Flash and JavaScript are required for this feature.
Download the video from iTunes U or the Internet Archive.
Instructor: Dennis Freeman
Description: Today's lecture solidifies the connections between continuous- and discrete-time Fourier series and transforms, converting between the time and frequency domains with familiar tools such as convolution, periodic extension, and sampling.
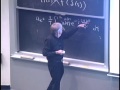
Lecture 19: Relations Among...
The following content is provided under a Creative Commons license. Your support will help MIT OpenCourseWare continue to offer high quality educational resources for free. To make a donation or view additional materials from hundreds of MIT courses, visit MIT OpenCourseWare at ocw.mit.edu.
DENNIS FREEMAN: Hello. So there's almost certainly no need to show this, but the good news is this is the last time I'll ever show a slide like this. It'll never happen again. At least not to this crowd.
So you can all rest assured this is the last time you'll see something like this. Any questions on this, everybody's happy? Those are two separate questions, I guess. Any questions on this? OK, good.
So today I want to finish up talking about Fourier transforms by comparing a lot of stuff we've already done. So there's nothing new today. This is intended, if anything, to be a bit of a review in light of things that might happen tomorrow. And also just to make things look simpler.
Now, the best way to make things look simpler is to start with something that looks incredibly complicated. OK, so this is my thing that looks very complicated. This is simply a summary of the transforms that we've talked about today. We've talked about four of them.
And the point of the slide is first, it looks a little complicated. But much more importantly, by the end of the hour, it's supposed to look very simple. Yes?
AUDIENCE: Could we increase the volume?
DENNIS FREEMAN: Could we increase the volume a bit? Well, I know that I can shout more. And if they notice me, they'll turn the volume up, too. So I will try to shout more. So if I don't, please tell me to do so.
So the idea, then, is to try to look for some structure. Not only within one of the transforms, but also across all of the transforms. And to start this off, let's just think about it at the most basic level.
What were the kinds of signals that we thought about? The kinds of signals that we thought about when we did DT Fourier series, DT Fourier transforms, CT Fourier series, and CT Fourier transforms? The kinds of signals look different, right?
So in the top ones, we had discrete time. In the bottom ones, we have continuous time. In the left we have things that are periodic. In the right we had things that were not periodic. Four different kinds of signals. Not surprising there would be four different kinds of transforms.
An interesting thing happens if you look at the Fourier domain. I haven't switched around the order. It was DT Fourier, DT Fourier transform, CT series, CT transform. It's still that.
I've drawn a sort of iconic view of how we would draw a picture of the Fourier transform of interest. Where is discrete? So discrete in the previous picture-- which was a picture of time-- NNTT. Where was discrete in this picture? Up.
Where is discrete in this picture? Left, that's weird. Where is periodic in this picture? Left. Where is periodic in this picture? Is there a periodic in this picture? Top. Why do you say top? Ah, unit circle! Unit circle is periodic.
So there's discreteness and periodicity in both pictures, but they're not at the same place. Well, that's kind of interesting, right? Somehow, discrete and periodic map to things like discrete and periodic, but they don't map directly. That's kind of interesting.
So part of the goal today is to try to get to the bottom of that. To understand why that's true. You already know a lot about how different transformations are related. So let's think of a simple example of the relation between Fourier series and Fourier transforms.
Imagine that we have one of those signals that was periodic in time, continuous in time. Then there'd be a Fourier series-- represented this way. So I'd end up with a Fourier series that has a bunch of coefficients, a, k.
Can somebody tell me, what would the Fourier transform look like? Fourier transform-- are you allowed to take the Fourier transform of a periodic thing? Yeah?
AUDIENCE: Could we take the same thing with impulses?
DENNIS FREEMAN: Take the same thing with impulses. OK, I like lots of those words. Could you put them together in slightly a different order? Where should I put the impulses? Yeah?
AUDIENCE: At the k omega not.
DENNIS FREEMAN: At the k omega not. How do you get that? That's exactly right. How'd you get that?
AUDIENCE: Because the [INAUDIBLE].
