Flash and JavaScript are required for this feature.
Download the video from Internet Archive.
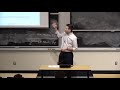
Lecture 8: Ridership Foreca...
The following content is provided under a Creative Commons license. Your support will help MIT Open CourseWare continue to offer high quality educational resources for free. To make a donation or to view additional materials from hundreds of MIT courses, visit MIT Open CourseWare at ocw.mit.edu.
PROFESSOR: So today we talk about ridership forecasting. Last time we talked about cost modeling. So we were looking at models to estimate costs of [INAUDIBLE] changes. Today we look at how ridership responds, which is the other big question the agencies, [INAUDIBLE] agencies have when a network will suffer changes. So we'll talk about route ridership prediction needs and issues, alternative approaches to ridership prediction and forecasting. We'll look at some examples from several agencies, notably the Toronto Transit commission and [INAUDIBLE].
We'll look at more advanced sort of DIS based models, simultaneous equations models, and show some examples of software packages that people use for this. So one note before we see is that all of this lecture focuses on short run root level prediction methods. We're not talking about regional level effects of, say, the land use influence on ridership, or the total amount. We're talking about specifically transit ridership. And it's a little more short run. So you'll see how that plays a role as we move forward.
OK, so what are the roles? Why do we need ridership models? Of course, predicting ridership is tied to predicting revenue, right? So when there is any change an agency is interested in, will they lose ridership or gain ridership, and what impact will that have on their fair bidding? So first role is predicting ridership revenues or total fare changes. This is the most typical example. All agencies go through periodic fare changes unless they are completely free.
So usually you want system-wide predictions. And the approaches are fairly specific calculations. So you might estimate some risk elasticity of the response, average response of ridership to fare changes. You could use a time theory [INAUDIBLE] model. That's a better approach if you want to control for external factors. And the best methods use a two-stage market segment model, recognizing that different segments of the population might have different elasticities, different responses to a fair change.
The second role is predicting ridership and revenue for general agency planning and budget [INAUDIBLE], so not necessarily fair changes. Here, again, we need a system-wide prediction. And the key elements are trend projection and another-- this is another place where a time series [INAUDIBLE] model plays a role. Here we're not necessarily looking at a response to something, not anything that the agency has control over, for example. But you might have the impacts of gas prices or other external factors, population growth, things like that, so trends, seasonality, things like that.
Then there's predicting ridership revenue as a result of service changes. This is the other very typical example. So here we are interested in more detailed root level prediction as opposed to system wide prediction. And therefore, we need to take into account the higher resolution set of variables, like the periods of operation, the headway or frequency of service, route configuration, stop spacing, and service type because of demand response to all of these factors.
And which factors affect transit ridership? We mentioned a few of them. Broadly speaking, we can divide them into two categories-- exogenous factors that are not under the control of the agency, and endogenous factors which the agency has some degree of control over. So exogenous factors include auto ownership and availability and operating costs. The cheaper it is to own and maintain and operate your own private automobile, the less likely you are to take transit.
Fuel prices and availability, same thing. Demographics-- it's age, gender, other income. All these things affect ridership and revenues. And then the activity system. So tractors, job centers, population density, things like that also are affecting ridership. So to the degree that there's an exogenous change in the system, population, employment distributions, these things can influence ridership, demand for public transportation.
So usually these things are assumed to be fixed in the short run. And we said that we would focus on short run predictions. Often these things are not considered in models. Some of them do. We'll look at some exceptions. And then there's endogenous factors. And these are the ones that an agency can control, so fare, the head of way which relates to how long people have to wait for service, route structure which relates to the walking time, access and egress times, and the ride time. And then there is crowding and reliability.
So obviously if we change frequency, we might change crowding. And also reliability through cycle time and available recovery time, as we've seen in previous lectures. So crowding is interesting because as crowding increases, that makes transit less comfortable, right? So that has an impact to, say, decrease the demand. And then if demand is to decrease, then that would really reduce crowding. So there is some sort of loop there, a causal loop which reaches some equilibrium, at least, when we look at snapshots.
Crowding and reliability are difficult to measure, especially reliability is difficult to measure without ADL. And crowding is difficult to measure without ADC. So usually these things are not accounted for in ridership prediction models if you look at practice. These things are often excluded. They are important, though, so they do effect demand. Reliability has a big impact on demand, according to revealed preference studies. OK, so--
AUDIENCE: Can I--
PROFESSOR: Yeah.
[INTERPOSING VOICES]
AUDIENCE: In the exogenous factors of auto availability, now in this world we're living where [INAUDIBLE], does that fall under auto availability, even though it's not a function--
PROFESSOR: But that is an exogenous factor, right? However you want to classify it. So the question is, transportation network companies like Uber and Lyft and Fasten, et cetera, all of these companies provide not necessarily auto ownership. But they do affect-- they provide a different mode of transportation that competes in some cases and other cases might complement public transportation. So that's an exogenous factor. It's not necessarily under the control of the public transportation agency. And therefore it's not-- I haven't seen any demand model that captures it, although there is some research here at MIT that is trying to look at that because that's an emerging trend. And it's important to see if we can capture those effects.
AUDIENCE: In certain places [INAUDIBLE].
PROFESSOR: We don't know, right? We're still investigating.
AUDIENCE: But you said we consider those factors to be fixed. But actually, TNC is an example of something which is rather dynamic in terms of pricing.
PROFESSOR: So I didn't say that we want to consider them to be fixed. I'm saying that if we look at what happens in practice, what the models that agencies have implemented and used to study, to predict ridership and revenue, those models, if you were to go survey the industry, and even some models in academia tend to assume these things to be fixed. And part of that has to do with the fact that the predictions of interest are shorter on predictions.
For example, I'm going to raise fares by $0.25. So should I include the effect of TNCs? Yes or no? Maybe. I don't know. But it could be a factor, right? So good question. OK. So the traditional approach is reactive. So if there is an exogenous change, the reaction is to monitor that change and maybe respond to it in service planning process. An endogenous change, such as a fare increase, it means that we're sort of modifying the system and if we see a ridership change, we might then react to the ridership change.
So it's always catching up to what we see. So no attempt to anticipate impacts prior to the changes. Current practice, typically little attention is paid to the problem in many public transportation agencies, unless it's fare increases or a major capital project, right? So we just set an example of TNCs being a different thing. We haven't really seen serious attention until very recently of what impact that would have on our ridership.
