Flash and JavaScript are required for this feature.
Download the video from iTunes U or the Internet Archive.
Description: In this lecture, Prof. Jeff Gore discusses the stability, resilience, and diversity of populations at a systems level. He begins by considering a single population, and then moves on to a simple model of interactions between species.
Instructor: Prof. Jeff Gore
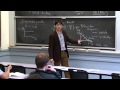
Ecosystem Stability, Critic...
The following content is provided under a Creative Commons license. Your support will help MIT OpenCourseWare continue to offer high quality educational resources for free. To make a donation, or view additional materials from hundreds of MIT courses, visit MIT OpenCourseWare at ocw.mit.edu.
PROFESSOR: Today, our goal is to discuss some concepts in this question of a kind of population or ecosystem level stability, resilience, and associated sudden transitions in populations. And something about diversity of populations. We will revisit this question of diversity more on the last class, where we're going to discuss some of these ideas from the neutral theory in ecology, but it'll be a bit of a preview today.
So what we're going to do is start out by just considering the dynamics of just a single population, where you have maybe a one population that's growing either logistically, or via some cooperative kind of dynamic. So, single population. And in here, we're going to understand something about this idea of either logistic growth as compared to something called the Allee effect, whereby the population has some cooperative type dynamic. So this is maybe logistic versus the Allee effect. And try to understand how this Allee effect can lead to the sudden transitions that you read about in the review.
And then we'll say something about the Lotka-Volterra competition model. So this is a slightly different model from the Lotka-Volterra predator-prey model that we've all been thinking about recently over the last week or so. But it's again, kind of the simplest model you might write down that captures this idea of interactions between species. In particular, competitive interactions.
Then at the end, and depending on how much time we have, we'll either finish it or not. We'll talk about some these non transitive interactions. The so-called rock, paper, scissors, RPS type interactions. And some of the measurements that have been made both in male mating strategies in lizards in the California mountains, as well as kind of a rock, paper, scissors type dynamic that was explored experimentally by Benjamin Kerr in the context of bacterial toxin production, or chemical warfare bacteria.
And this subject does come in a bit into this question of spatial structure, and how spatial structure may help facilitate the stability of populations. So there's this idea of that non-transitive interactions, whether you have Rock, Paper, Scissor type interactions, may facilitate coexistence of either genotypes or species. But in some of these studies, there's an argument that sometimes this non-transitive interaction may not be enough, but then in the presence of spatial structure, maybe it does help. On Thursday, the primary topic of the class will be trying to understand the dynamics of populations when their spatially extended. So this will transition naturally into the subject on Thursday.
Are there any questions about where we are here? Or administrative type issues?
All right, so I just want to make sure that we're first, all on the same page. We talk about logistic growth. So we can think about-- Well, what are the simplest ways that we might consider the population dynamics of just a single population. Well, the logistic growth is maybe the simplest model you might write down, where the population is at least bounded. And I might even-- Maybe I'll even just first draw exponential growth, just so that we can--
The very simplest thing you might do is you might just say that N dot might be equal to some r times n. In this case, if we plotted gamma, the per capita rate of population growth, this is just some line at r. In this case, the population grows exponentially. And that that means that if you plot say the number as a function of time, well it doesn't matter where you start, you always go to infinity. Right?
Now we can compare this to the logistic growth case, where we just assume that at low density, indeed, we grow exponentially at this rate r, but then we have a linear decrease in this per capita rate of growth as a function of density. So we might write this as something that looks like this. In which case, if we write this per capita growth rate of the population, as a function of population size, it starts out at r, but then it goes to 0. And indeed you, might even if you'd like, you could say that it goes down to a negative value. So in that case, you could start at a population size above the carrying capacity k, and you still come back down it.
One of things that we want to make sure we understand is what the bifurcation structure of these equations is going to look like. In particular, we can think about what happens if we add some sort of death rate to the population. Right now, in this case, a death rate corresponds to is something-- OK, we're looking at the bifurcation diagram. What we're going to do is we're going to think about what happens if you look at the size of the population. In the bifurcation diagram, what we typically do is we look at the fixed points. The fixed points in n, as a function of some external parameter. And here, we might just think about delta here which is just some death rate. The simplest way to include this here would be to have a minus delta n.
So the idea is that there's this population. It grows exponentially at small sizes. As the population size grows, it might run out of nutrients or so, and that limits its overall population size. Then, we can add another term here which is corresponding to something about the quality of the environment. So this could be the amount of hunting that is taking place, or it could be a reflection of pollutants in the environment or so. And what we want to do is try to make sure we understand how the size of the population will respond to some death rate.
Well maybe we'll go ahead and do a verbal vote. So in the review that you guys read, this was talking about in these early warning indicators in the context of sudden transitions and populations. So this was saying that whether you're looking at a population, or an ecosystem, or maybe even other complex system, such as climate regime shifts, epileptic seizures, and so forth, the authors were arguing that in response to a slowly changing environmental knob, the system can experience a sudden transition in the state.
Now, the question is here. And the sudden transitions that they were describing, those were fold bifurcations, or saddle-node bifurcations. Where there's a sudden transition in say, the equilibrium. Size of the population is a function of some external knob describing the quality of the environment.
So the question is whether this population experiences such a full bifurcation at least to a sudden transition. Do understand the question? I'll say fold bifurcation. I'll let you guys vote, just so you can use your cards. And this is a full bifurcation as a function of this delta, which is death rate.
