what you find is that it's not really constant on a per generation basis. But more on a-- maybe even closer on a per actual year basis, say.
In particular, this would predict that if organisms have the same mutation rate, I'd say roughly maybe humans and mice. But yet humans and mice have very different generation times. By [INAUDIBLE]. Then you would expect the rate of accumulation of neutral mutants in the human population on a per year basis to be much lower than mice. But that's not true.
We'll get into a bit later why that might be. But I just want to highlight that that's-- that this model is very simple. And it predicts something that is too simple, maybe. But at least it's saying that there's some sense in which the population size is not as relevant as you might have thought it was going to be.
And at least within a particular lineage, if you're talking about the accumulation of neutral mutations along humans, for example, then you can say, maybe that's roughly constant. It gets very-- it gets very tricky. I mean, if you look at the rate of accumulation of neutral mutants in one protein in humans, it's at a different rate than another protein in humans.
So everything is complicated. But at least each-- along each of these proteins, maybe it still is roughly some sort of clock, because it accumulates mutations at some rate that's roughly linear with time. Of course, it's hard to imagine how any process like this can not go with time like that. But at least this is potentially a useful thing.
And indeed, when you read about studies from sequences trying to estimate the time since the last common ancestor, this is the category of technique that is the basis for that, is that you're just counting up how many neutral mutations appeared along these along these different lineages.
And I think that there are a number of really fascinating things that you can try to address with this kind of molecular clock. And I'll maybe bring up one of them. Incidentally, I'm not a huge fan of memorizing things. But for both size scales, and time scales, and so forth, I really very much do like the idea of everybody having memorized a few sign posts.
Because that way, when you hear something new, you have some way of interpreting whether it's big, or small, or something else. So for example, the time since the last common ancestor between humans and chimpanzees. Does anybody have any sense of-- well, I'll actually have us vote, because I think it's useful. In case you're off by many orders of magnitude, that you make-- OK.
So the last common ancestor, human, chimpanzee. And incidentally, this is something that people do argue a lot about. But it's within a factor of two of something. So I'm going to go ahead and make some-- so hold on. I just want to make sure I get my-- well, 7 times 10 to the 6.
All right. All right, I'll give you 10 seconds to orient yourself relative to other things that you might know about the world. OK, ready, three, two, one. All right, we got-- that's interesting.
OK, yes. I would say it's kind of uniformly distributed-- oh. It's pretty unified. There are a minority of-- not very many E's. But I would say that the other things are pretty-- it's maybe peaked around here.
I don't want to get into any biblical debates here. But right, OK. So what are some things that if we have a timeline of the world. OK, this is going to be a flash course in-- all right. OK, here's, here I am, and I'm unhappy because we don't know when humans and chimps-- all right. So this is us. All right.
AUDIENCE: [INAUDIBLE]
PROFESSOR: Right. OK. So let's say-- we could start with-- you want to start with earth? OK. Four and a half billion. This might be on a logarithmic scale, somehow. So we're going to-- just to space things out a little bit.
AUDIENCE: [INAUDIBLE]
PROFESSOR: Right, you know, the universe is what, 13 ish billion years? I don't know. People are calculating with these-- 13 billion, four and a half billion, you know earth congeals, it's hot, whatever. All right, so life gets started, maybe, a billion years later.
AUDIENCE: 3.9 [INAUDIBLE].
PROFESSOR: 3.9 sounds like a fine number. Wait, what did you vote for human and chimpanzee? You're very specific on this one.
AUDIENCE: I actually voted for 3.5 times [INAUDIBLE].
PROFESSOR: OK, all right. So you're-- you want to get involved in this actual debate. OK, that's why. I just want to-- yeah, now I'm going to be stuck doing the linear and logarithmic scaling of how I want to-- this is going to some sort of funny logarithmic scaling from here to here.
So dinosaurs-- all right, 60 some million years ago. Right, so, say bye to the dinosaurs. Dinosaurs. [INAUDIBLE]
AUDIENCE: [INAUDIBLE]
PROFESSOR: [INAUDIBLE] explosion was before that. Yeah, I don't-- OK, it's-- OK, all right. All right, so this is around human chimp. And indeed, people argue about whether it's five or 10. But you know, given that we were uniformly distributed across this number, we shouldn't be nit picky about the left one.