DENNIS FREEMAN: So there's some kind of a relationship between the k's, in the ak's, and frequency that ought to be represented as an impulse, is what you're saying? One way you can see that is to take the Fourier transform of this expression.
What would you get if you took the Fourier transform of x of t, x of j omega? That's pretty easy. What would you get if you took the Fourier transform of a sum? What do you always get when you take the Fourier transfer of a sum? The sum of the Fourier transforms, right? It's within your operator.
That's one of the most powerful reasons we like it. So sum of the functions, take the transform of each one. Add them back up. How do you take the Fourier transform of e to the j omega not t?
What's the Fourier transform? e to the j omega not t transforms to what? Fourier transform-- impulse. So how do I know that? So impulse-- what else, anything? Am I done?
Where should I put the impulse? What should I put-- how should I indicate where I put it? So I need to say omega minus omega not, or something like that, right?
So how do I know that? And am I done? Yeah?
AUDIENCE: Multiply by 2 pi.
DENNIS FREEMAN: Multiply by 2 pi. And a good way to do that is just to memorize an absurd number of equations, right? Let's think about how you would remember that.
So if you wanted to take the Fourier transform of anything, right? You would say, h of j omega is some integral of anything e to the minus j omega t dt, right?
What happens if I plug x of t equals e to the j omega not t into this expression? Can you close the integral? I mean, that's the way you would do it, right? I want to take the Fourier transform of e to the j omega not t. You stick it in the formula, and it should come out, right?
So how many of you can integrate this function? No one, good. That's good, because I can't either. What's hard about integrating that function?
That was a much easier question, right? Can you integrate was a hard question. Well, maybe not. It's binary. What's difficult about integrating that function of time? What if I try to integrate e to the j omega not t e to the minus j omega t dt? What's difficult about integrating that function?
What do we do when we see these sorts of things-- e to the j omega not t? How do you think about e to the-- complex exponential. You can name out things like Euler's equation, right? So e to the j omega not t-- you might want to say, well, that's the same thing as cosine omega not t plus j sine omega not t.
If I took just the first term-- cosine omega not t, does it have a Laplace transform? Doesn't have a Laplace transform, hmm. How about a Fourier transform? Why does it have a Fourier transform and not a Laplace transform?
So Laplace transforms-- there's only a Laplace transform if there's some kind of a multiplicative kernel-- e to the minus st-- that has some values that make the integral converge. There's no value of s for which e to the j omega not t will converge.
The amplitude of e to the j omega not t-- the real part has an amplitude that fluctuates like this and never decays. Goes on forever and ever in both directions. There's no exponential function that you can multiply times that function to make it converge. There is no Laplace transform. Is there a Fourier transform? Yeah.
What's different? What makes us be able to do a Fourier transform and not a Laplace transform? Delta functions. We never write a Laplace transform with a delta function, ever. If you write on your exam h of s is some function of delta of s, that's definitely wrong, right? We just never do that.
We're much more liberal about using delta functions in Fourier transforms. That's one of the reasons they're powerful. And one of the ways you can think about that is that delta functions are easy to integrate. That's not quite enough.
The other thing that's important to realize is that we've got this integral here-- it's either going to be zero or infinity. There's going to be certain values of omega that when we multiply this together, we're going to get something that goes on and on forever and ever and never decays.
That's going to give us infinity. And there's going to be other values that the long-term integral's going to go to zero. And that information is summarized by the impulses, right?
So if we choose omega not-- if we choose omega to be omega not-- then when we do the integral, which comes out a function of omega, we're going to get something that goes to infinity. OK?
The easy way to think about that is to do the inverse Fourier transform, right? The inverse Fourier transform is x of t is 1 over 2 pi in the integral x of j omega e to the j omega t d omega.
Now, if we put the delta function in this, it's easy. Because the delta function over here-- if we say that-- it's x over here and it's h over there. But who cares? That should have been x.
If we put over there that the x of j omega is 2 pi delta omega minus omega not, then we can see that the function of the delta is just to sift out a particular value of e to the j omega t, the one at omega not. And the 2 pi's obvious, too. The 2 pi came from the 1 over 2 pi, right? We had to have a 2 pi out front so that the 1 over 2 pi killed it.