Typical or traditional planning models are ineffective. Generally, they're not lethal enough to apply them for a short run prediction. So we're thinking here about four-step modeling. It's expensive to run. It takes time. So for short run predictions, they tend not to have the high [INAUDIBLE] solution that you need to decide something about a specific bus route, for example. So these are typically not applied to specific case studies. Yes, question?
AUDIENCE: What is TNC?
PROFESSOR: Transportation Network Company, so Uber, Lyft, Via, Fasten, all these providers. So ad hoc judgmental methods dominate. So now these methods are not the most accurate, obviously. But they are useful, especially if there's a small change and you have many proposals on the table, you have people experienced with the area or the network. They might have a good sense of at least the direction and the overall level of change that one might expect.
But they tend to be quite inaccurate for disaggregate predictions, long term predictions, or very large changes in the network. So if you're doing something small, maybe OK. If you're doing something significant, not so much. OK, so we have four approaches here to modeling, right, is it? Ordered from in order of increasing sophistication, starting with professional judgment, then non-committal survey techniques, then cross-sectional data models, then time series data models. And let's talk a little bit about each one.
Professional judgment, so this is when you bring in as an agency either experts within the agency or consultants whose job is to predict ridership. They have a lot of experience doing so. They might have local knowledge. And of course, the problem is that there haven't been any scientific studies that indicate the accuracy of these methods. They tend not to be reproducible every time you call someone in. That's sort of a one shot deal, right? So you're not really able to replicate those results easily or understand what led to the prediction.
This reflects lack of faith in formal models in some cases, lack of data or technical expertise to support the development from all, or a lack of time or budget to do so. And maybe most importantly relative on importance of the topic of ridership prediction in general to an agency is compared to the impact of changes or impacts on existing ridership. So agencies tend to worry more about the reaction of the public that currently rides their service than the general increase or decrease in ridership.
Although, they do they are concerned about that. They worry more about the last four. So any questions on professional judgment? We all know what this is, right? OK. Survey-based methods, the next level of sophistication. So here we go out and ask people, would you ride a bus route if it were extended to Kendall Square. Or if we provide a new train line, would you do this instead of driving your own car? Things like this. And how much would you pay? So you can survey people and ask them, would you take this or not?
You lay out a proposal and you ask people for their reaction. This isn't called non-committal because people are saying that they would do it or not do it. But they are not committed to doing so. And there's no promise that they will do so. So the stated preference might be quite different from the revealed preference if one were to actually follow up with what these people actually, the-- question?
AUDIENCE: Is there a sense of accuracy [INAUDIBLE]?
PROFESSOR: It varies widely. And there are many papers and lots of studies that have looked at this. So--
AUDIENCE: [INAUDIBLE] can you just give us a sense?
PROFESSOR: So, yeah, right here. So typically we have a survey. You might ask, say, 500 people or 300 people whether they would do this or not. A typical example is a roadside survey where you stop cars and you ask them, would you have taken a park and ride using the metro system or an express bus line? And they would say yes or no and off you go. So noncommittal, right? There's no-- there's no necessity to actually do that if you said yes.
So the problem is that there's this noncommittal bias. People tend to say yes more than they would actually do so. This has to do with sort of psychological factors. Sometimes the desire somewhat subconscious to be friendly-- there is a perception that the person doing the survey is interested in [INAUDIBLE] result. And therefore, as human beings, we try to be friendly or try to be positive, right? This is psychological. It's subconscious. It affects results.
So what happens-- what ends up happening is that there's an adjustment factor for the non-committal bias. And this is where professional judgment comes in. So you do have some survey technique. But at the end, you need this fudge factor. You multiply it by anywhere between 5% and 50%. And what that range-- what that specific number is depends on the specifics of a problem, who you survey, how many people you survey, the experience with the person conducting the study. Any questions on non-committal surveys?
AUDIENCE: Yeah, maybe it could also be that I would say yes because I personally wouldn't use it, but I have a kid who might use it. And so I know someone who would use it. So I say, yeah, yeah, I use it. And I might even be--
[INTERPOSING VOICES]
--blatantly lying about myself. But I say, oh, well, if they provide this service, then my kid will use it. And so I want to say yes.
PROFESSOR: I'll repeat the comment for the benefit of people listening on the web later on. So the comment is that another reason for a noncommittal bias is that you might know people who would write it. Or another instance of this is, maybe you ask, would you today have done this? And maybe today you wouldn't have, but you think, typically I would, right? So then you want to be helpful and give what is most typical. Problem with that is that if everybody's is doing that, you have a bias, right?
Because you are doing, hopefully, a random sample. So you could have surveyed my spouse or my kid, but you didn't. You picked someone at random. If everyone you pick is trying to answer for someone else or answer for what I would have done on a different day and that all leads to a positive bias, then in aggregate, you could have a problem. So it doesn't help if people are dishonest and trying to be helpful, right? Any questions-- yeah?
AUDIENCE: Yeah. Is there any way to randomize the direction of the question and--
[INTERPOSING VOICES]
PROFESSOR: Yes, but that's-- so that's the next method. So we'll talk about that right now. So this is not recommended because this bias can be significant and it's hard to quantify. Now there's something called stated preference analysis or conjoint analysis. And is where we apply statistical techniques to try to correct for that bias.
So this originated in mathematical psychology. And what we do is that it's a rigorous, detailed sign of the experiment. And usually what you do is that instead of asking people if they would take this or not, you say, would you rather take this option or this option? And you put some bundles. And you ask them to trade them off multiple times. You say, well, what if it cost one more dollar? What if-- and you have multiple levels. So people start contradicting themselves sometimes in these trade-offs. And you can detect the biases using those contradictions, or at least you can rank the relative importance of different factors to that person and then apply those factors to correct for the bias.
So this is, again, this area's evolving. It is being applied already by high end consulting firms and also by researchers. So if you find research papers on the [INAUDIBLE] stated preference surveys, you'll find these kinds of techniques being applied. This is very useful for new services or new service areas. Why is that? Why would this technique be especially useful for that? Yeah, [INAUDIBLE]?
AUDIENCE: Because there is an existing status quo that they're implicitly comparing against.
PROFESSOR: If there isn't any service to start with, you're talking about a new line or a new area of service where transit isn't really present or not present to the degree that you're proposing, then there's no good way of looking at the existing amount and extrapolate from there. So then you have to ask people. And this is a method to correct for [INAUDIBLE] bias.
