Flash and JavaScript are required for this feature.
Download the video from Internet Archive.
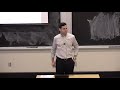
Lecture 10: Origin, Destina...
The following content is provided under a Creative Commons license. Your support will help MIT OpenCourseWare continue to offer high-quality educational resources for free. To make a donation or to view additional materials from hundreds of MIT courses, visit MIT OpenCourseWare at ocw@mit.edu.
GABRIEL SANCHEZ-MARTINEZ: I'll start today with an animation. I think most of you have seen this. Raise your hand if you haven't. So some of you have not. OK. So I'm going to just play it.
This is London. And you're going to see different colors. There's a legend right here on this corner, lower left. And blue stands for some cardholder in the London system that has not yet been [AUDIO OUT] or it's after the last time that person was seen. So it's a proxy for home, essentially.
Bright green is going to be a proxy for travel. Not a proxy, it's going to mean that this card is currently in the TFL system in a bus ride or in a train somewhere. And then red will show anything between trips that day. So that's a proxy for work. Or it could be a proxy for shopping, or restaurants, or anything between travel. So after the last trip is completed, and before the last trip ends.
So I'm just going to play this. And hopefully this will motivate the discussion, the rest of the lecture. So you can see the time at the bottom. Sorry. So you see the morning rush, and then people starting to work, so it turns red. That means most people are between trips. You see a lot of buzz in the middle of the city, middle of the day.
And then as we approach the afternoon peak, you start seeing more green activity, starting from the center, going out. And then some blue as people reach their homes and won't travel again that day. Still a lot of activity in some centers, especially in the center of London, a lot of travel still. And then past midnight, you sort of see Soho, so you know where to hang out in London. OK? OK. So before we continue, any questions about that video?
AUDIENCE: Yeah, it seemed to me that some of the dots were actually not moving along the lines. Is that deliberate?
GABRIEL SANCHEZ-MARTINEZ: So yeah. In this video--
AUDIENCE: [INAUDIBLE]
GABRIEL SANCHEZ-MARTINEZ: --there are multiple ways--
AUDIENCE: --magnitude?
GABRIEL SANCHEZ-MARTINEZ: Yeah. So there are multiple ways of generating this visualization. And the one that my colleague used to make this-- and by the way, this video was made by my colleague, Jay Gordon. You'll see in the last slide, the references to his papers and to the website with a link to the video. So yeah, for each stage-- and we'll talk about what the stage is. For each of these trips, for now, you could do it a straight line or you could really interpolate geographically along the line.
And in some aspects, the straight line one is showing isochromes almost. So it's easier to understand, visually, the OD pairs when you do it that way. But both have value. And you could look at it both ways. Any other questions about this animation visualization? OK.
AUDIENCE: [INAUDIBLE]
GABRIEL SANCHEZ-MARTINEZ: Yeah. Let's talk about how we made that. And so what goes into creating this visualization, and what data was used for it. So today's lecture is on origin destination and transfer inference. We abbreviate that ODX, O for origin, D for destination, X being more graphical, two lines crossing in the middle. So your opportunity to transfer or interchange. And if we use the British term for transfers, that would be interchange.
So what's common in all of these methods is that we're going to be basing these methods on automatically-collected data. So we're going to be using AFC, AVL, APC, instead of manual surveys. There are ways of estimating origin destination matrices in the traditional way, with manual surveys. You go out, and you distribute colored cards and collect them, and you can do this. So a little bit about that when we talk about surveys and survey planning.
Some of these methods can be used to infer destinations in open systems. So open systems are like the bus and rail, here in Boston, where you tap in, but you don't tap out. If you look at the rail system in London or in Washington DC, that's a closed system, where you tap in and tap out. And therefore, the OD pair for each trip is given in that part of the system.
It also infers transfers. So we'll talk about why this is important. One of the caveats of these methods is that they only look at the current public transportation demand. So if you want a model of all of the demand that could be there, the latent demand included, this does not look at that. So just be aware.
And also, specifically, one of the models we will look at, it can't infer destinations for every transaction. Some of that makes sense. If you see a card only one time a day, then you don't necessarily have information to infer destination or transfers. That also happens with cash transactions, which cannot be tracked using a smart card.
There's fare evasion. So some people might jump into a bus and not interact with the fare system, so we can't capture that directly. And then there's trips on other modes. So part of the logic applying to destinations and transfers will, essentially, assume that the people are mostly traveling with public transportation. And they're not going to take Uber or a long bike ride in between.
So we will look at that more in detail. Most of these methods have been validated with surveys, with good results. And they need to be scaled up because they don't make inferences for every card. If you want the full demand, you need to scale it up to the full demand. We'll talk about scaling. Questions?
AUDIENCE: [INAUDIBLE] but with London, fare evasion, that's not a big problem, right? Or is--
GABRIEL SANCHEZ-MARTINEZ: I don't know. I don't know.
AUDIENCE: All right.
GABRIEL SANCHEZ-MARTINEZ: I don't have information about that. So it could be fare evasion, or it could be-- you might have a pass and hop onto the bus, and technically, it wouldn't be fare evasion. But it is non-interaction, so that would still be counted here as fare evasion.
AUDIENCE: The more open a system, the more people will manage to evade. So I saw a number, then, the Boston commuter rail, they estimate 14%.
GABRIEL SANCHEZ-MARTINEZ: Yeah.
AUDIENCE: Yeah, that number's a lie, but yes.
GABRIEL SANCHEZ-MARTINEZ: But that's--
AUDIENCE: --a lot or--
AUDIENCE: Yeah, 70% of people pay with a pass, so it's--
GABRIEL SANCHEZ-MARTINEZ: Right. So there, they are using fare evasion more overtly. It is high, though, because the train attendants don't manage to collect tickets from everyone. And there are ways to game the system, where you can activate a ticket only if the fare inspector is approaching you. So that's a flaw in the system.