OK, anyway-- so the idea, then, is that simply by taking the Fourier transform of this, we can get an expression for the Fourier transform. And that tells us something about the great utility of using impulses, right?
Because we use impulses in the Fourier transform, we can represent a lot more signals than we could have represented in the Laplace transform. That's one of the utilities of a Fourier. And there's a big relationship between the Fourier series and the Fourier transform.
So in particular, if we have a signal that's periodic in time, it'll have both a series and a transform. The transform is a bunch of delta functions weighted by the Fourier series coefficients and 2 pi.
And what that says is, had we been willing to put in impulses to begin with, we would never have had to define the series. We did the series just because it was easy. Convergence issues were more clear.
But if we had been willing to think about delta functions in the transforms-- which would have been an extremely foreign concept coming straight from Laplace, where you would never see that. Had we been willing to accept delta functions in the transforms from the outset, we never would have needed series.
So the relationship between series and transforms has to do with the fact that the series represents periodic signals. Periodic signals go on forever. There's never a Laplace transform, and that means that-- there's never a Laplace transform. That means the Fourier transform has a bunch of delta functions in it, OK?
OK, so what I want to do, then, is think through other relationships that are on this table. And in particular, I want to think about them by thinking about the underlying structure of the signals.
So think about having DTFS, DTFT, CTFS, and CTFT. So what I want to do is think about the relationships among those four transforms by thinking about how they differ in time, right? So the top guys are discrete in time. The bottom guys are continuous in time. The left guys are periodic in time. The right ones are aperiodic in time.
So what I want to do to think about how they relate to each other, is think about a base case. Think about a signal that's down here-- aperiodic CT. And think about what you would have to do to the signal, and therefore what would you have to do to the transform.
If you wanted to move this way-- I'll do that one first. If you move up-- I'll do that one second. Or if you move that way-- I'll do that one third. So really, the rest of the hour is just doing three examples. Well, four examples.
What's the transform of a base case? Then what would happen as I move through this table? OK, everybody's with the game plan?
So here's my base case. I want to think about the Fourier transform of a signal, x of t, that's a triangle. We could calculate the Fourier transform just from the definition. That's trivial, right?
Good way, bad way. Good way. Smile. Better. OK, bad way-- I can think of a better way to do it. What's a better way to do it? Yeah?
AUDIENCE: [INAUDIBLE].
DENNIS FREEMAN: So one way is to think about turning straight lines into constants via derivatives and integrals and stuff like that. That's a very good way. Can somebody think of a different way? That'll work, it'll be fine. Yes?
AUDIENCE: Is that the convolution of two square pulses?
DENNIS FREEMAN: Convolution of two square pulses, that's wonderful. So a different way we can do it is to convolve a square pulse with a square pulse. That's particularly good for this example, because a square pulse is one of our canonical forms.
So if we think about what's the Fourier transform of a square pulse? We all know that it's something that has the form sine omega over omega. So all we really need to do is fill in the constants.
Filling in the constants is easy, because we know the moment theorems. The moment theorem says that whatever the area is under this curve, it has to be the height of that curve at 0. Whatever is the area under this curve, it has to be the height of that one. Except that you have to divide by 2 pi, because the annoying 2 pi factor.
The annoying 2 pi factor is something that's in the inverse transform and not in the Fourier transform. That makes it asymmetric. So in order to choose the constants-- the area under this is 1, so that height must be 1.
The area under this, by magic, turns into the area of that triangle, which is 2 pi. Divide by 2 pi is 1. So that means that this frequency must be 2 pi in order to make that constant be 1. So we're done.
So we know, then, that the transform of this signal, which is the convolution of this signal with itself, is just the product of this function with itself. Convolution in time maps to multiplication in frequency. OK?
So that's the base case. Now what I want to think about is how I would march through the table. That's my case down here. So what I want to do first is think about, how would I convert that this way? And what's the implication for that conversion in terms of time, and in terms of frequency?