One more comment-- the MTA useless in the 1980s. And they concluded that they would raise fares to repay bonds that they had taken for capital projects. So it's been applied successfully. The next method or family of methods is cross-sectional models. So here we develop a model of demand as a function of route and demographic data to explain ridership. So we're looking at a snapshot at a single point in time or, say, we pick a period of three months altogether and we look at the ridership over those three months across different bus routes in the region.
And then we observe that different bus routes have different demand and they have different frequencies and they run through sectors with different population densities and different employment densities. And we tried to relate the differences in demand on those routes to the ridership to those characteristics. This is called a cross-sectional model. So again, we explained the differences in demand as a function of the differences in characteristics.
And there are different approaches here. Rules of thumb is the simplest. So we might say if it's key bus routes, then it has this much demand. That's a very sort of simple, crude example. The similar routes method is I'm going to convert this bus route to-- I'm going to add frequency to it, say, increase its frequency. And then it's going to look a lot more like this other bus route that I have in another part of the network. So ridership should look a lot more like that.
Maybe I separate my bus routes in the network into high ridership key bus routes that have certain high frequency and other less frequent bus routes that run on less dense parts of the network. And I take those averages and I apply them to extrapolate, right? So that's a similar route. You find similar routes. You use those to make a prediction.
Then there's the multiple factor trip rate model. You, again, look at-- you do a regression, maybe. You can do this with regression or by separate factors. So those are the last two bullets here. So you essentially run regression where you have-- maybe you load up a GIS layer and you have population density, employment density, frequency. And out of the regression comes these factors where you can control for multiple factors at the same time.
Any questions on cross-sectional methods? [INAUDIBLE]
AUDIENCE: Because these models generally focus only on endogenous factors and not exogenous, so they don't physically capture the demand, how the demand is [INAUDIBLE] with difference to other factors. So how can this be generalized so that second point is that similar routes-- like, it can be generalized. One that uses less data or is shallow in its nature could not generally be generalized, right?
PROFESSOR: Correct. Yes. These models are hard to generalize because they don't-- actually, any cross-sectional model is hard to generalize, right? Because you are looking at a specific time window of analysis. So you are already, in doing so, excluding any longer-term trends that are often exogenous, like fuel prices and population changes and things like that. So yes, by doing a model, even the most sophisticated of these, which is a multivariate regression model, if you have any factors that you leave out, that can lead to biases in the estimators, right?
So will you actually see that bias? Well, that depends on how short run of a prediction are you trying to do? Is your prediction going to be applied to a period in the short term that is very similar to the period you have now where these exogenous factors have been out, have not changed, and therefore there is no impact on the bias of this model.
Or are you going to apply this-- abuse this, let's say, misapply it-- abuse it to predict outside of that window, outside of that domain, where you do have changes that you did not include in the model, and they have affected ridership? Yes. Any other questions about cross-sectional methods?
OK. Let's move on. A little bit about regression. So this is an example of how an agency might respond to increases in demand by increasing frequency and vise versa. So you look at-- you start with an observation that ridership is quite high and the frequency is some amount right now. And then you react to that. So as an agency, you might say, well, this bus route is quite crowded, therefore, let's increase the frequency, right? And let's increase the frequency.
That's going to have an effect on the demand curve. So all of these lines, d, are demand curves. And this demand curve shows the response of ridership to a change in frequency, assuming everything else stays the same. But there are also other changes that are not included here. So you might increase frequency but there might be other exogenous factors, exogenous in this case because frequency is the only variable being shown here. Any other variable that increases demand will not result in a change along the demand curve. It will actually shift the demand curve, right?
So you have demand curves going in a third dimension, increasing demand in a third dimension due to other factors. So typically, again, agencies are reactive. They sort of observe an increase then they move to the next one and they're always thinking about one demand curve, if at all. And so it's better for this reason to look at multiple factors at the same time instead of just one factor, such as frequency.
OK, so these are some typical transit elasticities. We have elasticities to fare, to headway, and to total travel time here. And so these are the typical values if you look at many studies and take averages, this is what you find. So elasticity to fare, negative 0.3. Let's do a quick recap. What is elasticity? Henry?
AUDIENCE: Percent change in demand over percent change in price.
PROFESSOR: Yes. That's-- so the answer is percent change in demand over percent change in price. I want to make two adjustments to that definition. That's often how it's calculated. But it's actually the derivative of the curve, not necessarily a percent change. That is an approximation of the derivative. And the other is that it's not just to price. In this case, we're looking at change to fare towards headway or to other disutilities, so any other factor that affects disutility. So what is the difference between-- what is negative elasticity?
[INTERPOSING VOICES]
AUDIENCE: As all those variables are going up, your ridership is going down.
PROFESSOR: OK. So as fare increases, the ridership goes down. Great. What is the meaning of an elasticity with an absolute value between 0 and 1 instead of greater than 1? Or, rather, what is the difference between an elasticity that has absolute value less than one versus one that has an absolute value greater than one?
AUDIENCE: Relatively inelastic?
PROFESSOR: OK, so--
AUDIENCE: So for example--
PROFESSOR: --inelastic versus elastic. Those are the terms used to explain the differences, right? So what is an elastic change?
AUDIENCE: A change in price would not necessarily diminish the demands from the quantities demanded by people. You could have--
PROFESSOR: Is this fair value of [INAUDIBLE] inelastic or elastic?
AUDIENCE: Elastic.
PROFESSOR: This is inelastic.
AUDIENCE: I'm sorry. Yeah, inelastic, yes.
PROFESSOR: Yeah, this is inelastic. So what does that mean in practice?
AUDIENCE: A change in fare--
PROFESSOR: So I know that--
[INTERPOSING VOICES]
AUDIENCE: --would not lead to an equivalent change in ridership.
PROFESSOR: OK.
AUDIENCE: It would lead to less than the equivalent change in ridership.
PROFESSOR: OK. Therefore if you increase fares, you will lose some ridership, but not enough to lose total [INAUDIBLE].
AUDIENCE: Right.
PROFESSOR: Right? If this volume were greater than-- have an absolute value greater than 1, then you might actually decrease-- the response in the [INAUDIBLE] might be so strong that you might end up loosing revenue because the amount is so much lower. So if you overprice an item by a lot and nobody buys it, you might end up getting less, right? In transit, typical [INAUDIBLE] are inelastic. So this is important. This means that any transit agency who needs more federal revenue can probably increase fares and know that they will get more money. That's sort of one that keeps conclusions of this.