Ready? Three, two, one. We have a majority now that are saying the answer is no, this does not actually have a fold bifurcation.
And the reason for that is that we can find the-- In the absence of a death rate, what is the equilibrium population size? k, right? Indeed, what's going to happen is that as we add this death rate, we can just figure out, OK well, the fixed points occur when N dots equal to zero. All of these things. There's an n here, we can divide by that. Well we could just do it, just so that we're all on the same page. So the fixed point.
What we want to do is set N dot equal to zero. That tells us that n times r1 minus n over k minus delta is equal to 0. OK. How many fixed points are there going to be in the system? Two. Right, so indeed, this is N dot. So n equal to zero is going to be one fixed point, but the other one is going to be when this thing is equal to zero. And so then we end up with 1 minus n over k, is going to be equal to some delta over r.
So we can see that this stable fixed point is going to decrease linearly with this death rate delta. And it's just going to go smoothly to zero. That's this key feature of this bifurcation digraph. So what happens is that we come down like this, and indeed, we could even continue this and the bifurcation occurs here. We want to draw-- This corresponds to a stable fixed point. And a dashed line is unstable. In this case, this is the stable and this is the unstable.
So this thing here is what's known as a trans-critical bifurcation. And it's a bifurcation because the fixed points do something. In this case, they exchange stability. So what happens is that this thing becomes unstable, whereas the fixed point at n equals 0 becomes a stable fixed point.
So there's no tipping point or sudden transition in this logistic growth as you change the death rate. All right, you can think of it as a situation here where the death rate just kind of starts pulling this thing down. And this bifurcation occurs when you pull it down-- when delta is equal to r.
And what this means is that if you plot say, the number as a function of time, for low delta, in particular, for delta equal to zero then this is your-- You come to this equilibrium k. Where as delta increases, eventually you just get to a situation where all of, regardless of where you start, you go extinct.
Now, in many cases, we don't draw what happens down here because n being less than 0 is not a physical thing. But it's useful to think about it mathematically, because that's what tells you that that was the bifurcation. Are there any questions about where we are here?
One thing that's valuable in the case of-- well, in general-- is to switch between the algebraic characterization of the dynamics a system and the graphical dynamics, because you end up seeing different things depending on how you do it.
For the case of the Allee effect, we're just going to look at it and analyze it graphically, just because the equation is a little bit cumbersome, and I think don't provide very much intuition. What you can see is that for the logistic growth, other individuals in the population always decrease your happiness as an individual. So if you imagine that you are some member of this population, and having more members the population there is always kind of bad for you, because it decreases your growth rate. And to think about the happiness of an individual, what you typically want to do is divide by n, because you're thinking about the per capita growth rate. So that's telling you about the ability hat you will have to reproduce. So what you did we do is just divide by n.
What you can see is that per capita division, gamma, is a monotonically decreasing function of the population size. This is saying that more individuals in the population just take up space, resources, mate, something. And so you as an individual never benefit from the presence of other individuals.
Whereas, when you have the Allee effect, what that's saying is that over some range of population size or densities, there is a positive effect of other individuals in the population. This is saying that if we plot gamma, in particular, the derivative of gamma with respect to n is greater than 0 for some n. This is just saying that if we plot this per capita division rate as a function of the size of the population, there's some region in which this thing has positive slope.
And often, people distinguish between the so-called strong Allee effect and the weak Allee effect. The strong Allee effect corresponds to the situation where at n equal to zero, you start out with negative per capita growth rates. So this is just some generic curve here, that describes the Allee effect. The important thing is that it's coming up here at low n.
And what this tells us is that we will have some sort of different dynamics where this is going to be the stable size of the population here. But there's also a minimal size required for survival. So if you start out below this n, then you come down. If you want you want, you could call it some-- This could be some k, and this could be n-min.
This is saying if we plot n as a function of time starting with different sizes of the population, then indeed, this thing is a stable state. But the key thing is that there's this other minimal size required for survival. So if you start out around here, then just above it you come up, but just below it, you come down.
What do you say? What you see is that this population that experiences the strong Allee effect has bistable fates. So it's bistable. This is bistable depending on the starting size n of the population.
Now, can somebody suggest possible explanations for why there might an Allee effect?
AUDIENCE: Sexual reproduction?
PROFESSOR: Sexual reproduction. And can you explain that a little bit more?
AUDIENCE: [INAUDIBLE]
PROFESSOR: That's right. And basically, just the need to find mates. So this implies that at some density, sexual reproducing species are expected to have this Allee effect. It doesn't mean that the Allee fact is super strong at moderate sizes, right? I think that this statement is true, but it might lead us to conclude that these extreme dynamics that we're talking about here are relevant for every sexually reproducing species.
But ultimately, the question is maybe how big is this n-min? If n-min is two, then it's not a severe constraint on the population. Whereas if n-min is-- if you need to have 100 California condors in order to have a viable population, then that's a problem. So there really is a quantitative question of how big is this minimal size. Other suggestions of why it is you might have an Allee effect?
AUDIENCE: The animal species in groups?
PROFESSOR: That's right. Group hunting. And are you aware of any cases that have group hunting ?
AUDIENCE: Wolves?
PROFESSOR: That's right, so, exactly. Right, so wolves for example, can take down like bison, of large animals they would never be able to take down on their own. So this could be this could be wolves, it could be primates. We historically did-- I guess we still have some groups. Societies will have things like this. But even at the microscopic realm, if you look at just bacteria or yeast and so forth, there are often cases where food is generated or broken down outside of the cell. So any of these cases where you have secreted enzymatic breakdown.