AUDIENCE: [INAUDIBLE]
GABRIEL SANCHEZ-MARTINEZ: Yeah. So more generally, when we talk about data collection systems-- we've seen the key ones, AVL, AFC, APC. I think we're all familiar with how these look. Does anybody have questions on any of these systems right now? You were looking at some data in your homework. Do you have a question?
AUDIENCE: No.
GABRIEL SANCHEZ-MARTINEZ: OK. So they can be used for many things. And so if you look at supply and demand, they both produce automatically-collected data. So on the demand side, we have the fare transactions of the AFC system. On the supply side, you have the vehicle tracking with AVL system. You have APC as well.
So they can be sent to some server or data warehouse and used for many things. It could be used for offline functions. So performance measurement is one example of that, where you want to measure reliability, running times, et cetera. It could be used for service and operations planning. And then it can also be used in real-time.
So you could use some of this information to generate customer information, which feeds back to demand. You could send alerts saying the trains are being delayed right now. Expect a longer wait. And that could, in fact, influence demand. It could make some people not take a particular trip or wait if they get it on their phone.
And then on the supply side, the information can be used to control service. So you might actually affect supply by changing the departure times. And that would affect the data that is being generated from the supply side. So there's a feedback loop.
In this lecture, we will focus on only one aspect of this framework, and that is origin destination matrices. Origin destination matrices [AUDIO OUT] one of the key inputs to service planning. They are the key demand input to service planning. You need them to figure out where people want to go. And it's just the data that expresses where people want to go. And you should try to design your system to match that demand.
So it used to be that we had to use manual surveys. They were expensive. They didn't cover all the times or places very well. And now, with all these automated data collection systems, we can infer some of the origin destination matrices from the data. And that's what we want to understand. How does it happen? What can we do? How can we do it?
So OD matrix estimation can happen at different levels. One way of looking at it is to think about route level versus network level. So route level, we're talking about one bus line. And we want to look up the trips made in the one bus line and understand where people get on and off. That's route level.
So if you have two routes here, route one, route two, we might notice or estimate that a person boards here and alights here. Some of the people doing that OD pair may, in fact, continue on route two. They might transfer to route two. So what's the drawback? Or why would it be important to know the transfer to route two and the destination on route two?
AUDIENCE: [INAUDIBLE]
GABRIEL SANCHEZ-MARTINEZ: Or is it irrelevant? Is it just cool? Or--
AUDIENCE: Maybe the destination of the route two is the real destination of the person's trips because if he goes to work, for example, the work will be at the end of the route two.
GABRIEL SANCHEZ-MARTINEZ: Right. So the real destination, the place where the person actually wants to go, might be on the destination route two, and not on the destination at route one. So this might be-- the only reason why the person is alighting there is because that's the only way-- that's sort of a function of the network of the supply you provide. So if there were a direct route from the first origin to the last destination, perhaps they would prefer that.
And for service planning, you want to know what people want to do. That's what demand is. So we want network level. So we go from unlinked trips to linked trips. That's part of what we want to do.
At the network level, we are looking at all the buses and the rail system. So again, that's what we want to do. We'll look at both kinds of OD matrix estimation in this lecture.
Let's start with one of the simpler cases. Consider a bus having APC and only APC. So APC data looks like this. You have timestamps, you have a bus ID, you have a route ID, a trip ID, some information about direction, perhaps, and then counts at each stop of how many people get on and how many people get off, boardings and alightings. And, of course, a stop ID, or a stop name, or something like that.
So you can aggregate that across trips or do it at a trip level, and count how many people get on at each stop, and how many people get off at each stop. These are called control totals in the context of scaling. So you might be aggregating across days, for example, for a 30-minute period, and count how many people are getting on at each stop and getting off at each stop.
And then what we want to estimate, knowing how many people get on and off at each stop, is the origin destination matrix. That is the cells inside of this matrix saying how many people get on at stop one and off at stop three. We're showing here, 10.
Now you may notice that the matrix here, it doesn't necessarily match the totals. So here we have 35 and 237, and the target is 40. So we have to scale it up a little bit. Here, we have 30 people getting off at stop two, and we only have 25. So that number is wrong. We need to scale up.
So what we're going to look at now is a procedure called iterative proportional fitting that estimates, given some control totals, what the origin destination matrix is. This is known as biproportional fitting or matrix scaling as well. And we start with some initial matrix or some seed matrix here in the center.
The value of that seed matrix is important. It affects the solution. So having an accurate seed matrix improves the accuracy of the final estimate. If you don't have an idea, then you could certainly initialize that seed matrix with all ones, and it will produce an output. But it may not be the best output or the most accurate result.
So it has been shown that if all the values provided in the matrix are strictly positive-- and here I am excluding what we call structural zeros, so all the cells in which people could actually be traveling. Here we are showing a route with four stops, A, B, C, D. And we have a matrix showing how many people go from A to B, from A to C, from A to D, from B to C, from B to C, and from C to D.
Those are the only possible OD pairs. Nobody is going to go from A to A because that's the same stop, so that's not a valid trip. And we're only looking at one direction, so anything below that diagonal would be in the opposite direction. And we're not including it in this example.
So what we want to do is start off with adding up each row, adding up each column, so we have total alightings and total boardings. I want them to match boardings, and match target alightings, or the control totals. Questions?
AUDIENCE: What are the control totals again?
GABRIEL SANCHEZ-MARTINEZ: They're counts of boardings and counts of alightings at each stop. And they can, in this example, come from APC.
AUDIENCE: That's what come from APC.
GABRIEL SANCHEZ-MARTINEZ: So what we do, the algorithm for iterative proportional fitting, it calculates a scaling factor for each row. We start with rows. And we say, well we need to scale up everything on the first row by 40 over 3, so that that number adds up to 40. And you calculate the scaling factor for each row and apply it to the cells in the matrix. And of course, the sum of cells column-wise is not going to add up to the target alightings.
So the second step is to apply the same procedure on the columns. And now we realize, well, we need to apply a scaling factor of 30 over 13.3 to get B to add up to 30. And you do the same for each column. And now the columns sum up perfectly, but the rows don't anymore. So now we go back and repeat the process. And we go to the rows and the columns.