The range of these values is from negative 0.1 to negative 0.5. The elasticity of headway is a little larger in magnitude. So the response of demand to frequency is stronger a little bit than the response to fares, and often overlooked in ridership spikes. And then total travel time, people respond very strongly to that. So
OK, then there are these important other points that I want to go through. Small cities have larger fare elasticities than large cities. Let's talk about each one and suggest the reasons for them. So why would small cities have larger elasticities?
AUDIENCE: The absolute value?
PROFESSOR: Yes, absolute value. So you're saying that they are more elastic, right?
AUDIENCE: More sensitive.
PROFESSOR: Yeah, more sensitive. So small cities-- ridership in small cities is more sensitive than ridership in large cities.
AUDIENCE: There's not as much of a-- there's not as much utility lost by switching two different modes. There might not be as much traffic if you drive instead. Some fare might be more of a reason that people that use it. And also that parking might not be as costly so that if you raise the fare a little bit, you might get more expensive than parking.
PROFESSOR: OK, so you have other sort of characteristics in large cities that you don't find in small cities, such as parking costs.
AUDIENCE: That would be a way of--
[INTERPOSING VOICES]
PROFESSOR: I'm trying to summarize it, and also repeat for posterity. [INAUDIBLE]
AUDIENCE: Also in urban cities, since these elasticities are being computed for the population as a whole, there are more people dependent on the mass transit system.
PROFESSOR: That is very important. This is one of the key factors affecting this, actually. So you have a much larger dependence on public transportation in large cities. And also-- yeah.
AUDIENCE: Is it really the size of the city or is it the density of the city--
PROFESSOR: It's probably density. These are correlated. So maybe this could have been more carefully chosen. And you could say older cities, as well. I would argue older pre-auto industry, pre-highway expansion cities as another way of putting footings. Yes? More ideas about this? Henry?
AUDIENCE: I don't know how you're defining this--
[INTERPOSING VOICES]
Sorry, sorry, sorry. Someone first--
PROFESSOR: Henry. OK. You decide.
AUDIENCE: I was just curious about, how do you define small cities? Because for example, I'm thinking in my own country, a small city would be a city of around 200,000, 100,000 people. And over there, for example, an increase in the fare would have this characteristic because you can still walk [INAUDIBLE]. So walking is still an option. And I think it's kind of correlated with what you were saying about
[INTERPOSING VOICES]
PROFESSOR: So all of these things have to do with where you apply them. And maybe they're not completely generalizable. But I think this observation has more to do with the North American and European context. So but that's a good observation. Henry?
AUDIENCE: I think smaller cities also have less extensive coverage.
PROFESSOR: Yes, so our imports--
[INTERPOSING VOICES]
AUDIENCE: --are already trained to not rely on--
PROFESSOR: So the public transportation supply might be quite undesirable or inefficient. And so therefore--
AUDIENCE: And if you jack up the price, that will make it less desirable.
PROFESSOR: Exactly. OK, great. OK, let's move to the next one. Bus travel is more elastic than commuter rail rapid rail travel.
AUDIENCE: Simply, bus travel is smaller traveled distance and there are possible another alternatives.
PROFESSOR: Yes. That's the key reason. So if you have a shorter distance, and bus travel tends to be shorter distance, then you are more likely to have other options, whereas commuter rail, you might be able to drive maybe your own car, so et cetera. So there are fewer options in general to the public for a longer distance travel. And commuter rail and rapid rail travel tends to be longer distance.
AUDIENCE: Commuter rail, rapid rail, certainly in Russia, have a time advantage, tend to have a time advantage over the private vehicle.
PROFESSOR: That's true, as well.
[INTERPOSING VOICES]
This is another reason--
AUDIENCE: The bus never has--
[INTERPOSING VOICES]
PROFESSOR: --competitiveness of the mode in rush hour where buses might be stuck in traffic and rail is not stuck in traffic, right? So that drives the elasticity more because so many more people are traveling in rush hours. Great. Let's move on to the third one, off peak fare elasticities are double the size of peak fare elasticities. Lee.
AUDIENCE: The difference between leisure and business travelers where you can change your trip decision if it's a leisure trip, but not if you're going to work.
PROFESSOR: Trip purpose, right? So peak travel tends to have a higher proportion of business and you don't have the option of not doing the trip. So you must do the trip. What else? Something related to that. Yes?
AUDIENCE: Again, congestion has a role. To consider the idea of commuting by Uber in New York City, it might not make sense to do that during a peak period if you're going to be sitting in traffic on the road and the subway has, then, a time advantage. But returning home late in the evening, that time advantage of transit could be eroded because there's less congestion.
PROFESSOR: Right, so congestion can have a major effect. And congestion is larger at peak. And therefore, it could make modes that are segregated have their own right of way more-- so related to the previous one. What else? Eli.
AUDIENCE: [INAUDIBLE] I think it was discretionary ridership during--
PROFESSOR: Very important, yeah. So can you elaborate?
AUDIENCE: Yeah. During the off peak hours, you have more people who have no other choice to get around, use transit.
PROFESSOR: And why not? Why might these people not have other choices?
AUDIENCE: Because they are lower income.
PROFESSOR: Lower income, right. So lower income people who work may have no other options. This may be the only option they can afford. And therefore, they are less sensitive. So if you raise fares, well, it doesn't look good for them. But that's the only alternative for them. So they'll react. Great.
Short distance trips are more elastic than long distance trips. We already covered that in the context of rail, so let's skip that. Fare elasticities rise with income. This should be straightforward.
AUDIENCE: More options if you have more income.
PROFESSOR: If you have more income, you have more options. You can afford all the other options. So there you go. And what about fare elasticities fall with age? So older people, senior people are less sensitive to changes in fair. Why is that? Emily?
AUDIENCE: Higher income [INAUDIBLE]. They tend to have fewer options.
PROFESSOR: Why?
AUDIENCE: Because, well, walking might not be an option.
PROFESSOR: So the active modes might be less desirable?
AUDIENCE: They might not have a driver's license anymore.
PROFESSOR: They may not be-- have a driver's license anymore.