Right, OK. And agriculture was maybe 12,000 years ago. Some sense of things.
AUDIENCE: B is good for human and Neanderthal, and homo erectus.
PROFESSOR: Yeah, human and Neanderthals, right. That's-- 70, OK, let's say yeah. This is when-- right.
AUDIENCE: Common ancestor was definitely [INAUDIBLE].
PROFESSOR: Oh, common ancestor was before. But in terms of interbreeding, was sort-- all right. So this was the interbreeding, if you want to read that paper. But human chimp is here. Around seven million years.
And it's not that this is the number that's magical, that you have to memorize. But I think that you should have some event in the history of the world at each logarithmic spacing. Just so that you-- when you hear about when something happened you know kind of vaguely where to put-- where to put something.
Otherwise it just doesn't mean anything. One of my favorite examples of how the molecular clock was used to come up with something that I think is pretty neat and nontrivial, is to try to answer this question of when humans started wearing clothing.
So this is, a priori, not very obvious. Right? Because we know we have evidence for clothing maybe 30,000 years ago. And there are needles that were used for clothing. There were-- and some of these little figurines, at least some fraction of the figurines, like fertility goddess kind of thing, some fraction of them have some clothing, right? So then it suggests that there were clothes. But the question is, before that it's actually rather difficult to know when we started wearing clothes, right? Apparently, we lost our body hair something like a million years ago. So you might say, oh, maybe that's around when we started wearing clothes. Of course a lot of animal hide and so forth wouldn't last. So there's not any archaeological evidence of this.
And so is anybody aware of how researchers have used the molecular clock ideas in order to try to answer this question?
AUDIENCE: [INAUDIBLE]
PROFESSOR: Yeah, this is amazing. So you use lice. There have been a number of studies doing this, and apparently, there was a researcher in Germany, who was at the Max Planck Institute for genomics or something, and his son came home with a note saying-- and actually this happened to me recently, they got an email that there's a lice outbreak to stay out of preschool, so watch out when you're going by the play area-- so he got this note back from his son's preschool that said, oh yeah, there's a lice outbreak, so this is what you have to watch out for.
But it said, oh, there's a different species of lice that inhabits our clothing as our hair. All right, so I'd say this is one of those things that you could just read that and say, oh, well whatever. Or if you're a geneticist you read that and say, oh, I can use this to figure out when humans started wearing clothes, right? Because presumably the species that specializes in living in our clothing was probably not there or had not yet speciated before we had clothes.
Course, you can imagine ways that this could fail, but it's a neat hypothesis. So then you can go and you can basically sequence the species of lice that lives in our clothing as compared to the kind that lives in our hair, and you can ask, how many neutral mutations accumulated along these different lineages.
Now, you can imagine that based on, since we just did this very nice study, we know that it should be more than 30,000 years and it should be less than 7 million, probably, hopefully. Although, it's always possible that our ancestral state was wearing clothes and that the chimpanzees stopped wearing clothes. But we'd be surprised if that were the case. All right, so this is basically just asking about head lice versus clothing lice. And the original study by this researcher Max Planck estimated 70,000 years, but then just a couple years ago there was another publication from a professor at the University of Florida that estimated 170,000.
So there still is a fair range, but I guess the most recent estimate we'd have to say is 170,000. Which is neat. I don't know-- it's not that it changes, necessarily, how I go about my daily life, but I really love this idea that it's a very basic question that your toddler son might ask you-- something that you'd think that might be totally unknowable in the sense that we would never have any way of getting any estimate all, right? But using some clever theoretical ideas together with data on this accumulation of neutral mutations allows one to at least make a ballpark estimate of something that there's no physical record of except in the DNA of our louses. Is that a word?
AUDIENCE: It's lice.
PROFESSOR: It's just lice? All right. Are there any questions about this point? So this is all neutral mutation, but of course we'd like to move beyond these neutral mutations to try to understand how non-neutral mutations spread. I'm not going to do the derivation, because the derivation is in your book, and you just read about it. But I do want to just make sure that we understand what this equation is telling us.