I've put it in all the slides so you can actually repeat this in your spreadsheet program, if you want. It has been shown that if all the non-structural values of the cells in that matrix are not zero, and then they are positive, then it will converge. And it will converge to the maximum likelihood estimate, of the best possible estimate, given your seed and given your control totals.
So you can apply this if you ever have a situation where you have control totals, but not the origin destination matrix. And that's one example of-- that would be having APC and nothing else. Any questions on this method? We're to see it again in a different application later in this lecture.
AUDIENCE: Is it guaranteed to converge to the correct value?
GABRIEL SANCHEZ-MARTINEZ: Well, what is correct? It may not be the truth, if that's what you mean by correct. So it's the best estimate of the truth, given the information provided.
AUDIENCE: How might it converge to something that isn't correct?
GABRIEL SANCHEZ-MARTINEZ: Your seed matrix might be wrong. Or there might be aggregation errors, for example. So if you start with all ones, that's clearly not true. And this is the best possible estimate, given your starting assumption that all of the pairs are equally likely, and then adjusting from there, right?
And then I mentioned structural zeros. So if you have a non-structural zero, say that with onboard survey, you collected OD demand only for a couple of trips and used that to seed the matrix. And let's say that first some of these OD pairs, you didn't observe a single person taking that trip. So you say, well, in the seed, I have a zero, but that's a non-structural zero because-- it is a structural zero, rather.
So this value, some people might be taking the OD pair. And if you seed it a zero, then you can't scale it up above zero. So in this case, you would not converge necessarily. And you certainly would not converge to the maximum likelihood estimate. OK?
AUDIENCE: I was going to ask how you get a better seed matrix, but you--
GABRIEL SANCHEZ-MARTINEZ: So with any kind-- we'll talk about other methods for ODX, so for estimating origin destination matrices. Manual surveys is one example. Any knowledge that you have about what OD pairs are busiest should help. So you could do on-off counts with the traditional way if the only thing you have is this. And we've talked about surveys extensively.
AUDIENCE: So just to [INAUDIBLE], APC is the target boarding [INAUDIBLE].
GABRIEL SANCHEZ-MARTINEZ: Yeah. So over some number of trips-- and this is a toy example, clearly. Over some number of trips, you counted 40 people boarding at A, and 30 people alighting at B, and so forth. So you want the cells to match that. OK?
So you could do this in Excel, or you could write your own little function to do this. It amplifies errors in the seed matrix. You're scaling up, so if you have errors in your seed matrix, they will be scaled up too. Just be aware of that.
So what about if we don't have APC? What if we only have a AFC and AVL? So now we don't have control tools. AFC might give you boardings, but not alightings. So what are the ways of scaling up with that?
You have different systems, and it depends on the system. So if you look at TFL in London, we said AFC there is closed, so the origin station pairs are given by the rail system because people have to tap in and tap out. On bus, however, people only tap in. So there, you would have to apply this inference method.
Here in the MBTA, both bus and rail are open. You tap in. You don't tap out. So we have to infer destinations in rail and in bus. And then in some more advanced systems, a lot of information, including transfer information, is given. Seoul is one example of that.
AUDIENCE: How is that different? They--
AUDIENCE: They tap in [INAUDIBLE].
GABRIEL SANCHEZ-MARTINEZ: They tap in between-- yeah. So that there's an interchange tap.
AUDIENCE: They actually have to tap out to leave--
GABRIEL SANCHEZ-MARTINEZ: And by the way, in some parts of London's network, that is true. You tap to prove that you were transferring. There might be a fare advantage to doing that. So control totals.
So here in Boston, with the MBTA buses, some portion of buses have APC, but not all of them. So you could use the first method applied to only a fraction of vehicles and then scale up to all vehicles. That's one possibility. Or you need something else.
In London, they don't have APC, at least not widespread. And they do have the ticketing machine. So in theory, drivers are supposed to push a button if somebody boards, and they don't tap. Do they actually do it? Not clear to what extent the drivers comply with that instruction.
And then gates and rail gates. So tapping in or out of the subway system there, there's a counter. So it counts people passing through. So if somebody goes in through a gate and out some other place, and we don't know exactly what they did, the total number of people at each node in the system can be counted.
And we can use that information to scale up. So we'll talk about that later. So it depends on the context.
Let's start with origin inference, the first letter in ODX is origin inference. So we're looking at a bus, which has one stop and then another stop. And if we match the AFC transaction times to the AVL stop visit times, we can put them on the same timeline and realize, well, there was a tap right after that AVL system said that the bus left that stop. It's very close, however, to that stop. So let's assume that the tap-- maybe the bus pulled out and started moving, and the person was finding the card.
But you still tap-- it if it's close enough, let's assign it to that stop.
AUDIENCE: Just a second, are there systems where the AFC is connected to the AVL directly?
[INTERPOSING VOICES]
GABRIEL SANCHEZ-MARTINEZ: Are there systems where the AFC connected to the AVL? Yes. In London, they do that now.
[INTERPOSING VOICES]
GABRIEL SANCHEZ-MARTINEZ: They didn't when we started with this, but they do it now.
AUDIENCE: Because I know from the process that you and Neema wrote for Chicago, where you had to connect the AFC to the AVL, it was a headache. But it seems to me like it shouldn't be that way.
GABRIEL SANCHEZ-MARTINEZ: Well, remember these systems--
AUDIENCE: It should have an AV feeder into AFC.
GABRIEL SANCHEZ-MARTINEZ: So it's starting to move that way, but none of these systems were put in to capture data. None of them. APC is the only one, actually. So APC was put in to collect data and not have to do all these surveys because that's expensive.
But AFC was put in to collect fares, and avoid theft of fare revenue, and simplify the duties of drivers, improve safety. So there are many advantages to it. Smart cards have the advantage of having passes and all these things, so many advantages to an AFC system.