AUDIENCE: They might have failing vision and therefore not be able to drive or have other--
PROFESSOR: That's exactly right. OK. Of all trip purposes, the work trip is the most inelastic. We've covered that. And then promotional fare elasticities are slightly larger than short term fare elasticities following permanent fare additions. So--
AUDIENCE: Can you explain that? What does promotion--
PROFESSOR: So if you do a free fare day and you put ads everywhere or something like this. The response that you will get is going to be much stronger than if you are on a routine basis raise fares and-- yeah. So why does that happen?
AUDIENCE: Maybe the increase in demand due to the lower--
[INTERPOSING VOICES]
PROFESSOR: Increase if you reduce them or decrease if you increase them, right?
AUDIENCE: If you increase fares, they-- Better promotional if you have lower fare, right?
PROFESSOR: Yes. But we can extend this to how frequently this change happens and how much buzz is there about the spare change. Yes?
AUDIENCE: People feel like, oh, it's happening once. I've got to do it now, or I won't--
PROFESSOR: Yeah, so the psychological effects of marketing, the effectiveness of marketing on-- so if you look at agencies that every year at a certain dates raise their fares more or less with inflation, there's much less sort of the reaction-- the public reaction is much lower. So--
AUDIENCE: Are those promotional fare-- free fare day, free fair weekend, are they effective? Does anyone--
[INTERPOSING VOICES]
PROFESSOR: That's what your goal is, right?
AUDIENCE: No, the goal is--
[INTERPOSING VOICES]
PROFESSOR: But it might be effective at increasing ridership temporarily if you have some event on the city and you want to-- if you want people to use those modes instead of drive, that might be a success. So you want to increase the efficiency of vehicles by decreasing load times so people don't have to interact with a fare box, you can open all doors. People go in and out. That might be a success. So--
AUDIENCE: Paris once did that for exposure, to try to lure people in because they already-- yeah. But the question is, is it effective?
PROFESSOR: Well, again, it depends on what your measures of success are. Emily?
AUDIENCE: Also, promotional fares might attract more discretionary trips than people--
PROFESSOR: People might say, oh, I want to try this, right?
AUDIENCE: Yeah. Whereas permanent fare revisions tend to effect people who are just doing their commute.
PROFESSOR: And therefore--
AUDIENCE: Who are therefore less--
PROFESSOR: Right. So if you do any kind of promotion, you might make people that tend not to even be aware of transit fares be aware of this option. And so you might lure them in. Yeah, Henry?
AUDIENCE: There are also a lot of cities that do free transit on New Years Eve to reduce drunk driving--
PROFESSOR: Yes. So that's another example. So on New Year's Eve, to reduce drunk driving, offer free fares. Yes?
AUDIENCE: So it's safe to say that promotional fares are only useful for short-term effects but they don't have any effect on long-term [INAUDIBLE].
PROFESSOR: I don't want to make that broad claim, but I think I agree with the premise of it. OK. OK, let's revisit a model that we saw on an earlier lecture. This is the TTC elasticity model, which we saw is used or has been used to predict the rider changes to service changes, so ridership response to service changes. So this is the-- let's recount. The total weighted travel time is a sum of four components-- the in-vehicle travel time, the waiting time, the walking time, which is the access and egress and transfer time, and a penalty for the number of transfers that you have to take for that [INAUDIBLE].
And then there are these weights. 1.5, so what does that mean, 1.5 in this equation? I'll generalize this utility equation, a systematic disutility equation. It has some factors before each of the explanatory variables. Sonia?
AUDIENCE: People hate waiting. So it is extra--
PROFESSOR: How much more do they hate waiting?
AUDIENCE: They hate waiting 1.5 times as much as they hate being in their vehicle.
PROFESSOR: Yes. Exactly. So now these numbers are nice and round. So TTC did not conduct a revealed preference study to estimate it from data. But they align somewhat well with revealed preference studies. So the order of magnitude, at least, is correct. I've seen factors as high as 3 for waiting time, as low as one, in cases of rush hour and, say, metro. So that gives you a range. Walking, I've seen 5, 10. I've seen higher. But penalties for number of transfers, I've seen five minutes, 10 minutes. So people hate transferring.
This is the street bound. So this is 10 minutes for every transfer you have to make. Great, so what happens? You compute the total weighted travel time before and after. So you have the current situation and then you have a proposal that will alter these values and result in a new total weighted travel time. And then we apply some elasticities. So these elasticities defer by period. So during the peak, we have negative 1.5. Mid-day, we have a higher response. This aligns with what we just said. Peak period ridership tends to be more inelastic.
So we have a stronger response during the middle of the day. And if you look at early in the morning or evening, even stronger. So you have higher values. Question, why are these values have a [INAUDIBLE] greater than one? This is a trick question, in some sense.
AUDIENCE: Travel time is-- ridership is dependent on travel time. It's very elastic.
PROFESSOR: OK. So that's one way of putting it. So I guess the observation is like, these are now fair elasticities. And they are not frequency elasticities. They are elasticities of total weighted travel time. And these are four components coming together and we can't really think about it in terms of fare elasticity and single variable, really. These are multiple effects coming together.
So great. So do we understand how this applies? We have a service proposal. We estimate the effects on riderships in [INAUDIBLE] waiting time, walking time, and number of transfers. We know how many people would ride before and after, or we-- well, we don't know that. We want to predict that, but we know for each [INAUDIBLE] how each of these components would be affected. And then we use the [INAUDIBLE] elasticities to predict the change. OK? Questions?
AUDIENCE: Yes. Is the 10 in front of in trans, does that mean the average transfer is 10 minutes, or does this mean people just really, really don't like transfers because they want a simple route?
PROFESSOR: So this excludes waiting time. This is the fact that I have to transfer is so annoying that I would rather spend-- or it would be the same as spending extra 10 minutes in a vehicle. If you would give me a situation where I have a single ride option that takes 10 minutes longer and you gave me another one that takes 10 minutes less but I have to transfer, I wouldn't know which one to take. They would be equally desirable or undesirable. Yeah.
AUDIENCE: Would you say that in a more-- if this was a more distinct model, then the penalty for transferring rail to rail would be less than the penalty for transferring--
PROFESSOR: Yeah, of course. You could make this much more sophisticated. And you could say, are there escalators or not? You know, you could argue, are some parts of the city, say, less safe, and therefore walking time is very undesirable, especially at night? So you could really go disaggregate and apply all these behavioral impacts. So yeah, you can make this more sophisticated. But you have the idea, right? This is an elasticity-based model. OK.