That was an i. Other thoughts? Yes?
AUDIENCE: Protection from predators?
PROFESSOR: Right, protection. And so this is the flip side of this, so this would be predator avoidance. Or an example of that would be what?
AUDIENCE: Antelopes.
PROFESSOR: What do antelopes do?
AUDIENCE: They run in groups.
PROFESSOR: That's right. So the standard example here is kind of what herding behavior of land animals, schooling behavior of fish. Of course, researchers argue about to what degree the benefits are primarily this, versus something else. I am not going to weigh in on that debate. But, I'd be surprised if this were never true.
In all these examples, there's clearly something cooperative about the dynamics. In that sense, it kind of makes sense to think there's some element of cooperative growth, whether it's on the hunting side, or the protection side. So if you look at all these cases these would all be very naturally described as some sort of cooperation. I do want to highlight though, that the Allee effect can be caused by things that lead to what you might call effective cooperation. So it's not like obviously cooperative, or not intentionally cooperative, but still has the same effect.
A nice example of this is what we call predator satiation. Can somebody guess what this might mean? Somebody's laughing so it's--
AUDIENCE: If there are enough animals around, the predator will be fed.
PROFESSOR: That's right. If the predators gets full, then you can end up with something that's like this. And the way I think about this is just in a group like this, if 20 bears come in and eat 20 of us, then we hope that there are 20 other people that will get eaten first, so that you know that the leftovers can run off. Right?
This is embodied in that joke that people tell when they're the two hikers in the woods, and they see the mother bear that's angry and one of the hikers reaches down and starts tying his shoelaces. And the other thing the hiking partner says why are you tying your shoelaces? You can't outrun a bear, right? And the guys says, well yeah I know that, but I only have to outrun you. That's predator satiation. So if the predator gets full, then you can get something that looks like this. It's below some size, below this n-min size, they all get eaten by that bear. And above it, the population may be able to grow. So this is a good example of just that it's an effective form of cooperation.
Now can somebody explain-- Well, first of all, does this lead to a full bifurcation if we add a death rate? We're going to think about this first seven seconds, then we'll vote. The question: if we add a death rate to this, does that lead to a fold or saddle-node bifurcation?
I'm going to give you more than seven seconds, because I see a lot of brain activity. Does this one lead to a full bifurcation? Ready? Three, two, one. The answer is, in this case, yes. So we'll say Allee effect is in general the kind of thing that leads to a full bifurcation. In this case, it definitely does.
So it's really very important to be able to take curves like this, and figure out what the bifurcation diagram looks like. And just remember that the solid and dashed lines here are very useful for getting intuition on what's going on, because stable is telling us that any perturbation away from this point, it goes away. So this is why we draw arrows here. Arrows come here. So this is saying that starting at 0, from a differential equation standpoint at least, you can add a single individual n, and that individual will reproduce and gets to whatever the effective carrying capacity is at that delta.
So what we can do is we can draw the same bifurcation diagram here. Where we look at the population size as a function of delta. And now it's actually somewhat more interesting looking, because in the absence of this death rate, we have it start at k. So what's going to happen is it's going to come like this. And the reason we call it a full bifurcation is because these fixed points fold over on themselves.
Now this is a bifurcation where as a function is a control parameter, it's not that these fixed points exchange stability, but rather that the fixed points collide and annihilate. So in general, a bifurcation is where something qualitative happens to the fixed points. In this case, the number of fixed points did not change as a function of the control parameter. Whereas in this case, it does change. But there are only certain characteristic ways in which these six points are allowed to change.
So from this, we can draw our arrows. n equal to 0 is still a stable point down here. So we can draw. This is where colored chalk would be useful. We can draw our arrows. What do I do out here?
Now, the reason that we say that this population experiences a sudden transition, or a tipping point, is because you can imagine that this death rate being a slowly changing parameter. So you could imagine that it could be a slow acidification of the oceans, or a slow increase in the amount of fishing that takes place here. So then what can happen is that the size of the population, it's very healthy here. It's pretty happy, happy. Here it's maybe decreasing some, but you might not sound an alarm. But what can happen is that at some critical level of environmental conditions, some critical level of fishing, for example, you can get this catastrophic collapse of the population. Where over short periods of time, it can suddenly collapse.
Indeed, this sort of thing has been observed in a number of fisheries around the world. So if you go to, for example, the Monterey Bay Aquarium in Monterey, California, they have a very nice display explaining how that space ended up becoming and aquarium. Because it used to be a sardine fishery, and was a very productive fishery in the years leading up to World War II. But if you look at the number of fish that were caught as a function of time, it goes up, up, because there's fishing more and more, then all of the sudden, they just presumably fish too much and over a time span of a few years, the fishery collapsed, and there were just no more sardines to catch. That whole street there used to be canneries. But then it all went out of business. And those buildings were largely unused for many years. But then eventually, the aquarium went and bought some of them and refurbished them, and now it's a beautiful aquarium. So I encourage you to check it out.
But that being said, the collapse is probably still bad, even though it led to this nice outcome eventually. And what you can see is that not only are these tipping points or the sudden transitions maybe undesirable in the case of fishery, but it also would be very difficult to reverse. Because it's not that-- You couldn't imagined that the bifurcation diagram look rather different. It could have looked like this.
What kind of bifurcation is this? So this is still a transcritical. It doesn't have to be a line throughout, because in principle there was this unstable thing down here. This is again, and, as a function of some parameter, we'll say delta. And so you'd say, oh this is a rather sudden transition. In the sense that a modest change in delta will lead to a dramatic change in the size of the population.