AVL was for safety. If there was an emergency on the bus, the driver could hit a button, and the police and the ambulances could be dispatched to the location of the bus. That's why it was started. Later on, it started being used for management as well. Data collection of how many miles of service you provided, which is a requirement for the NDT reporting. So aggregate level reporting.
But none of these systems were put in thinking, oh, we're going to estimate origin destination matrices with them. So that's something that has come after the fact. Now that people are thinking about that, yes. We start seeing, can we hook up these two systems, which might be from different vendors, and make them talk to each other? So London does that now.
So if you see some tap that is very far away, in time, from any stop, you might not be able to infer the origin. If it's close or between the reported arrival and departure, we match that transaction to that origin. Simple, right? So in London, we did that. This is Jay Gordon's thesis, which is referenced in the last slide.
Looking at 10 weekdays. Oyster is the AFC system in London. And so 96% of boarding locations were inferred within plus or minus five minutes. And that was one of the thresholds they looked at. 28% were exactly between the reported arrival and departure. So some tolerance before the arrival and after the departure from each stop was needed to infer a large portion of these. All right? Simple.
Destinations, that's the next step. So we have origins. It's a rail system, you tap in. It just tells you I'm gate number blah, and that is at some station. If it's a bus, you can join AVL with AFC, and you get it. Now let's look at destinations. So there are different methods for inferring destinations. [AUDIO OUT] AFC and AVL. And one of the simplest methods, or the family of methods, is the closest stop assumption.
So what are the key assumptions? We start by saying that the destination of each trip segment is close to the origin of the following trip segment. So in other words, that is true, physically, right? You have to move somehow through space. So we, further, now assume that that movement is happening mostly through the public transportation network, and that no trips on other modes are being made.
So if you go from home to work in the morning, and you have to then-- say that you work, and then at the end of the workday, you go back home. We see that your next origin is the stop across the street from where you got off, hopefully. So we'll look at which stop was closest on the trip you boarded to the next origin. And we'll infer that is the destination.
If it's a rail system-- that's what we show here in blue. So we have an origin on this bus line. We want to infer which of the downstream stops is a destination. And then we look at the next trip, and the next trip started at T, the target. And we want to get as close as possible to the target. So we'll say that the destination is D, if the distance between the D and T is small enough.
Because if it's three kilometers, we might say we have no clue. This person may have moved with a different mode. And therefore, this assumption of closest stop may not apply in that case. So in those few cases, we won't make an inference of destination. If it's rail, so two rail lines, and you may be able to change between lines behind the gate, then closest stop is the same station that you next enter because that's the closest.
So if it's a bus-- so you may have boarded the Red Line here and somehow gotten to the Blue Line. We don't know that yet, but we observe that the next tap is at a bus, then we find which station on the rail network is closest to that bus stop. And that's the inference. That's destination. So this is the simplest method for destination inference.
Any questions with the closest stop rule and that inference method? Here's an example of one card with four trips. It's a time-space diagram of sorts. So we start the day here in the morning, and we maybe observe a boarding at this line.
This person, in reality, transferred to the second trip. So we don't know that at first, but we do see the origin and the second trip. So we find which of these stops was closest to that origin and, we say that's destination.
And likewise, from the trip leading to work, and from the trip returning from work-- work, school, whatever it is-- we find the closest one, and we say that's destination. And we just keep doing that. What happens at the end of the day? There's no next one, right? So what do we do?
AUDIENCE: If a person gets on a bus that does go to where they started, if the last bus-- let's talk about bus for a second. If it leads back to where they started, then we can assume that they--
GABRIEL SANCHEZ-MARTINEZ: Right. So that's the key assumption. They key assumption is that the person returns to the first place seen that day. Another option is to look at the AFC system and see what is the next place of origin the next day, if you have that information. Both things are possible. OK?
AUDIENCE: But if they get on the last bus of the day, does not [INAUDIBLE].
GABRIEL SANCHEZ-MARTINEZ: Well then you can't make an inference. So if they get on-- the question was, what happens if they get on, say, a bus. And none of the downstream stops of the origin get close to the first origin of that day or the first origin of the next day, then we can't make an inference. So we leave that destination uninferred for now.
All right? So there are some tests. We talked about distance. Time is another one. So there's different ways of looking at this. In London, when Jay Gordon did this, he got an entrance rate of about 75%.
Here's a distribution of speed between station exit and inferred bus alighting or subsequent station entry. So very slow speeds here. This goes up way higher than 800. What does that show? This is meters per hour, so if you move zero or, say, one meter per hour, what does that imply?
AUDIENCE: Someone was taking a bus?
GABRIEL SANCHEZ-MARTINEZ: Who are those people? Emily?
AUDIENCE: People who get off at, say, a tube stop and then go to work--
GABRIEL SANCHEZ-MARTINEZ: Go to work for eight hours.
AUDIENCE: --for eight hours. And then--
[INTERPOSING VOICES]
GABRIEL SANCHEZ-MARTINEZ: And then next boarding is right across the street, 8 hours later. So those are people who are between trips. And then to the right, here, we have something sort of bell-shaped, with some distribution, quite wide. These are in the range of walking speeds. So it checks with what we know, and what we infer.
Here is a distribution of distance between subsequent tap and closest stop on the current route. So how far away that you walk to the target from your destination, in other words. And there's a cutoff. If this were too far, we would not want to make an inference. But you can see that most people have quite short distances.
So that's good. That means that our inference is more likely to be true. OK? And you have some details here. So in the case of London, there was a comparison of the origins and destinations produced by this algorithm, with the bus OD survey, which is a manual survey. And it compared favorably.
One thing with BODS, of course, it had the biases that a manual survey has. So it seems that BODS underestimated ridership during the peak periods, where it was maybe harder to count. Sometimes the BODS return rates were low.
We saw some of the reasons for biases in manual surveys. If it's a very full bus, people are less likely to return a survey. Or if you are a person who is getting off at the next stop, you are less likely to answer the survey.