So one way to think about the transformation from a transform to a series-- you can only series with things that are periodic. The transformation that we talked about in class was periodic extension.
So you think about taking the base case, which was a single triangle wave, and turning it into a sequence of triangle waves. There's lots of ways to think about that. But the way I want to motivate is to think about it-- well, no, backing up.
So if we think about the triangle wave and transformation and make it into a periodic triangle wave, we could just substitute this into the definition of transform. That's ugly, why? For the same reason that one was ugly. What's ugly about this? The signal goes on and on and on and on and on.
There will be certain frequencies where it'll blow up. Those will be infinity. There will be other frequencies where it is 0. There could be frequencies where it's minus infinity, and those are the only three options.
Since it goes on and on and on and on, if it's anything different from 0, it has to be either infinity or minus infinity. It's going to be a train of impulses for the same reason that thing was some kind of a train of impulses. So we want to have a good way of thinking about it.
I told you one way to think about this-- by taking the inverse transform. This is a little harder to guess the transforms, so you can verify it with the inverse. But you can form this by thinking about convolving the base waveform with an impulse train.
So what if I had an impulse train? So I'm-- oh, it's on the next slide. What if I had an impulse train like so? If I think about having an impulse train, if I had the base waveform and an appropriate impulse train, how could I manufacture the signal of interest, which is this periodic extension?
So if I have these two signals, what would I do to get that signal? Convolve. So if I knew the transform of this, which I do because this is the base case which we just did. If I knew the transform of the base case, and if I knew the transform of the impulse train-- impulse train is something that goes to function of time.
0-- let's say that they're separated by t. Let's say each one has a height of 1. What's the Fourier transform of an impulse train? I can see you're all on the edge of your seats. How do you find the Fourier transform of an impulse train? OK, ask your neighbor.
So how do you find the Fourier transform of an impulse train? Look it up in the tables! Boo, hiss. How do you find the Fourier transform of an impulse train?
Is there anything easy about an impulse train? What a horrendous thing, right? It has an infinite number of impulses, yuck. Each impulse is horribly misbehaved. How would I find the Fourier transform of a train of impulses? Yes?
AUDIENCE: So if you actually just plug that in to the formula, then you get different exponents and like, [INAUDIBLE], and then, since there's negative infinity to infinity, they add up to the [INAUDIBLE].
DENNIS FREEMAN: So you're saying that somehow I should stick it into-- so I'm thinking about finding the Fourier transform by going back to the direct formula. So if I put an infinite train of impulses here, what would I do? So I'd have a sum of delta of t minus, say, n times t sum over n e to the minus j omega t dt.
That's a good step. So now, what I might do is interchange the order, because that's what everybody does, right? And it's a little dicey doing that, right? You're always safe to do that if the integrand is absolutely summable. That's not precisely absolutely summable.
Everybody knows what absolutely summable means? So it means if you took the absolute value and you summed it over all of time, you would get something less than infinity. That's not quite the truth here, right?
So some of the sums in Fourier series and Fourier transforms can be hard, OK? But throwing caution to the wind, we would probably get something like that.
And then we could say, well, that just samples it. We could sample this thing at t equals nt. So that would be e to the minus j omega nt. Then I end up summing a large number of complex exponentials-- large being infinite. It's kind of ugly. Yes?
AUDIENCE: Could you now [INAUDIBLE].
So I could split this into two sums-- n less than 0, and a similar sum-- n bigger than 0. And then throw in the 0 term, just for good measure.
AUDIENCE: And then we can see you have a bunch of sines-- actually, in this case, you have a bunch of cosine terms. So you know that-- by having the cosine terms, [INAUDIBLE].
DENNIS FREEMAN: So you just said something that seemed to take a left turn, there. What was the thing about series?
AUDIENCE: So if you take your Fourier series of a sum of cosines, it's just the coefficient of the same sign?
DENNIS FREEMAN: So, that sounds good. Could we use that idea of taking a Fourier series a little earlier in the proof? How would I take the Fourier transform of that? How would I take the Fourier series of that? Yeah?