But there's something that's very qualitatively different about these situations. Which is that, here you can imagine that if you improve the quality of the environment, and then you reintroduce fish, or maybe you don't even have to introduce fish, they can come from a different area. Then, just by improving the quality of the environment, you get recovery. Whereas here, that's very difficult to do, because the system is hysteretic, or has memory. That means that even if you improve the quality of the environment here, it may be very difficult to get back to your previous state.
People think that they've seen this in the context of these transitions in lake ecosystems, so-called eutrophication transition, where you get as a result of maybe runoff from agricultural uses. You can get the sun transitions of not just a single population, but of an entire ecosystem. So the lake can go from this thing where it's nice and clear and beautiful summer vacations spot, to this really green kind of algal take over. And it can be very difficult to get recovery. There's was a question? No?
And so the basic-- We'll say something about the early warning indicators. Well maybe I'll say it now. So based on the review that you read, there's this phenomenon of critical slowing down that tells us that in principle, you can anticipate the transitions about to take place. And the statement was that the dominant eigenvalue described in dynamics, a system went to 0 at one of these points.
Now, the systems they were talking about were presumably systems like this. This is a so-called zero eigenvalue bifurcation, were the eigenvalue goes to 0 at this point right here. So here's a question for you. Does the eigenvalue describe the dynamics of a system go to 0 at this point? At transcritical. In a transcritical bifurcation. Do you understand the question?
I'll let you think about it for 20 seconds, because this is maybe not totally obvious.
Do you need more time? I'm wondering. Maybe people are sufficiently lost or confused. They're not sure what-- Nod if you want more time. OK. Ready? Three, two, one.
I'd say it's a kind of a 50-50 thing. So go ahead and turn to you neighbor and try to figure out, using some combination of math and graphical analysis, whether the bifurcate-- Whether at this transcritical bifurcation the eigenvalue goes to 0.
[SIDE CONVERSATIONS]
PROFESSOR: It sounds like the discussion is maybe gone to completion. Let's go ahead and vote again. The question is, does the dominant eigenvalue of this system go to 0 at this point here? The trans critical bifurcation. Ready? Three, two, one. Alright. It doesn't seem like it's had much of an effect. I see that one person has been convinced of something. I don't know if he's actually convinced, of if he was just surrounded and bullied.
All right, so what are some different ways of thinking about this? Yes?
AUDIENCE: Well, one of my neighbors decided to actually just lineralize that equation at that point.
PROFESSOR: All right.
AUDIENCE: If you were completely into you intuition, this would be a way to check.
PROFESSOR: Right OK. And now in general, in life, I'm a big believer when you're confronted with some question, you should first think about it, what you should guess based on whatever intuition might be available to you. And then after you have made a guess based on intuition, then you go and you do the calculation. Which in this case, linearizing around. And where do linearize around? Around the fixed point.
I would say that you really want to linearize maybe not around-- You get the same answer if you lineralize around 0, but you really want to, I think conceptually, you want to linearize around this stable fixed point. Right? Because, in some ways, it's the stable fixed point that the system is sitting in, and you want to know how is it that you're-- How is it is you're going to react when you get perturbed away from that fixed point? And you do get the same answer.
The thing is that it's true that the eigenvalue describing the dynamics around this unstable fixed point also goes to zero. But that's because, I think the rules of mathematics somehow say that the fixed points are going to have to do something similar. So I think you do also find the eigenvalue here as it goes to 0, but conceptually that's not the eigenvalue that you actually want to know about. Do you see why I'm saying that?
Again, the eigenvalue here is going to 0. We can try to explore this a little bit, but I just want to kind of tie this together and say-- These local bifurcations that you have maybe heard about, which is that this fold bifurcation, the transcritical bifurcation, and also the Hopf bifurcation.
So the Hopf is one that looks where you have a stable fixed point that goes-- And in terms of an unstable line, you end up getting oscillations. So that's where you have something that that's going to look like-- but now that I'm drawing this, I'm a little bit-- Is there a difference between a Hopf and a pitchfork bifurcation?
AUDIENCE: It's whether you have oscillations or not. You can't really show that.
PROFESSOR: OK. So as drawn, it's either or both, or?
AUDIENCE: It's both.
PROFESSOR: OK well. I'll say that this thing also the eigenvalue goes to 0 here. Because I guess, in this-- You don't have to have oscillations in order-- And it depends on the higher dynamic systems. But again, this is another 0 eigenvalue bifurcation.
And so the statement is that in principle, you can use this fact that the eigenvalue goes to 0, this thing called critical slowing down, to measure some changes in the dynamics of the system before, in this case, you get collapse, or before, in this case, you get extinction. The issue maybe is that in this transition, there really is something big that happened at the bifurcation.
So you really want to have an early warning signal because there's a dramatic change, and it may be difficult to reverse. Whereas here, the population size is smoothly going to 0. And once you get down to your last two California condors, you know that you're in trouble. You don't need any sort of fancy early warning indicator based on fluctuations or return time, and so forth. Instead, just the number is maybe your best measure for the health of the population.
So I think that yeah, maybe I won't do the linearization, but I encourage you to do it. Because, that's what's going to be relevant. In particular, what you want to know is if there's some-- If you linearize around there some, just be clear, there's some n equilibrium. OK there's some equilibrium, and then you want to ask, well OK now you want to say if your n is equal to some n equilibrium, plus some epsilon. Then, if you plug it into the N dot function, you should get an equation that is something where this epsilon dot is going to be equal to something. What should it be equal to? Something times epsilon. And what something?