So first of all we're going to assume that A has some relative fitness r. So r is defined as basically the relative fitness of of A, or the fitness of A divided by the fitness of B. So r is greater than 1 means that A is advantageous. Less than 1 means it's deleterious. And what we're told is that x sub i, which is the probability that A fixes, is equal to this expression. If A fixes, given i A individuals and N minus i B individuals.
AUDIENCE: So I guess that this assumes that they die at the same rate [INAUDIBLE].
PROFESSOR: That's right. That's right. The assumption is that we're placement is unbiased, purely random, and it's only birth that is different by a factor of r.
And so I think that this is, on one level, wonderful. It's kind of a simple expression describing a lot of information of the dynamics of the stochastic process. On another level, the problem is that you look at it, and I think it's easy to have like absolutely zero intuition for what this thing does. So what I always like to do when a student comes to my office and says, oh I derived something great for our project. You take a few limits to get a sense of what's going on with it. At half-time you find that it's not true. But at least it's a way of developing intuition for what's happening.
All right, so what are limits that this thing should behave--
AUDIENCE: It should be 0 if there's no A.
PROFESSOR: Right, so x of 0 should be equal to 0, is what you're saying. If you have 0 individuals, you should have 0 probability of fixing, independent of your fitness, right? All right, that sounds like a reasonable thing to check. And does it work? So r to the 0 is equal to 1. So that's 1, so it's 1 minus 1-- 0. Yep. Yes?
AUDIENCE: r goes to infinity independently of what you start with in step zero, then you expect A to fix?
PROFESSOR: That's right. So the limit of xi for any i other than 0-- as r goes to infinity, this should be equal to 1. All right, so let's see. If r goes to infinity, you get 0-- this is also 0-- 1 divided by 1 is equal to 1-- all right. Did everybody agree with that?
And that makes sense just that if A is just super, super fit, then it should fix. And of course, what's tricky here is that r has to be surprisingly large before this thing ends up being true. This limit is great, and it's correct and true, but it's also a little bit dangerous because-- well we'll see that even things that you think of as being very beneficial mutations typically do not fix. So this is the danger, but at least the limit is still true. Any other limits that we think ought to happen?
AUDIENCE: If an i goes to N?
PROFESSOR: An i goes to N? OK, right. This is the opposite of this one. This is just saying that if you already have fixed then you fixed. Indeed, if i is equal to N-- that works. Any other limits that you believe should be true, think should be true?
AUDIENCE: The one we already checked for r equals one?
PROFESSOR: Yes. Indeed. So if it's neutral-- so the limit as r goes to 1 of xi should be equal to what?
AUDIENCE: i/N.
PROFESSOR: Should be equal to i/N. So this one is a little bit less obvious, because if you set r equal to 1, does this mean that it's equal to 0? And what's the problem?
[INTERPOSING VOICES]
PROFESSOR: Well, OK, but even that statement's not true. It's not even necessarily close to 0.
AUDIENCE: [INAUDIBLE] L'Hopitals?
PROFESSOR: Right. This is the L'Hopitals. There was another context already were L'Hopitals came up, right? Maybe? OK, so the problem is that if you set r equal to 1 here, then you get 0. So then you think, oh, the answer is 0. But you have to be more careful than that, because this also is equal to 0. And so L'Hopital's-- L-H- -- is it above the H?
AUDIENCE: No, that looks right.
PROFESSOR: Is it good?
AUDIENCE: Yes.
PROFESSOR: All right. You're French, right? I mean, sort of. He's from Quebec, so I don't know what that question-- how it's interpreted.
So this [INAUDIBLE] looks all right. See, what you just have to do is then you take the derivative with respect to r for both the numerator and the denominator, and then you see what ha-- but you take the limit again. And sometimes you have to apply L'Hopital's rule multiple times, right? So what we write here is this is the limit as r goes to 1, and we take the derivative of the numerator respect to r. So we get out an i, 1 over r to the i plus 1, maybe? And here we get out an N.