AUDIENCE: All 1's. All 1's.
DENNIS FREEMAN: All the 1's, yes, yes. This is periodic. It has a series. Trick? No, no, no-- clever observation, not a trick. So it's a periodic waveform. It has a series.
OK, why is that good? Periodic waveform series, I only need to look at one period. I reduce an infinite number of impulses to one. That's a good move.
So all I need to do is-- to find the series, all I need to do is think about the Fourier series sum. Which is almost the same thing, except that now I've got something that has k's in it, right? Because a series only has harmonic frequencies.
So what I can do is, instead of thinking about a Fourier series-- so now I can say, instead of finding the Fourier transform, let's find the Fourier series. That's find a sub k, which would be 1 over t to the integral on t of x of t e to the minus j 2 pi kt by t on t, OK?
So now I only need to find the series. And by integrating over t-- OK, well I'll do the easy one, right? Never do something that's hard. I can choose any interval of t. Let me take that one.
So the only thing that persists, then-- so if I do minus-- whatever, t by 2 to t by 2, then this becomes delta of t. And delta of t sifts out the 0 value of this guy. But e to 0 is 1. So this is 1 over t for all k.
We knew that, right? The Fourier series for a unit impulse is 1 everywhere. All the harmonics have the same size-- same size, same magnitude, same phase everywhere.
Then, since we know the series, we can trivially convert it into a Fourier transform, right? Using the deep insight from a few slides ago, right? So how do you take a series and turn it into a transform? Substitute for every k.
AUDIENCE: [INAUDIBLE].
DENNIS FREEMAN: Put an impulse in place of every k at the frequency-- omega-- that corresponds to that k, OK? The k's-- 0, 1, 2, 3, are the 0, 1, 2, 3 harmonics. The harmonics are harmonics of the fundamental. The fundamental is 2 pi over t.
So you put one impulse at every multiple of 2 pi over capital T. And you multiply them all by 2 pi. So here's your answer. You start with this thing you recognize as a periodic waveform. You turn it into a series. Then you convert every one of the harmonics into a continuous frequency representation so that the spacing is the base period 2 pi over cap T.
The height of these guys was 1 over T, because of the annoying 1 over T factor in front of the Fourier series formula. And you get an extra 2 pi, because the annoying 1 over 2 pi in the inverse Fourier formula, OK?
So what we see, then, is that the Fourier transform of an impulse train is an impulse train. And so we can use that, then, to find the Fourier transform of this mass, remember? Oh, I've got-- oh, here it is.
I was taking my base waveform, which was a triangle, trying to figure out what would happen if I did an infinite extension of that-- an infinite periodic extension. I think about the extension as being convolving the base waveform. Cover that up.
If I think about convolving the base wave, form-- oh, no, it's gone. If I think about convolving the base waveform with periodic train of impulses, that means that-- so the time waveform is the convolution of two things. That means that the Fourier transform is the multiplying of two things.
So I write down the transform of the base waveform-- thing that we did a long time ago. I write down the Fourier transform of the impulse train. The period of the impulse train is the same as the period of the periodic extension, which is capital T.
It was 4. So that means the impulses are separated by 2 pi over 4 pi over 2. And the height of the impulse is always equal to their separation. So they're also pi over 2. So then all I do is multiply this times this, and that gives me the transform. Convolving time, multiplying frequency.
The important thing that I found is that the effect of periodic extension-- I took a base waveform and turned it into a string of waveforms, right? So over here, I took a base waveform and turned it into a repeated thing, like so, in time.
The effect of the periodic extension, which we understand trivially in time, is to sample frequency. What I get in the frequency representation is samples. The samples being controlled by how long is the periodic extension. So what I get is samples. Instead of getting all of these, I just get part. I just get a uniformly-spaced sample set separated by pi over 2.
So then the effect of the periodic extension was to sample the Fourier transform of the base waveform. And that's the reason we can think about the Fourier series as being just a sequence of numbers. In fact, those numbers are the same. Or, they differ by 2 pi, right?