So BODS was, of course, subject to those biases. And essentially, the people who were doing this validation were happy with the inference method. Now what happens in rail in Boston, say? It's an open system. So you can apply the nearest node method.
But you have a complication in Boston, the Green Line. So the Green Line is-- if you take it in the branches, you board, and then you tap into the vehicle. So it looks like a bus from the fare standpoint. And then you could end up anywhere on the rail network.
So it's a little harder to make an inference of where you get off in that case, especially going back. Going back to the branch, it's not clear. Besides that, there are some other reasons to try more sophisticated destination entrance methods.
We know that it may not always be the case that the nearest station in the rail system is actually the alighting station. There are some cases where you wouldn't take an extra 15 minutes to get a little bit closer. And you are willing to walk and make a compromise between those two.
So the minimum cost path method is an improvement over the closest stop method. What we do there is we look at-- here's the origin tap location, the entry to the system. And we, essentially, explore using a minimum cost formulation, a dynamic programming approach. All the feasible paths that the person could take to their next tap location, the target. And that includes walking links from any possible exit station.
So we then use a generalized cost equation to assign a cost to each of these paths, with relative disutility weights on each component. So waiting is more than vehicle time. Walking is more than waiting time. We've seen these equations before. And now we have a list of paths, and we assume that the person took the one that minimized their disutility. Their combined, generalized disutility-- avoiding walking, in-vehicle time transfers, all those things.
So in this case, perhaps the person prefers to get off of the Red Line and walk to the next location. You could think of this as the Red Line from Kendall to Park Street. And then the next entry is at, say, a bus stop close to Boylston Street.
You could transfer to the Green Line and take it one stop, or you might decide it's a nice walk. I'm going to walk. I'm not going to wait for the Green Line. And then some possible paths take you way far from your next location, so they are pruned. They're not included.
So what happens if we compare the two methods? What's your intuition? Or what do you think happens if we compare the results of nearest node with this more sophisticated method? What percentage of destinations do you think will be inferred at a different place? Is it close to 5? Close to 50? Close to 25? 10%?
AUDIENCE: 5%.
GABRIEL SANCHEZ-MARTINEZ: 5%?
AUDIENCE: What percent of the destinations will be inferred or will not be inferred?
GABRIEL SANCHEZ-MARTINEZ: Will be inferred differently.
AUDIENCE: Oh, will be inferred differently. 5%.
GABRIEL SANCHEZ-MARTINEZ: Five? OK. So that's actually close. I actually don't think I wrote the results, which is good. So let's look at two examples. It is close to 5%, in fact. Some of the differences in the Boston network are clear improvements in the accuracy.
I'll give you one example of that. Some people go from Forest Hills, and then their next tap is at Copley. So the walk between Back Bay and Copley is five minutes, and it's a nice walk. If you use nearest node, you have to remain on the rail line. And you have to transfer either at Downtown Crossing to the Red and then at Park Street to the Green or go to Haymarket and transfer it to the Orange Line. That's a 20-minute, 25-minute ordeal.
AUDIENCE: For a walk from Downtown across to Park Street, that's--
AUDIENCE: Sure, but then you are not following the method.
AUDIENCE: Oh, you don't [INAUDIBLE] transfer?
GABRIEL SANCHEZ-MARTINEZ: Yeah. So this is a case where the minimum cost approach says, yeah, you get off the Back Bay, and you walk. So yes, it's an improvement. There are some other cases--
AUDIENCE: What was the person destination?
GABRIEL SANCHEZ-MARTINEZ: Well, we don't know destination. We're inferring destination.
[INTERPOSING VOICES]
GABRIEL SANCHEZ-MARTINEZ: And what we know is that they get on at Copley the next time.
AUDIENCE: Yeah, but was that their afternoon trip? Or was that their--
GABRIEL SANCHEZ-MARTINEZ: It's a morning trip. They go from Forest Hills.
AUDIENCE: So both Forest Hills and Copley were morning taps?
GABRIEL SANCHEZ-MARTINEZ: Oh, I don't know. Copley might have been the afternoon tap.
AUDIENCE: It doesn't really matter if it was in the afternoon.
AUDIENCE: [INAUDIBLE]. But then why would a person get on--
AUDIENCE: The question is where do they get off the Orange Line.
GABRIEL SANCHEZ-MARTINEZ: Right. We're trying to infer the destination when they board the Orange Line in the morning.
AUDIENCE: But the time gap also matters. Forest Hills--
GABRIEL SANCHEZ-MARTINEZ: We are making an assumption that people [AUDIO OUT] too far from their--
AUDIENCE: But I--
GABRIEL SANCHEZ-MARTINEZ: --destination in a non-public transportation mode.
AUDIENCE: Sure.
GABRIEL SANCHEZ-MARTINEZ: That's one of the assumptions in this method and in the previous method as well.
AUDIENCE: But I'm still troubled by the time gap. Was Copley and afternoon tap? Or was it--
GABRIEL SANCHEZ-MARTINEZ: It could have been. There are many people who do this.
AUDIENCE: It makes a difference--
GABRIEL SANCHEZ-MARTINEZ: So there are many people who do this. So there are some people who do it close in time and some people who do it in the evening, after they leave work. So I'm giving you an example of one origin target pair. And I would say it's a marked improvement. It's certainly not the case that the person goes all the way to Haymarket and turns around. OK.
The other example is less clear. So somebody-- an OD pair starting at Maverick, and then the next tap is at Downtown Crossing. So obviously, the closest node assumption is that you transfer at State Street and take the Orange Line one stop. That's actually not too bad.
The algorithm-- and first, for a lot of these people, that you get off at State Street, and you walk about four minutes, and if you look at Google directions, Google will say that's what you should do. The transfer to the Orange Line would take six minutes, instead of four. So it's very close.
And it depends on the weather that day. And it depends on people's preference. It might depend on real-time information about whether the train is right here, and I can run, or arriving in one minute, or if it's 10 minutes away. So this is more subtle, more nuanced. And I wouldn't say that was an improvement.