AUDIENCE: What we've been calling lambda?
PROFESSOR: What we've been calling labmda. So this is saying that if you go a little bit away from the equilibrium, so small epsilon here, that epsilon-- The solution to this is an exponential, it's going to get exponential decay, assuming that lambda is what? Negative. And that's the definition of-- is When we say this is a stable fixed point, this is unstable, that's equivalent to saying that the lambda here is negative and the lambda here is positive.
AUDIENCE: That's a very easy argument. They exchange stability. So you know that lambda has to be completely 0.
PROFESSOR: Yes, this is the point. So we know that lambda here is positive lambda-- I'm sorry, lambda here is negative, lambda here is positive. These fixed points at this point, they're going to exchange stability so that means they had to be equal. And where they exchange is when it crosses. So they both-- Well, I guess independently this went-- We should be able to draw this, although I'm a little bit worried that once I try to draw something I'm going to get confused. But this is a useful exercise.
In particular, if we plot as a function of delta. So here, we have the lambdas, and we have this delta. and a delta equal to r what's going to happen is that we're going to get the one guy's going to go like this. Something like that. Given, that the fixed points went from stable to unstable, unstable to stable, so they had to cross 0. So if they both cross 0 at that point, they're going to be equal n zero. You guys agree?
AUDIENCE: Do we know that the eigenvalues are going to change linearly with the death rate?
PROFESSOR: No. So in general, they don't. I'm trying to think if close to the bifurcation, whether I can say. The mathematicians I'm sure can say something. I'm not going to.
AUDIENCE: Does this also mean that whenever we're on a steady state, approaching bifurcation, we'll always see the critical slowing down? If it's a stable fixed point at a critical value.
PROFESSOR: I think that the statement is that in principle, critical slowing down occurs. But that does not mean that you'll necessarily be able to see it. Because it could be, there's just too much noise, or this, or that.
AUDIENCE: So something that I was thinking about related to that is-- So in this case, it seems like everything about the system, the fluctuations, or anything, should be getting very small, as you approach that.
PROFESSOR: In principle, the fluctuations are supposed to grow. Although this is assuming that the strength of the noise is constant. And in this case, the strength of the noise may not be constant, because if it's demographic noise, and the size of your population is going down. This is actually subtle then, I think.
And when we typically talk about these dynamics, we're assuming that there's an added noise source that is independent of the variable. But if it's demographic fluctuations you're talking about, that that won't be true.
I think that it's also useful to draw, to get some intuition around this, based on this idea of an effective potential. So these are one-dimensional systems, which means you can always write down an effective potential. And in this case, what it's going to look like is-- This is some u effective as a function of the population size. And up here, we have some stable state here that corresponds to something that looks like this. So it starts out maybe at k. What happens is, as the death rate increases, this thing kind of turns-- Or, maybe I didn't quite make it.
So what you see is that as the death rate here-- So, as we go down the death rate is going up. So that what happens is the effective potential, describing the dynamics of the population near the equilibrium is broadening out. So you can think about the dynamics as being equivalent to an overdamped particle in effective potential.
So for example, for those of you that have studied single molecule biophysics kinds of things, this could be thought of as a [? poly-centered ?] bead that's trapped in a the laser trap. And as you turn down the power of your laser, then the spring constant describing the dynamics of that B in the trap, it gets weaker and weaker. So the potential broadens, and that means that for fixed injection noise-- fixed temperature-- you get these effects, where the fluctuations will increase in magnitude, and you get an increase in this autocorrelation time. Because for example, the bead in the trap, the autocorrelation time is indeed just equal to the relaxation time of the bead in the trap.
And the bifurcation occurs right at this point here, where you see that this local potential is, or the local minimum, is disappearing. That's when this stable fixed point is gone. And then you just kind of fall off here. And that's what leads to this collapse, which I have covered up. That leads to the bifurcation.
And you should, for example, be able to draw an effective potential here as well. But I'll let you play with it.
But you can see the location of this unstable fixed point shifting as well. I'm not sure how good my drawing is. Because in principal, this unstable fixed point should have to come together. I'm not sure if that's very clear in my drawing.
Just to summarize this discussion, I think there are a few ways that you can think of these early warning indicators. And there's a diagram that I like to make, that I think makes things more clear, for me, at least. Which is that if you look at this population as a function of time, it goes. And if there's an environment quality as a function of time. If the environment has a perturbation, then the population will shrink, and then you'll get recovery. And this thing here tells you about the time for recovery. And this basic phenomenon critical slowing down tells you that as the tipping point approaches, as you approach the bifurcation, that time to recover from this perturbation is going to grow.
Of course, the other thing that you can say is that even in the absence of a defied perturbation, even if the environment is constant over time, there could just a natural noise in the system that will fluctuate, just like temperature, for the bead in the trap. And principle, then you can measure the size of the fluctuations, the variance, as well as the autocorrelation time tau, which is, for civil systems, equal to t. And this also grows as you approach the bifurcation
AUDIENCE: Is it [? clear ?] that-- Because the perturbation [INAUDIBLE] that you're talking about is I think n [INAUDIBLE] perturbed n into epsilon, and then it relaxes the steady state. Is it [INAUDIBLE] to perturb the environment?