Direct demand regression models, again, we covered a little bit of this. We match census tract data to route service characteristics. This is hard without GIS tools. GIS is a geographical information system where you have a map and you load layers of data showing things geographically like population density and a point of density. Your bus stops, your bus lines, your train lines. So we use GIS tools to apportion population and employment data to different bus routes.
So you know that the bus route goes along these centers that have certain properties. Then we run a regression of trying to explain ridership as a function of those characteristics. And often what happens is that you have large or high significance of dummy variables and track-specific constants. So the general variables like frequency, the variables that apply to all the bus routes together, tend to be maybe not as significant statistically as these very specific variables that apply only to one or two bus routes.
And that means that in some sense, we are specifying a model that is over fitted and there are some issues with this. So the other issue is that these models tend not to recognize network interactions, or they never do, rather, because if you have two bus lines running through the same employment center and going to two different places, both at the same frequency, then presumably they should share the demand from that employment center, right?
If you only had one bus route and you leave the characteristics the same, then that ridership would be a lot higher. This model doesn't really account for that, the complementarity or competition of the supply in the network. So that's an issue. And then the relationship between supply and demand is not-- the interaction is not captured. So we can-- there are alternative approaches. Simultaneous equation models are capable of addressing the relationship between supply and demand.
And then some other models are able to capture the relationship between competing and complementary routes. And we'll look at an example. That's the logical next step beyond direct demand models. And then the full network models which sort of have-- you have the GIS. You have all the network. Those deal explicitly with competing complementary routes because people in that mall have the option of any bus route and they see all their options. And in these models you can include [INAUDIBLE] distribution of model split effect.
So that's the next step beyond-- the logical next step beyond the TTC-type model-- the elasticity model that we just discussed. Both of these require GIS-- some computer representation of the transit network and the service area. This used to be a challenge. Not so much anymore. Most agencies put out TTFS data and write layers showing where their bus stops are and so this is now commonplace. This is not an obstacle anymore. Questions about this? Henry?
AUDIENCE: What is TTC?
PROFESSOR: Oh, the Toronto Transit Commission. It's the bus agency in [INAUDIBLE]. Other questions? OK, so let's look at a simultaneous equation model. So here we have ridership of some route, I, on some segment, Z. So we divide up bus routes into segments. And we have many bus routes and many segments.
And we say that is a function of the level of service provided. So think of that as frequency, the level of service provided on that bus route on that zone, and other external factors. Those external factors are demographics, socioeconomic characteristics of ridership, the population density, things like that, so maybe exogenous. Then you say supply, on the other hand, so my level of service, is a function of the ridership I have right now because if I have more riders, I will provide more service.
Also, it is a reaction to ridership as it was in the past. And it's also a reaction to other external factors. So these two equations are simultaneous. You have to solve them at the same time. And they could take different functional forms. We are specifying here at a sort of conceptual level. [INAUDIBLE]
AUDIENCE: Yeah, is that in the second version, is it a typo or do we actually consider the overall ridership in the previous time period?
PROFESSOR: It could be a function of the ridership in the past. In other words, this is a way of saying, my supply right now, my supply today, is a function of what ridership used to be.
AUDIENCE: No, what I mean is, should that be segment dependent or do we then scale it up to the entire [INAUDIBLE]--
PROFESSOR: Oh, it could be segment dependent or not. So you're saying why not have z? You could. If you want to add it in, go ahead. Again, this is conceptual. So what I'm saying is this is not a specific model. Rather, this is a conceptual description of how you set up a simultaneous equation model. So the point of that variable is to say that you could include the effects of previously observed ridership.
So don't think about this as a specification of a model. I'll put this on Cellar. This is the paper that describes this in detail if you want to look at the specifics of such a model. Here's an example from Portland Tri-Met on that paper. You have route 19 and route 20 which were on, in this diagram, kind of east-west. So route 19 runs along this line right here. And for each bus route we have a [INAUDIBLE] area.
So route 19 captures this part on top and also this middle part that is shaded both ways. Another is route 20, which runs along this line. And it captures people here and also people in this area. So this area in the middle is-- people from that area go to route 19 or route 20. So in that area, route 19 and 20 are competing.
Then they kind of merge and go through zone 0 and they are fully competing. They are-- maybe it's a branch and trunk system. And then there are these other bus routes, route 70, route 75, 21, and 22, that are across South-North, North-South, that corridor. And we say that these bus routes are complementary because people might transfer, people going from somewhere on the south might transfer to one of these route 19 or route 20. So the fact that route 70 or 75 is there, it means that people get-- more than not will be attracted to the corridor.
So then we quantify the degree to which bus routes compete with this overlapping population fraction percentage or proportion. So this is the percentage of the fraction of population that is on the overlapping portion. So we look at route length in route 20. We're saying how much relation is on this overlapping area divided by the sum of the population captured by route 19 and the sum of population captured by route 20?
So that gives you an idea of the degree of overlap. We also measure population in each catchment area for each bus route. And we can then capture inter-route effects. So we do that by modifying the equation two slides ago and adding this third question here. So before we have ridership as a function of level of service and external factors. Now we're adding the ridership on competing bus routes. So these are bus routes that are competing on the same segment. And they run with overlapping population.
So to the extent that this increases, the ridership on Route I decreases. And then we also consider the ridership on complementary routes across this catchment area. And to the extent that this increases, the ridership on route I increases. We also consider the degree of population overlap and the external factors. Again, this is conceptual. If you want to look at the specifics, I suggest you look at the readings. I will post the paper.
So this is a way of modeling the effects of complementarity and competition. OK, a different approach, moving down the list of sophistication of modeling approaches is the network-based modeling analysis approach. So here we have a transit origin destination matrix as an input. We measure how people are using the system right now. We have layer like a DTFS layer with the base transit network. And we put that into a model. And then we tell that model, we're going to make a change. We're going to add a bus line or we're going to increase frequency or we're going to increase fares, whatever it is.
And that model will look at the current transit demand and predict changes. OK? So that's the general idea. It'll also output not just ridership and revenue but things like rider attributes. You might have information about income and things in GIS layers that can be allocated to different services. And there are three levels of analysis for how to use-- three levels of detail on the OD matrix. The first one is fixed transit flows.
So again, in order of sophistication, starting with the least sophisticated, fixed transit flow. So we look at the current transit relief flow. This could be from a telephone survey or from any kind of survey. Or we have, now, modern techniques based on AFC and inference models that we will discuss shortly in the next few lectures. So we have the OE matrix, current OE matrix for transit. And we assume that the demand for transit won't change as a result of the service changes in the short run.