So part of the 5% is clear improvement, and another part of it is, well, it might be an improvement or not. It depends on people's preferences. So if we look at the distribution of the results from destination in this case, 70% of destinations were inferred. And then we have different reasons why we can't infer it.
So for 16%, there was no target location. That means there was no other tap that day, essentially. So there was no target. For 8% of them, there was another target, but it was very far. So somehow, the person went to another bus stop in the system, and it was far away from any rail station.
So we're not so comfortable, in that case, saying that the destination is close to the next tap. So we will not make an inference for those people. Some paths were non-feasible. So that means that the algorithm did not find any path that made it to the target on time to make the next.
So that could be about data. It could be a number of things. There are some assumptions about how quickly people can access trains and hop. So many things can go into that.
Yeah, and so forth. And then unknown origin, so errors in the data, et cetera. OK? And the inference probabilities, the total ones are shown here.
So the blue line is overall destination entrance rates, so I said close to 70%. That's what you see on the blue line. It dips a little bit on weekends because there are fewer taps and maybe more walking between taps or between trips.
For rail, it's a little higher than the general. For bus, shown in yellow, it's a little lower. And if you take away the part that didn't have a second tap or a tap after that the transaction, then it goes up to closer to 90%, not quite 90%.
AUDIENCE: [INAUDIBLE]
GABRIEL SANCHEZ-MARTINEZ: Yeah?
AUDIENCE: Does this include only people who tapped? Or does this also include people who paid cash?
GABRIEL SANCHEZ-MARTINEZ: So these numbers in this slide are everyone. If the person is cash, then they are not counted on the red line because they wouldn't have an inferable destination. But certainly, the bus line does include cash transactions.
[INTERPOSING VOICES]
GABRIEL SANCHEZ-MARTINEZ: And that's one of the reasons why it's lower.
AUDIENCE: [INAUDIBLE] target location on the last slide includes cash transactions?
GABRIEL SANCHEZ-MARTINEZ: So this was only for the rail. Sorry, this is for the whole system, but the two examples that I gave here, I was looking at-- I quoted 5% difference, and that's a case study where I compared rail transactions, not bus transactions. So that's the one thing to have in mind. But yeah, this is overall destination inference in the MBTA, and this as well. Different ways of looking at it.
AUDIENCE: So if you paid cash, you'd be in the no target range.
GABRIEL SANCHEZ-MARTINEZ: Yes. And you could infer an origin for that person, but not a destination, so you leave that trip uninferred destination for now. OK. We've covered O and D. Let's move to X, transfer inference. We talked about why transfer inference is so important.
We also call this interchange inference. Interchange is a term preferred in London by the British. In the US, we say transfer. So we have seen this diagram before. But now there are these blue boxes surrounding both, say, the morning pair and the afternoon pair.
So the inference we want to make now is whether this first trip was connected to the second with a transfer. Or whether, in fact, the person was doing something else in between those two trips, and this was the actual intended destination of that passenger. And that's an important question for the reasons we talked about earlier.
These are some definitions for your reference. A journey, in this subject, is everything that is accomplished from the real origin to the real destination of the person, including transfers and, possibly, multiple fare payments. A fare stage, not included in the slide, is everything that you do in a single fare payment. So it could involve behind-the-gate transfers, or it could be one bus ride.
Transfers are transfers between stages. So they link segments of a journey. How do we do this linking? This is also from Jay Gordon's thesis, which is referenced in the back. We look at a series of three kinds of conditions-- temporal conditions, logical conditions, and spatial conditions.
Temporal conditions, say, how much time happened between the inferred destination and the next origin, the inferred origin. If that was a very long time, and the distance was short, then the person might have been doing something else. So we can't necessarily assume that this was a transfer.
We also look at bus wait time. So what if the distance was short? A long time happened [AUDIO OUT] of next bus or every 20 minutes, and the person had to wait that long? Well, that is also considered. So if we look and see that that next bus passed after a reasonable time allowed to get to the next stop. Or how many buses passed? Maybe you want to allow one, just in case that bus is very full and can't take that person.
So these are the considerations in temporal conditions. Spatial conditions, you want to look at maximum interchange distance, assuming that a person can actually do a transfer that is two kilometers long, for example. You probably would be doing something else, if that's the case. And we look at circuity, so circuity at the journey level and between stages. A circuitous journey is one that ends very close to where you started.
So if you infer transfers, and you end up back where you started, then somewhere in that chain of stages, there must have not been a real transfer. There must have been a non-transportation activity. So therefore, you can't really infer that all of that chain is linked with transfers.
And then circuity between stages. So if you, for example, board the same bus line going backwards, even if the time was short, and the distance was short, you may have seen your friend and given your friend something, and then hopped on the bus again. So it might have been a quick transfer. And therefore, we want to look at circuity to infer if that was a transfer or not.
Logical conditions, I actually gave an example of that right now. So if you're entering the same station you get off at, or you take the same bus line, then that shouldn't be a transfer because you could have stayed on the same bus. One example of that breaking would be a bus being taken out of service or something like this.
So you could consider not to make that logical condition more accurate. In many cases when that happens, though, people are not asked to tap again. So take that with a grain of salt.
Questions about these assumptions and the tests that we impose? So essentially, if all of these tests pass, we say, yes, this is a transfer. If one of them doesn't pass, we say, we're not sure. It could have been or maybe not. And therefore, this will be a conservative assumption about whether these two stages are linked as one journey.
Here is in London, the results. So about 2/3 were one stage, about 1/4 were two stages, and then about 10% were more than two stages. And here's a distribution of duration of journey from first origin to last destination, instead of the unlinked trip time. This includes transfer time in between. So very powerful for service planning.
There was a comparison with the London travel diary survey, little tedious. And it lined up quite well, but there were some differences. So if we look at people reporting that they only took one journey on the day they were queried about, it lined up very well.