So I have to multiply these guys by 2 pi to get the Fourier transform coefficients, because the Fourier transform of cosine of e to the j omega not t, I should say, is 2 pi delta, OK?
So the reason the Fourier series coefficients are related the way they are, the reason they sample the Fourier transform, is because we can regard the periodic extension, which was convolved by an impulse train as being equivalently multiplying by an impulse train and frequency. OK?
That's what I wanted you to see. I wanted you to see that if you think about periodic extension-- which is moving left in this diagram. If you think about moving left, what you're really doing is sampling in frequency.
So that's the reason it came out having a sampled representation on the left. The periodic extension convolving with an impulse train was the same as multiplying by the impulse train and frequency, which has the effect of sampling the frequency response.
OK, so now the effect of moving this way was the same sampling in frequency. Well, what happens if you actually sample in time, which is what you do when you go up? It's got to be an [INAUDIBLE] like this, right?
So, the most useful thing about the Fourier transform is that the Fourier transform and the inverse transform look almost the same. That's the most useful property of the transform.
Now, the Fourier transform has a simple inverse relationship, and is almost precisely the same except for the annoying 2 pi and except for a change of sign-- e to the j omega t, compared to e to the minus j omega t. Except for those trivial differences, they're the same thing.
So we might expect that if we understood the effect of moving that way, which is convolve in time, multiply in frequency, you might expect an analogous thing to happen this way. And of course, it does.
So here, let's think about the base waveform-- same base waveform. But now what we're doing is sampling in time. And I'll just arbitrarily, for the sake of illustration, sample it with capital T equals a half.
Question is-- what would that sampling in time do to the Fourier transform? OK, sampling is time. That's something we understand completely in the time domain. What would happen in the Fourier domain?
OK, well that's a little hard to compare, because the domain of these two signals-- this is continuous domain, T. This is discrete domain. And that's kind of annoying. So it's kind of hard, because they're not on equal footing to compare them.
So what I can do is map the same information that was here into a representation over here that is continuous in time. The information over here was 0 everywhere, except one half-and-half. Here it's 0 everywhere except one half-and-half.
Anyone know why I used impulses instead of just making the function different? Why didn't I, instead of using the complicated-- wouldn't it be much easier if I didn't use the impulses? And if instead, I just said, OK, my xp of t-- why didn't I say xp of t is almost everywhere 0, except at that point, at that point, and at that point? Why didn't I do that? Yes?
AUDIENCE: [INAUDIBLE].
DENNIS FREEMAN: Right, let's assume that it is continuous. And that there's exactly three points that differ from the straight line at 0. Yes? The integral's 0. That never works. In fact, the most trivial representation that only has energy at three places is three impulses. That's the only way to get non-trivial energy.
OK, so I can't do that. I'm forced to do something like this. So now the idea is-- I switched to this kind of representation that has the same information, but has the same domain-- CT, continuous time. So that now I can think about-- what's the Fourier transform of this guy?
Well, it's the same idea. The way I can think about computing this thing from that thing is to multiply in time by an impulse train. What's the effect of multiplying time by an impulse train? What's the effect in frequency?
Multiply in time by an impulse train, what are you doing to frequency? Convolving frequency. So we take the original base waveform, but now we convolve it. And lo and behold, we convolve this signal-- which could be anything-- with this signal-- which is always periodic.
If you convolve in anything with a periodic, what do you get? Periodic, inevitable. So the fact that I sampled in time leads to periodic in frequency. That's the point. Sampling in time leads to periodic in frequency. That's always going to be true.
So we end up with a relationship here. The sampled waveform is going to be periodic. In here it's periodic, and 4 pi. That's a little different from what we've seen before. If we had looked at the x of n, we would have seen something that was periodic in 2 pi, but that's because the frequencies are different. One
The first one I showed is a function of little omega, which has the units radians per second. This one is a function of cap Omega, which has the units radians, they're different. One's frequency in discrete time. One's frequency in continuous time.