So that could be a strong assumption. That's typically what we do. That's typically how these models work. Again, we're more interested in the impacts on sort of current riders than on big changes. The next approach is to say the total demand is fixed if I look at the demand across all modes, including car and bike and TNCs and all these things. So that you have to get from surveys, the kinds of surveys that are used to input data into a four-step model.
But then you allow the model split to change. So as a result of service changes, people might switch between modes. They might go from transit to car or from car to transit. So that's preferable, especially if you have significant service changes. But it's not often put in place. And then there's the variable total [INAUDIBLE]. Now you require all the steps of modeling, the four-step modeling. And you are essentially allowing the total demand to increase or decrease as a result of service changes or fare changes.
Typically no one does this. And one could argue this is unnecessary. Why? Because we are looking at short-run changes. And total demand shouldn't change in a very short horizon as a result of at least the typical service changes. So this kind of model is great if you want to capture the influence of land use patterns on long term demand, for example.
If, however, you were to bring in a new rail line that connects two employment centers, that's a change in land use. If you somehow think that some change that you will do in your city, on your transit network, will bring in a change in population or a change in where people live significantly, then you have to do this. That's the only case where this would be useful.
Otherwise, you probably are thinking in the short run total the amount is the same. People might switch between modes. So you will get step two. And if it's a very small change, you could even get away with step one, where you look at current demand and people might change when they make the trip or what bus route or rail line they choose, but not necessarily the level of ridership.
OK, there's different modeling and analysis packages. Let's show three of them. MADITUC, EMME/2, and TrainsCAD. Have anybody heard of any of these? Raise your hand. OK, about half of you. Great. So let's start with MADITUC. It was developed in Montreal. It was a common technique in Montreal. It requires only survey data that includes route choice information. So this is a line level model, essentially.
It's designed for transit service planning and it uses all or nothing assignments. So it looks at, for a given [INAUDIBLE], which is the best path on the network. And then it puts all of the people on the [INAUDIBLE] into that one path. So everybody makes the same decision kind of thing. And it doesn't have built-in data analysis or a graphics capability. So you take the output of it and you put it somewhere else.
These people were using SAS and other software to generate graphics and to generate plots and calculated statistics. It's been used in four of the Canadian cities-- Montreal, Quebec, Toronto, and Winnipeg. And here's an output from of that data, plotted with a different software. So this is-- you can sort of see the business plot of OD. I'm not sure, actually, how it's-- if it's destinations for a given origin or what it is. It might just be-- oh, this is number of entries. Yeah. I don't know French. But if anybody--
AUDIENCE: It's number of entries by foot or--
PROFESSOR: On foot.
AUDIENCE: On foot?
PROFESSOR: Yeah. What is that? I don't know that last word.
AUDIENCE: [INAUDIBLE]
PROFESSOR: Anyway. And then it says AMP 629, right? OK, great. EMME/2. So this is multi-modal equilibrium. It was also developed in Montreal. It's a little more sophisticated. It was developed with the general regional transportation modeling package. It can generate transit OD flows from a travel demand model, which the other package needed that as an input. It has a link node oriented approach instead of a line level approach. So you can have bus stops and you can-- it has more detail, [INAUDIBLE].
There are two options for a transit assignment. There's aggregate zone to zone, flow, multi-path assignment. So that's usually not precise enough because it's zone to zone. And then there's a disaggregate point-to-point trip assignment procedure, which you could know which bus stops and lines and everything.
It is probabilistic or multi-path. So now you have an OD pair and different alternatives of how to get from both O to D. And one may be preferable, but people might be split on which lines they take. And more, maybe 2/3 of people go one way, and a third go the other. And some equilibrium is reached this way. It's a standalone package. This has all the graphics built in. And here's a picture.
So yeah. The TransCAD, this is much more local. It's over caliber develops in Newton. It has good tools to edit transit networks. It has an API that allows you to add your own models. So if you have your own model split model, you can put it in. It has good interactive computer graphics, database, built-in database. It has a network assignment procedure, lots of display options and output formats and very general purpose.
So typically, you use a transit network database for this. So that means geocoded transit links and nodes. You have mappings of transit lines onto network links and nodes. So you have essentially a street network, right? And you say, this bus route goes from-- along these road links, crossing these intersections. And transit lines are modeled with some attributes. So headway is by service period. Travel times, models, mode of service or bus, subway, et cetera.
And there's also system attributes, like operating cost data, energy consumption data, and fares. Some of this is-- well, these things are put in. And they then are used to compute outputs. So hybrid outputs. So you get ridership by link and by line, boardings by node, by line, by link. You can have OD travel times, so in-vehicle time and also including the access and egress portions, transfers, et cetera.
Revenue predictions, operating cost predictions, energy consumption predictions, even CO2 emission predictions, revenues, operating costs, characteristics. And you have all sorts of ways of displaying that-- tables, reports, plots, et cetera. So how about transit route assignment? How do we go about that? So transit route assignment is the process by which we assign origin-destination flows to specific paths on the transit network.
So there are two ways of doing that. There's an all or nothing assignment, which we talked about. That's the example of MADITUC. And then there's the multipath assignment, which we gave an example for EMME/2. When would all or nothing be OK to apply? If you have not two dense of a network and not too many alternatives, right?
Then some networks, there's only one sort of feasible, logical path that one would take for a given OD pair. But if you look at a dense network, say, London, there are often for an OD pair multiple good ways of traveling that OD fare. And therefore, that would not be a good model to use because the flows on each link might be way off. Is that understood, difference between multipath and all or nothing?
OK. Another way of dividing these or categorizing these is aggregate and disaggregate. So we also looked at these in the context of EMME/2. So aggregate is zone to zone based on zone centroids. So you have [INAUDIBLE] of transportation and ultra zones or census tracts. And you look at demand at that block level. Or you have disaggregate.
So if you load a DTFS layer, you have sort of stop level, line level, much more precise, high resolution output on where the demand would be assigned to, OK? And how do we go about more choice and sometimes how do you go about even assignment to specific lines or options within the transit mode? Often with logit mode choice model. So I think most-- well, some of you-- anyone who took 201 or is taking 202 has seeen this?