If you then look at two or more, it turns out that a lot of people in LTDS say that they took two, but they may have taken more. And they're just simplifying the reporting. That's one possibility of errors. And it is a known bias in surveys that people to try to help you out by saying what I usually do, instead of what I did yesterday, or things like that. So percent of cardholders, shown on the second graph, and the number of stages per journey, similar pattern.
So in LTDS, you tend to have fewer people. Well, yeah, here we have more people inferred with one stage, so no transfer. But if you look at two or more, a similar pattern emerges where people might be reporting two and, in fact, it might have been more or less. So in this case, the bias is towards more direct trips on the inferred side, versus the questionnaire side. OK?
So it didn't validate perfectly. But there were some known issues with LTDS. London has since decided that ODX is more accurate. Now they continue LTDS because LTDS is useful for other things, like asking about social demographics, and trip purpose, and things like that. That doesn't obviate the need for LTDS, but it might reduce the need to have as many LTDS surveys.
Scaling. So we've done ODX, and we've inferred a percentage of destinations-- or we've inferred destinations for a percentage of transactions, not all of them. And we've linked up the ones that we could. Now, we want the full matrix, because for planning, we want to know how many people want to go from here to there.
So there are different methods for scaling. We have different situations. So AFC, AVL, and ODX, together, given an OD matrix, but it's only for a sample of passenger trips. If you have APC, that gives you the full boarding count [AUDIO OUT].
So if you have that for all your bus fleet, then great. You can use IPF. And you could apply your ODX matrix as the seed to make it more accurate. That's great. In some cases, you only have APC on a fraction of vehicles or on no vehicles. And therefore, that's a little tougher.
So IPF can be applied in this context, not just on the whole matrix, but also on the part of the matrix that is not inferred. So you can, essentially, subtract from your control totals the portion of the demand that was inferred, and apply IPF only on the remainder. And that will scale up only that part.
OK? That's better if you don't want to distort your seed too much, essentially. If you're not very comfortable assuming that all the people that are not inferred have the same demand OD structure as the people that you do have an inference for, then separating those out and using IPF on the uninferred portion will give you a more accurate result because you're not amplifying whatever you observed and was able to infer.
So one example of that is transfers. And we'll give an example of that in the next few slides, actually, right here. So consider scaling when you have transfer information from ODX, and you don't have APC on every bus. You have it on some buses, but not every bus.
So the real complete OD matrix is what you want. And we could split it. I'm using algebraic notation here. The real matrix, R, can be split into the inferred portion and the missing portion, M.
And the missing portion-- there's two reasons for missing data. One of them is, I saw the boarding, but I couldn't infer destination. So that's U, the uninferred portion. And then there's the N, the non-interaction part. Those are the people that board without interacting with the fare box.
We want all of R. We know I, or at least we made an inference for I. And then we want to estimate U and estimate N. And then we can add those two estimates together to the I, and we'll have the estimate of R. And that's what we want.
That's what scaling accomplishes. Now there's one critical observation to make here. If you take a trip on a bus line, and then you transferred somewhere else, there will be a tap close to your destination, shortly after your destination. So the likelihood that you were able to make a destination inference is very high Do you agree with that? Yes or no?
AUDIENCE: [INAUDIBLE]
GABRIEL SANCHEZ-MARTINEZ: If you have some bus line, and let's say that at stop B, there is a rail station. And you are taking the bus line from D, C, to B. If you are actually going to transfer to this transfer station, then you will have a tap onto x, shortly after you get off at B. Right? OK.
So given that I inferred your origin being D, the probability that I actually infer that your distinction was B is very high because I have the information to make that inference. It's close in time and in distance. It will pass the checks. So if we make the assumption that in every case where we have a transfer, we've successfully inferred the destination, then we have to then say, well, then none of the people who are uninferred had a transfer afterwards.
Right? Right? OK. And what happens with-- what if this is a very popular rail station, and a lot of people take it? Then the uninferred portion of the demand are people who don't transfer there. And if you applied the ODX matrix of the people that you had a destination inference for, you would be weighing B as the destination too much.
Those people are not very likely transferring to-- some people might be getting off at B, but fewer of them because you're looking at the people who don't end up transferring. So it could be people that go somewhere else around B, but the percentage will be lower.
So what we want to do is produce destination probability matrix from the portion of I that we inferred that was not followed by a transfer. So we prepare a different matrix, excluding the people that transferred after this trip. And then we use that to scale up the remaining origins in probability. So that's what we have here, expressed mathematically.
U is the vector of boarding locations with uninferred destinations. And we multiply it times L bar, where L bar is a matrix of destination probabilities of trips not followed by transfers. So that comes from ODX, But we remove trips followed by transfers. All right?
And then we have to take care of the non-interaction trips or not observed trips. Some of them are trips with uninferred origins. So it could be that we know that this person was at this trip because the origin inference failed, or it could be that the person-- there's some information that, in general, some portion of passengers don't interact. So you want to scale everything up by 5%, say, as an example.
That could come from surveys or from APC, if you have APC on a portion of the fleet. So essentially, n, here, is the scaling factor. It could be bump what you have so far, which is I plus U, by some amount, some percentage. That's the simple way of doing it.
n could be a vector. So you could have different scaling factors for each boarding stop. It could be a 5% overall, or it could be there's a lot of non-interaction at this stop, but not this stuff stop. It could be correlated to loads. So many things could happen. You can scale up this way.
And now we have everything together. So this is just combining the terms. This is the scaling factor for not observed, so if n is a flat 5%, this is 1.05. And then I is what you had from ODX. And uL is the application of the destinations improbability to the people that had origins, but not inferred destinations. OK?
Questions about this method, the scaling method? This for one trip, or for bus trips together, say. It's not journey-level scaling. So let's move on to journey-level scaling. So now we're getting a little more complicated.
So we have journeys, which include full itineraries of people boarding at one location, or entering a station in one location, doing several trips, including transfers, possibly. And each of those is considered an itinerary. An itinerary could be one stage, or could be multiple stages linked together with transfers.