All right, so we took the derivatives back to r here. But we left it as a limit because we might need to apply it again, right? Just because after you take the derivative you're not guaranteed that it's going to work out fine, but in this case it does. Because already, this limit, we're allowed to just set equal to 1 because nothing blows up. So this is indeed equal to i/N. N. And the important point here is that it's not necessarily approximately equal to 0. It could be anywhere between 0 and 1 depending what i and N are.
PROFESSOR: So that means that this expression here captures the dynamics, actually, for all i, r, N within the Moran process. This thing is simply just true in this model. There are no approximations yet. There is, however, one approximation that is very useful to make, which is the approximation of what happens when r is approximately 1.
In particular what we're going to ask is, if we define something called a selection coefficient, that is 1 plus s, the idea here is that in many cases-- well for Thursday we're going to read a paper that I think this is quite interesting. And where they were analyzing the appearance of these mutations that would allow bacteria to survive in some environment to do better.
And typical selection coefficients here are kind of 1% to 3%. So the mutations that appear and that allow one of these cells to do better in this new environment, convert an advantage that was on the order of 1% or 2%, or so. Which means that s here would be like 0.01, 0.02. Which means that for basically all the situations that you see in the laboratory and so forth, what you really want to know is what happens for small s. So for s, much less than 1. So where r is approximately equal to 1.
And in this case, we can say xi, well-- and we actually are going to want to ask about x sub 1. So that's a 1 now. And the reason for that is that we want to know, are there some rate that new mutations will appear in the population? When they appear they'll be present in a single individual, and we want to know what is the probability that one individual-- let's say has a beneficial mutation, well, the probability it'll fix-- so we want to know is for s, much less than 1, but larger than 0.
So far, it's a beneficial mutation of modest effect. What's the probability that it will fix? Well the idea here is that r to the N is going to be much larger than 1, because N is often a big population.
Now in that situation, this is just approximately equal to 1 over r. And r, we've already decided it can be expressed as 1 plus the selection coefficient. Now this is something that you want to be able to simplify in your sleep. 1 divided by 1 plus s is approximately equal to 1 minus 1 minus s. And this is indeed approximately equal to s.
This is saying that in the Moran process, if a beneficial mutation appears in the population with selection code coefficient s, that might be 1% to 3%, then it has a 1% to 3% probability of surviving. Because this is the probability of fixing, but in this situation fixation and survival are the same thing, because we're just considering this one mutation. So it's the only thing that we're considering is the fate of this one mutation of the population.
We're going to assume for now that you can't get new mutations in the population to compete with. And then either you go extinct, or you take over the population. And what's surprising here is that even if it's a fact that you think it was big, like 3%, 4%. I would love to get such a mutation. But still, in a population in the Moran process, or really in any other model like this, it will typically go extinct.
Now, it's worth saying that depending upon the model that you're using, you'll get different numbers in here. In this case, the probability of fixation or survival is 1 times s. But in some other models and it depends on the branching process. It could be two times s. But it's something of order unity times s.
AUDIENCE: And so we say that if s is equal to 0, then x of 1 should be--
[INTERPOSING VOICES]
PROFESSOR: Right. Exactly.
AUDIENCE: [INAUDIBLE] assume that s is much, much greater than 1/N?
PROFESSOR: Exactly. So what we've assumed is that this is true and that I think that's actually already sufficient. And indeed, you can go and you can do the expansion and find what x sub 1 should be equal to in general here. And for small s, but including the possibility that it's very-- I'm sorry-- for small s but not super small s. It's a matter of what you're comparing to.
And you end up getting 1/N plus s/2. And this is for s times N much less than 1. And indeed, this is the definition of what we mean by nearly neutral. Because up to now we've been talking about neutral mutations as if they just had to be exactly, exactly neutral, but then really, that probably doesn't actually literally exist. But if a mutation appears and it only changes your fitness by one part in 10 to the minus 30, then it is equivalent to being a truly neutral mutation. But the way that you quantify that is this thing. s times N. You want to know whether it's larger or smaller than 1.
So for example if you plot x sub 1 as a function of s-- 0, right? It crosses 1 over N here. Now, it's going to have a slope here-- s over 2-- but then it kind of goes up. It eventually hits this-- this is just the s line-- because if s is much less than 1, yet s times N is much larger than 1, then x1 is approximately equal to s. And then over here, this goes down exponentially.