PROFESSOR: Yeah, right. So that's a good question. What I've been talking about is a situation where you really literally pull it away, and then you let go. And that's equivalent for in the bead in the trap, that you pull the bead away, and then you let go, and you watch it come back.
The situation in the case of the environment is that, if it's a kind of a sudden shift-- It could be anything, it could be a brief change in-- Well it could just be delta. So it could be that over some period of time, the death rate increases, or some period of time, the growth rate decreases. Or it could be that for-- The perturbations don't have to be bad, they can also be good, and you actually get the same. The principal for small perturbations to go, it's the same thing anyway.
So these are early warning indicators of an impending transition that are based on temporal indicators. And one of the things that we've been excited about in my group is actually just trying to measure these things in a well controlled laboratory population. Since in our case, we're using yeast that are engaging in what you might call a group hunting behavior, where they secrete an enzyme that breaks down sugar, and that kind of leads to this cooperative growth. And at least in that case, we can experimentally measure an increase in all of these things as we approach this bifurcation.
The other thing you might think about is what happens for spatially extended populations? We'll talk more about spatial populations on Thursday, but just while we're here, it's useful to think about it. So instead of thinking about just n as a function of time, now you want to think about density of population. So one thing that people talked about is that-- Sorry, this is not a function of time, it's now a function of position x. Density is a function of position. Now, environment is a function of position. If you have a uniform environment over position or space, then in principle you can look at density fluctuations. And this is something that people have talked about.
One of things that we have argued is that there should be a spatial analog to this recovery time. So that corresponds to a situation where you have environment as a function of position. Like for example, we have a sharp boundary, or just a region of poor quality. And in that case, you can look at the density as a function of position, and you get some linked scale here.
So the statement here is that, just because you're in a region of high quality doesn't mean that you're at your equilibrium density, because if you're close to a poor region, so if you're close to hunting grounds, then you'll get local depletion of the population. You have to get some distance away from a bad region before you get your equilibrium. That distance or recovery length tells you about the quality of the environment. And it's the quality of the environment at this region, the good region. Where you are.
At least in the laboratory, this is something that's actually much easier to measure than other things. Because, this is a deterministic phenomenon. You don't have to measure fluctuations over time with a high quality time series. You don't have to wait for a perturbation in time, like a drought, and look at recovery. Instead, you just take advantage of natural variations in quality of the environment over space, position, and then you measure profiles.
We have a collaboration with a professor at the University of Pisa who does field ecology experiments with these algal mats on intertidal communities, on islands in the Mediterranean. And we have a [? pronates ?] that we can measure this in those island communities as well.
Are there any questions about this stuff before we switch gears? Yes?
AUDIENCE: In that example, is it-- How much control can you have over actually [? saying ?] the quality to the environment?
PROFESSOR: So in that manipulation, what they did was they basically go in and they-- So there these, they're like miniature forest somehow. So they're little-- They have alternative stable states. What they actually do, they go and they like chip away at the rock to remove the things. And they do it over some range. So they experimentally basically make it a challenging environment. And apparently, they have permission to do this. So don't try that at home without asking the proper authorities.
Any other questions about this?
So I think that the nice thing about studying all these dynamics just for a single population, is that it really clarifies the essential ingredients, in order to get things like sudden transitions. Of course, in natural populations, we have many, many species and tracking in lots of complicated ways. But we would like to understand those things, but I think since it's so complicated, you kind of like, oh anything can happen, and you get a little bit discouraged from thinking deeply about it. Because, you just think it's going to be too complicated to try to understand. I very much like the idea of trying to really hone your intuition on the simplest possible situation like this, and then bringing that intuition to more complicated or complex situations. Yeah?
AUDIENCE: Can you partially [? adjust ?] But what I want to ask you is whether any of this can be applied to human society?
PROFESSOR: Oh, yeah. So whether this could be applied to human society. It's a good question. People certainly try to. I think you'll always have to decide what we mean by apply. I would say that this basic idea that there could be these feedback loops that can lead to sudden transitions in complex systems. I think this is a very robust phenomenon, in the sense that I think if you have strong enough interactions, then I think you kind of expect it to be true.
Another question is whether you could quantitatively predict when that's going to happen. There was a recent article written in science or nature about potential tipping points in human society on a global scale. Things in terms of productivity of crops. And so they have a question mark about, they say 2050, question mark, collapse maybe. I say it's very important to be thinking about these things in. But the question is what to do, whether given the uncertainties and your knowledge of where these tipping points might occur, it's always hard to know whether you could make a strong argument saying, oh we have to stop fishing here because it's going to collapse.
There's always uncertainty in your decision making. But certainly in the context of climate regime shifts, people are worried about these sorts of feedback loops and the North Atlantic Oscillation and so forth. And I'm not at all an expert for that, so I don't know whether we should be worried. But it's at least good to remember that systems can respond in dramatic ways to small changes. And then, you have to decide what to do with that knowledge, and I think that's more of a judgement call.
AUDIENCE: In the time example, you had death, it was a death rate. Is that in a [INAUDIBLE] space?
PROFESSOR: OK. So I want to be a little bit maybe more clear. The claim is that-- Like the death, the delta there that we're thinking about, that didn't have to be the perturbation. It could be, but it didn't have to be. When we're plotting the bifurcation diagram, we're assuming implicitly somehow that there's a separation of time scales, such that this delta might be changing very slowly, and then other things are changing more rapidly. So this bifurcation diagram, it's really that you're tracking it.