And there's a relationship, and that relationship is always this. That's the reason I write the two frequencies differently. One way to relate the two frequencies is via sampling. That's a way of taking a continuous time signal and turning it into a discrete time signal.
When you do that, if you sample by the sampling interval capital T, that dictates that this relationship's going to be like that. And it's very general. All you need to do is look at the definitions.
If you look at the definition of the DT Fourier transform-- that's this-- the CT Fourier transform-- that's this-- and just remember that we form this thing by samples of that thing.
So now, if you think about flipping the order-- and again, not worrying about whether or not it will converge. You just assume it will for the time being. Just flip the order of the summation and the integration. This then sifts out the value of little t equals n cap T, and we get a formula for the continuous time Fourier transform that looks just like the formula for the discrete time Fourier transform.
The only difference in the two is that one has discrete domain, n. The other has continuous domain, time. And by sampling, we convert continuous domain, time, into discrete domain, n.
So we're always going to end up with this relationship that the discrete frequency-- that's generated by sampling-- the discrete frequency is related to the continuous frequency multiplied by capital T. Capital T has the units of seconds. So that takes radians per second and turns it into radians.
OK, almost done, one more. So I've gone from here to here. What happens if I do a periodic extension? The answer was that you convolve in time, which is the same as multiply in frequency by an impulse train, which is the same as sampling in frequency. Convolving time, sampling frequency. That's what happened when I did this one. I just now did this one.
What happens if you sample in time? Sample in time is multiplied by an impulse train. Multiplied by an impulse train is the same as convolve in frequency. Convolve in frequency makes it periodic. Sample in time, periodic in frequency.
The last example is just to do this direction. What happens if I take the DTFT and try to turn that into a DTFS? Not surprisingly, what I have to do is the same thing I did in continuous time. If I want to change this DT signal-- which is aperiodic-- into a periodic DT signal, I do periodic extension.
I can think about the periodically-extended waveform, here extended by multiples of 8, as being the original waveform convolved with that unit sample train. And so if I convolve by the unit sample train, what do I do in frequency? Convolve by unit sample train in time, gives [INAUDIBLE] and frequency.
Multiply-- so I'm expecting that the answer will be sampled, and that's what happens. So I take the Fourier transform of this case. Which, like all Fourier transforms, is periodic in 2 pi.
And now I think about convolving it with this unit sample train, which has the same relationship as the impulse train. And the impulse train-- the train of impulses separated by capital T, turned into a train of impulses separated by 2 pi over t. Here separated by capital N, turns into impulse trains separated by 2 pi over n-- same thing.
So if this is 8 over here, I do 2 pi over 8, which is pi over 4. So convolving in discrete time is the same as multiplying by this impulse train, which gives me, then, a string of samples from that waveform. So the effect of going this way, which is periodic extension, which is the same as convolving in time, is sampling in frequency.
OK, so the idea, then, is that that's a way of rationalizing how the Fourier series would have worked. So the Fourier series was a discrete set of numbers exactly for that reason. I started out with something that was continuous in frequency, but I sampled it because I was multiplying by an impulse train.
So what I hope this did, OK, was dragged you through a couple of examples that will make things a little bit easier tomorrow. But I also hope that you understand how we went from a diagram that looks kind of complicated an arbitrary-- here we had DT on the top and CT on the bottom, periodic on the left and not periodic on the right.
The transforms had all that things turn 90 degrees. We ended up with discrete on the left instead of on the top, and we ended up with periodic in the top rather than on the left. And that's entirely because of the relationships between the various Fourier transforms.
So the idea is-- and you can understand all of those relationships by simply thinking about impulse trains. We take an aperiodic signal, turn it into a periodic by convolution in time, which is the same as multiplying frequency, which is the same as sampling.
We sample in time by multiplying by an impulse train, which is the same as convolving in frequency, which makes it periodic. So the hope is that this looks like more than just-- 1, 2, 3, 4, 5, 6, 7, 8-- eight independent equations, they're not.
It's really two equations-- the transform and the inverse transform-- and they're almost the same equation, except for taking into account the special properties of the different domains. OK, have a good time, and see you tomorrow.