But the statement is that if s times N is much less than 1, then the mutation acts essentially as if it's neutral. So then you can just work with that, whereas, if s times N is much larger than 1, then you end up-- it's not guaranteed that it's going to fix, but it's much larger than a probability of 1/N. Whereas down here, it becomes very unlikely to fix once s times N is larger than 1 on the deleterial side.
Are there any questions about what's going on here? Yes.
AUDIENCE: I'm wondering about that factor of 1/2. [INAUDIBLE] seems like we didn't get it over here when we had N equals--
PROFESSOR: That's right. Yeah, sorry. And indeed, when I did this calculation originally, I was very confused, because I thought it should be that. But what you see is that if you plot this, the slope here, which is the 1/2, is less than slope over here, which is 1.
AUDIENCE: [INAUDIBLE]
PROFESSOR: Well, what's funny is, I actually spent, like, hours, trying to figure out where I had made my mistakes. No, but I think that if you just draw it, I think it's all consistent.
AUDIENCE: [INAUDIBLE]
PROFESSOR: I mean, I'm plotting the entire curve, analytically, perfectly.
AUDIENCE: [INAUDIBLE]
PROFESSOR: It's just that what we know, is that it behaves like this around here, and like s up here, and I've just connected them-- it probably doesn't answer your question but it--
So I think we have just enough time to say something about this Muller's ratchet idea. Verbally, can a deleterious mutation, s less than 0, can it fix in a population? Yes or no? Ready, three, two, one.
AUDIENCE: Yes.
PROFESSOR: Yeah. This is greater than 0. Now it's, very unlikely to fix if the negative s times N is much larger than 1. But for small size populations, you could actually fix relatively deleterious mutations. So the idea is that for small populations, it's easy to accumulate deleterious mutations.
And indeed, This is related to something called a mutation accumulation assay. So if you take a population of bacteria or other microorganism-- so you grow up your bacteria in a test tube. And so you have a bunch of bacteria. So now there's selection acting in here, because the faster dividing cells are spreading.
However, what you do is that you then plate them as colonies. And these colonies each started as single cells. Then what you do is you just take a random cell, a random colony, that came from a single cell, and you grow it up here. Or you could just replay directly if you like. And then you just repeat this process.
The idea is that you've picked a random cell from this population that you allowed it to grow up, and so you've kind of removed the effects of selection in here. So when you pick a random colony here, maybe that colony got some weird mutation that decreased its fitness, but it wasn't really selected against because you just kind of picked one of these colonies randomly. So this kind of process is a way of reducing what's known as the effective population size-- N effective.
So when populations are not constant in time, but instead oscillate or fluctuate, then in many cases the dynamics or the strength of this drift, or the stochastic stuff, that could be characterized by some ineffective. And of course, depending on which variable or which quantity you're trying to study, you might have a slightly different N effective. But the point is that if you have fluctuating population sizes, then the relevant population size for thinking about these sorts of ideas, is often towards the smaller side of the range of the fluctuating population.
So you're kind of dominated by how small the bottleneck gets. And here, you're kind of going through a single cell bottleneck, so that leads to a very small N effective, and it allows for the accumulation of deleterious mutations.
Now, we'll say something more about this Muller's ratchet idea, because in the field of evolution, I'd say one of the big overriding questions is trying to understand the evolutionary advantages of sexual reproduction. Because of this famous twofold cost of sex. That it seems that an asexual population should be able to grow twice as fast as a sexually reproducing population, because the males are not giving birth.
So it's a huge cost associated with sexual reproduction. The question is, why is it that so many organisms engage in it? And one of the explanations that's been proposed is this Muller's ratchet idea, that it may alleviate this accumulation of deleterious mutations. We'll talk more about how this works out, maybe quantitatively, and the other proposals and so forth later, but you may, over the course of your studies, come across this Muller's ratchet vis-a-vis the question of sexual reproduction. So I just wanted you to know that there is this discussion out there, about how sexual reproduction may allow for the separation of these beneficial and deleterious mutations that would otherwise accumulate in the population.