So in the context of this n as a function of delta, this thing goes like this. And the idea is that oh, slowly you're getting more and more agricultural runoff, or the temperature is increasing. Doesn't have to be human induced, by the way, it could be something else. So the idea is that the population is slowly changing like this. And then eventually maybe you get this collapse.
Now, a perturbation could be something that could just be a drought, or something that is independent of this delta. But the statement is that, over here-- Now I'm going to be mixing things. At low delta, the claim is that you should get a dip and then rapid recovery. As the delta gets bigger, you get maybe even a larger-- The same perturbation could [? principally ?] do a larger dip, and it takes longer to get recovery.
This actually brings up another point, which is that these could all be the same perturbations. So this is a case where delta is increasing as we go down. So they could be the same perturbation here. Here you survive, here you survive. But then that same perturbation that was survived here could be the same magnitude drought, just as delta increases you expect this-- This may be able to push the system past the unstable fixed point, because of a loss of resilience.
This is something that we've seen in a variety of different contexts. I think it's a very general phenomenon. Just because the separation here is some measure of the resilience of the population, or the ability of the population to withstand perturbations. We can see that the resilience shrinks as we get close to this bifurcation. Did that answer your question? Sort of? Not really.
AUDIENCE: No, but it answered another question.
PROFESSOR: It answered another question? Oh good. I'm glad that I answered something. But what was your-- Because you were saying is it the death rate that is causing this perturbation?
AUDIENCE: Well, no. I was wondering for [? per ?] space.
PROFESSOR: OK. Oh yes, I forgot. Yes, I do remember that you did ask that. So the idea here is that this could be a region that we fish, and this could be region that we don't fish, for example. Or it could be that, here there's just not as much food for the organism as there are here. So just the idea is that you would need kind of a sudden sharp boundary between regions of different quality. And that's kind of the situation where you would be able to measure this recovery length.
And the basic reason for that, is that if the environmental quality is something that changes very slowly, then the population density will just track that. So then you can't use that to measure this recovery length.
Of course, the same statement is true here. That if you have environmental perturbations that are changing slowly over time, then that also can complicate this picture.
Any other questions about that before we move towards multispecies ecosystems? We're going to start with two species.
So what I'm going to do is I want to spend the rest of the class talking about Lotka-Volterra populations. And we're going to move the rock, paper, scissors discussion to Thursday, because it's a nice spatial example anyways.
So let's think about Lotka-Volterra. Now, there's a lot. Lotka-Volterra competition model.
So the assumption is that we're going to make here is that we have these two species that going to be interacting, but they're going to interact in kind of the simplest way that you might imagine. Which is that, if we plot the derivative of population size n1 as a function of time, we get something that looks like this.
Now the first thing that you want to do when you see something like this is just to make sense the basic equation. In particular, in the absence of the other species, what happens to these populations?
We're going to assume here maybe first is that r1 and r2 are both greater than 0. It's in the absence of the other species. Do these populations survive or not? Ready? Three, we're going do it A, yes, B, no, our typical. All right, absence of a species. ready? Three, two, one, survival?
Yeah, they survive. For sure, because I told you that these guys are greater than 0, that means-- We can think about n1. In the absence n2, what is this equation? Logistic.
Now, if we wanted to think about these as describing competitive interactions, what does that tell us about the sign of the betas? Are the betas greater than 0, or are they less than 0? I'll think about it for 10 seconds. All right. Do you need more time? Ready? Three, two, one. Competitive means that the betas are greater than 0.
And what is the assumption somehow that's going into this? And we should remember that this is a minus everything up here.
AUDIENCE: I guess that somehow these two things are-- there's a fixed amount of some resource, that both of these things need.
PROFESSOR: OK, so there could be some fixed resource.
AUDIENCE: It's basically like an effective carrying capacity.
PROFESSOR: That's right, so somehow it's modulating the effect of carrying capacity. And how is it that you would describe these betas?
AUDIENCE: Sort of seems like you expect that the beta should be probably less than one. [INAUDIBLE] I guess it doesn't have to be less than one. Compared to how well n1-- and individual of n1 takes up some of this. This is how much [INAUDIBLE]
PROFESSOR: That's right. I think that's right. Now I think we-- There's a question about how explicitly to be thinking about these resources. Because it could be, it doesn't have to be one resource. And this is of course, a very phenomenological model. It's lumping all the interact-- All of the ways in which a species interacts in just a single parameter beta. But it's somehow, the betas are telling us something about how much does a member of the other species inhibit my growth, as compared to a member of my own species?
Because we have this n1 here, and just this species one will naturally lead to a carrying capacity k1, you only have species one. Now, the betas are telling you something about how much overlap they have in terms of maybe [? niche ?] or so. Now, if the betas are small, it means that they're not competing with each other very much. The betas could be larger than one, and what that's saying is that a member of this other species is inhibiting my growth more than a member of my own species.
So the standard way to analyze these equations is to look at these isoclines. So basically, if you say, OK well n1 dot is equal to zero, what does that mean? Well, we can just see that that's equivalent to saying that it's n1 plus beta1,2 n2 is equal to k1. So this is giving us a relationship between n1 and n2.
What do these look like on a plane, n1 versus n2 drawings? So the parabola, it's a line. And indeed, we can think about what happens when each of these is equal to one thing or another. So if n1-- If n2 is equal to 0, this thing is going across at k1. And that makes sense, because we already decided that in the absence of n2, this thing is just following logistic growth, where the species one will grow and go to k1. And that's a stable fixed point that n1 dot has to be equal to zero. It's a fixed point, so n1 dot has to be equal to 0. And it's going to cross at this other point here where n2 is going to be some k1 over beta 1, 2. And then we end up with a line.