Raise your hands if you've seen what a logit mode choice model, if you're familiar with discreet choice modeling at all, have heard about it? OK. So it was something what-- I could see some shy hands that I know are more exposed to it. So in this kind of model, the-- when you specify systematic utility equations where we say for each of the alternatives that this person has, there are some variables. These variables include travel time, cost, reliability, fare, anything, right?
And the differences between the alternatives are explained in terms of those variables. And then what we get out of-- so we run a regression. It's not linear regression. It's a different kind of regression. And what we get out of that is the probability-- so we get some system-- we get some parameter estimates, beta. And we can use those to calculate the probability that an individual will take some of the options that we listed rather than the others that were listed.
So there's a very good course taught here at MIT, 1202, demand modeling. If you're interested in this topic, I recommend you take it. And it's taught in the spring only. So yeah, so this is obviously [INAUDIBLE] probability that [INAUDIBLE] you would choose one mode and not the other. Often, this is the structure that is underneath the TransCAD mode choice model or anything like that. And it's great if you understand how it works. And if you can build your own and put it in there, even better.
So all right. And what are typical variables included in these systematic utility equations for, say, the work trip? Well, it would be travel time, out of pocket travel costs, vehicle travel time, income, gender, auto availability, and occupation. So some of these are traveler characteristics which vary by person surveyed.
So typically, you will survey and you collect information on income, gender, things that vary by person. And then you also collect or put in characteristics of the choices or the options other person has. And both of these go into the model, both the decision maker characteristics and the options characteristics. And sometimes we defer those two by calling one attribute and the other characteristic.
OK, that's it for ridership prediction. But if you have questions, let's discuss. Yes?
AUDIENCE: What level of modeling is the T doing for their-- and do they change their bus network to match their modeling or is it sort of a longer-term--
PROFESSOR: The T has a model called [INAUDIBLE], which is an elasticity-based model with market segmentation. So we talked about-- that was mentioned earlier in the lecture. So they split population by type of fare product. So people with passes might-- that's a proxy for, say, frequent riders and those who might have-- those people might have different elasticities than people that are less frequent or people that pay cash or people, right? So you split people into-- you segment the market, then you look at-- it looks at previous fare increases and it calculates the elasticities for each of those market segments across several time periods and everything.
So then it calculates the total change in ridership and revenue and also any changes across would somebody now take, let's say, would some people switch to paths or not switch to paths. The Transit Hub has done some research on it. And some of the people who-- one of the persons who worked on their research here at MIT now works at CTPS. And he's one of the people who applied the model.
And then I think for other products, it depends on the product. So certainly, there is kind of the regional long term planning, which is more four-step modeling approach. If there is any specific project, I haven't seen what they've done for the green line expansion. Ari, do you know? You always know something.
AUDIENCE: I mean, I-- it's the [INAUDIBLE].
PROFESSOR: So that's the professional judgment?
AUDIENCE: That is a completely non-professional judgment. I don't--
PROFESSOR: Does anybody know what they've done for the green line extension?
AUDIENCE: I mean, I'm sure they had to do something.
PROFESSOR: Often these capital [INAUDIBLE] require demand analysis. So I'm sure they did some modeling. I'm not sure to what degree.
AUDIENCE: [INAUDIBLE]
PROFESSOR: Yeah, exactly. So whether the modeling of the FTA requires is the best one is subject to discussion. And I think a lot of transportation experts have issues with it. It tends to put a lot of weight on-- if you save many people one second, then that's great. And instead of being sort of goal-oriented, and--
AUDIENCE: Yeah, I feel like often agencies will do one analysis for their grant and then their own analysis because they think they have benefits.
PROFESSOR: Yeah. So, any other comments or questions on ridership? This is an important topic, right? It's one of the key topics in public transportation. If it's a new system, how many people will ride it? And therefore, what mode will I choose? How much will it cost, or many, many applications. Ari?
AUDIENCE: Well, I guess, maybe could you speak to how well models do? Do we have evidence that models are good, models are-- how-- what are some--
PROFESSOR: Yeah. I haven't actually seen-- I'm not very familiar with any study that systematically looked at that.
AUDIENCE: Yeah.
PROFESSOR: But again, what we have seen a lot of is the earlier approaches. And these sort of fudge factors put in, so the 50% stated preference without conjoint analysis, things like that. And those are all over the map in terms of whether they were accurate or not, often because often they overshoot the prediction. And you wonder, to what extent is that a bias because you want funding and you want to show that there will be ridership for it if it's a capital project? But I haven't really seen-- and maybe it exists-- a systematic look at recent models and how accurate they were.
AUDIENCE: You mostly see it for new systems and extensions, not for existing service changes.
PROFESSOR: Yeah.
AUDIENCE: I'm sort of interested not in comparing models to what happens in reality but what we talked about an hour ago about surveys, how stated preference surveys compared to real preference surveys, if-- has anyone done any significant research about a project that was open? What did people say before about the moment that they were going to use it? What did people then say after about what mode did I use?
PROFESSOR: Yeah. I can't site specific results because I don't remember them. But that has been looked at. And there is research on the literature on that topic.
AUDIENCE: Isn't there generally pretty bad follow-up, though, when--
PROFESSOR: Yes.
AUDIENCE: --these demand models are done, there is very poor--
PROFESSOR: But I think generally--
AUDIENCE: They don't actually predict--
PROFESSOR: Yes, that's true. So usually once you do a study, you predict the demand. If the party goes forward, nobody bothers to compare how bad it was often, at least not formally. I'm sure people comment, gee, that was way off or that was great. But do we have sort of documented studies showing how well or how accurate were these predictions? I haven't really seen many of them.
AUDIENCE: [INAUDIBLE] newspapers. And [INAUDIBLE] what we do with that? Should we require follow-up and then if--
[INTERPOSING VOICES]
--portion of the grant you got?
PROFESSOR: Yeah.
AUDIENCE: Well, and maybe it would allow us to say, OK, which models have-- where have the models worked well? Where have they not worked well?
PROFESSOR: Yeah. Yeah. Well, the idea of having a disincentive for overshooting is interesting.
AUDIENCE: The price is right. If you consistently overshoot, we'll penalize you in the future.
PROFESSOR: So that would maybe put you on a position where you want to be as accurate as possible.
AUDIENCE: But then you might be too conservative, maybe.
PROFESSOR: Yeah. It could be either way, right? You could be penalized for undershooting it.
AUDIENCE: [INAUDIBLE]
PROFESSOR: So, yeah. Any other comments or questions? All right.