Now if we go, and we ask what n2 dot is equal to, and this is equal to 0, we're going to end up with something that looks very similar. But now it's going to be n2 plus beta 2, 1, n1 is equal to k2. This is also going to be a line.
Given what I have said, how many different qualitative outcomes can you get in this model? Where we just assume-- Where we have beta-- Overall I think we're thinking about competitive interactions. How many cases do we need to consider? Alright, it's going to be a, 0.
Do you guys understand what I mean by cases? No? I mean, how many different kind of qualitative outcomes can there be in terms of species one or species two winning, or can you get coexistence? Or how many different kind of qualitative outcomes can there be? And if you're confused, you can not vote, or give me an unhappy face. OK, ready? Three, two, one. So
We have many Es. And can somebody explain why it that this is the case, without invoking that you've already studied this and you know the answer?
AUDIENCE: If you add another line, completely on top, or under, or it can cross too.
PROFESSOR: That's right, that's right. So the next thing I was about to do is draw another line. And the reason I stopped is because there are actually four different ways in which this other line can be drawn. And basically, you can have another line that does one. You can have two. You can have three. And you can have four.
OK? These two cases-- So this is we'll say one. I don't know what order i did it. Two, three, and four. Two and three, in both cases we have the crossing of the lines. So maybe does that mean they're the same outcome, the same qualitative outcome? No. And what will end up happening here is that we're going to get cases of--
So you basically can get that species one dominates independent of starting condition, assuming that both are present at the beginning. Species two could dominate. They could coexist, or you get bistability. History dependent, mutual exclusion. I just want to draw. So this one here was the-- We had a dashed line for n1 dot and we had the solid line for this. Yes?
AUDIENCE: Where is [? the breaking ?] [INAUDIBLE]
PROFESSOR: So I guess if you swap the labels, or so, you're saying? I guess in the case that if one of them is just above the other one, then you can distinguish them. So if you have a dashed line here, you have a solid line here, that means that they--
AUDIENCE: How do I distinguish case 2 and case 3? [INAUDIBLE]
PROFESSOR: Maybe we should try to figure out which one's which, and then we can figure it out from there. Can somebody remind us, what are these lines again? Why are we drawing them, or what do they mean? So they're not actually quite a fixed point. It's a no incline. What's the difference between these things? If we have a fixed point here, what we're saying is that both n1 dot and n2 dot are equal to 0. Fixed point means that if you start right there, you'll stay right there. We're not yet say anything about stability. And this is going to be very relevant for Sam's question.
AUDIENCE: [INAUDIBLE]
PROFESSOR: The axes are definitely labeled. I think I may agree, but I'm a little worried that I'm-- If you're happy I'm happy. OK let's-- A fixed point of this pair of equations will be when both of the derivatives are equal 0. And that's not the line that we've drawn. The lines that we've drawn are just when one or the other one is equal to 0.
So what does that mean about in case four, is it possible well to have coexistence? No. Because there's no fixed one in the middle. The only case it's possible to get coexistence is either line two or line three. But that tells us there's a fixed point, it doesn't tell us about the stability of the fixed point, though. What we'll find is that in one case, it's a stable fixed point, you get coexistence. In the other case, it's an unstable fixed point, and you get bistability.
What we're going to do now is we're going to ask and try to figure out in which case, how do we label these things? So what we'll do is ask, first of all in line one, we want to figure out, is that a situation where we know it's not going to be coexistence are bistability, but is it going to a situation where a species one wins, or when species two wins? But, if you want, you can vote for one of the others. I don't want to constrain your choices. So it's going to be a is one wins, B, two wins, not three wins. This is something else. I'm going to give you a minute to think about what might be going on here. So the question is put in situation one.
Oh no, I did that wrong. In this. For the solid line, this is what we're asking for n1 is equal to 0. This is just equal to k2.
AUDIENCE: [INAUDIBLE]
PROFESSOR: What's that?
AUDIENCE: There's other [? null ?] lines.
PROFESSOR: You want more [? null lines? ?] I feel like there are lots of lines up there already.
AUDIENCE: Where n equals 0 [? null ?] lines. The axes are actual [? null ?] lines.
PROFESSOR: Yes, that's right. Yeah, that's true.
AUDIENCE: That helps-- I'm confused between labels.
PROFESSOR: Oh, I see. OK I'm hesitant to draw anything more up on the board. But it is true that the axes are also [? null ?] lines. Because we have n's up there. I'll give you another 20 seconds to try to figure out this case one. Which of the species is going to win?
And let's go ahead and vote, and see where we are. Ready? Three, two, one. So there's a slight majority that are agreeing that in this case, it's going to be that one is going to win. And I think that it's a little bit hard to figure it out completely, but what I will say is that in the case where we're down here, what this means is that k1 divided nu beta 1,2 is larger than k2.
Now the question says, what happens in the limit of-- If species two does not hurt species one? Well that's when beta 1,2 goes to 0. And that's the situation where this goes up. So this is saying that if the beta 1,2-- And then we can figure out where this line is as well, because this thing is K2 divided by beta 2,1.
So if species to doesn't hurt to see one, but species one really hurts species two, that's going to be the limit where species one is going to win.
We are out of time. Maybe what we'll do is start class on Thursday by-- I'll start with this on the board, and then we'll complete these four possibilities, to try to get some intuition about it. And also draw these options.