So again, we have inferred itineraries for a portion of the demand. But we want to scale up the demand, knowing control totals, but at the itinerary level, because we have information about itineraries. So why not do it that way? It could be more accurate. So it's challenging because there are many possibilities. And some places that people go through don't have good control totals.
But essentially, we can follow an approach that is, in essence, IPF but applied not to boardings and alightings, but to the scaling factors themselves. So this is a toy example. Here's a rail line where there's tap in and tap out. Here's a bus line where there's only tap in.
So there are many possible itineraries that a person could have here. Going from A to B, transfer to D, alight at E, that's one itinerary. Go from A to C, through B, that's another itinerary. And at each of the nodes here, A, B, C, D, E, there might be some counts. So on D and E, we only have on counts because people are not tapping off. On A, B, and C, we have on and off, or entry and exit, counts.
That means that there are these count nodes, A in, B out, as two examples, where we count how many people go through that place. And we know from-- we have, for a portion of the people, this ODX sample here, some number of itineraries that go through A. So this is A in. So we have some people going-- a portion of those people are inferred to have gone from A to B, a portion to ABC, a portion on the itinerary ABDE.
And then there's some portion of it, shown here as delta A in, who are included in the counts, but we don't have an inference of their itinerary. OK? So what we want to do is scale up the mixture of ODX here to make up the total entry count. But there's a catch.
These itineraries are affecting counts elsewhere on the network. So we also have B out, as one example. And we know that the people on TAB also show up on the count on B, not the people going ABC, though. Those are not included in the count of B out because they don't exit at B. And there are some new itineraries showing up at B out that are not in A in.
So we want to somehow match the counts at all the places that are affected, or that are showing up, or associated with each itinerary, and scale the demand so that the control totals are satisfied at all locations. So the method is similar to IPF. We prepare a binary location itinerary incidence matrix with zeros and ones, associating each itinerary with the count nodes.
So A in, well, AB is one itinerary that is shown there. So is ABC, ABDE, not CB, not CBDE, not DE, as an example. So we have this big matrix of zeros and ones. And we have two equations.
Ti is the total scaled up itinerary demand on itinerary i. And we know that that total is going to be 1 plus the scaling factor, or the scaling factor is 1 plus alpha, really. Alpha is the portion over 1 that we want to scale by times the observed or inferred flow on that itinerary i, which is t.
Then we also have this other relationship that the remaining portion of the count on a node, which is the count on node n, the control total on node n, minus the portion that was seen through that place is the amount-- it adds up to the sum of all itineraries going through that place times their scaling factors.
So now we need to figure out what the scaling factors are for each itinerary, satisfying both of these equations. We have two equations on vectors, we have it on control nodes, and we also have it on itineraries. So we're back to the same situation. We can do IPF on itineraries and count nodes. And that's what we do. If we have better data, we could initialized with a good seed matrix, otherwise you could initialized to 1.
We then update the estimated count nodes, which is delta. Again, delta here is the difference between the count of flow through that node and the observed flow. So it's the part that you have to scale up to. And you do that for all nodes.
And then you-- oh, let's look at what happens here. So when you apply this equation, you calculate a delta hat right here. That is much higher, it looks like. Yeah, it's much higher than the actual measured delta.
So you know that that initial scaling is producing demand flow that is too high through those nodes. And that's because we said that alpha was 1 for all of those nodes. But it isn't, so those alphas need to be adjusted now. So we moved to alphas. And we update alphas by looking at, essentially, the average scaling factor required across these control deltas that apply to each itinerary. Not all of them apply to each itinerary.
So for itinerary AB, you would take the average required adjustment factor across all the deltas that apply to AB, which are only the first two. You wouldn't include the last three because they don't touch AB. So you calculate the average, and you adjust the alphas.
But now you're not getting the demand that you expected, so you have to go back, and you cycle through again. And you go back and forth, back and forth. You apply these two equations until you converge. And convergence in this case means that the delta hats will match the deltas that you measured, and that the alpha values are not changing much between iterations. OK?
So we haven't seen the proof that this converges. It relies on an average here. So it's an introduction or a new aspect of the method. But in every test that we've run, it does converge. On a toy example, where we didn't know what the actual journeys were, because we produced the real data, and then we hit it, and scaled it up, we started with different required scaling factors by itinerary.
So in the blue line case, this blue line right here, the scaling factors required across itineraries were very similar to each other. They all needed to be scaled up by the same amount. And what we show here is that the solution converged very quickly, and that the accuracy was high, because the root mean squared error was low. OK?
But then as we start moving to differences in scaling factors required, the algorithm did converge and produced different alphas for each itinerary. But it took longer to converge, and the root mean square of the final solution was higher, which makes sense. All these methods, the IPF founding methods amplify errors. They amplify whatever you give it at the beginning. So if you start with something all 1's and in fact, they are quite different from 1's or some of them are 1, and others are not, then you're going to have a bigger error in the final solution.
So this was applied to London once again. In practice, there is another complication. People who are counted at each node, they don't all finish their journeys, or they don't all start their journeys in the time band that you are including the counts in. So if you do a trip that takes a whole hour, you might be seeing tapping in here, and then you might end up tapping out at the next hour.
So you need control totals, in this case, by the hour. We were looking at hour scaling, scaling of demand every hour. But you need to adjust the control totals to get what percentage of people who are tapping out at this location actually started their journey in that hour. How many of them actually started on the hour before?
So there was an offset correction, and this is what is being shown here. Here you have raw entries and raw exits in dashed lines. And the correction essentially shifted those entries backwards in time a little bit, so that the control totals matched. And then you can run the journey scaling as we just showed.
And the results, we don't have ground truth data in this case. You could run a survey. I guess LTDS, in some ways, is ground truth, but it's a low sample. The overall scaling required was about 50%. You can see the 3/2 line here.
One way of validating it was to run it only on rail. Because rail has OND, we can run IPF on the rail matrix, because you have the control totals for all the gates in and out. So there's no complication of the bus. You can run IPF on that. And it aligned very well with the more sophisticated solution of running this by proportional fitting on the itinerary scaling factors, instead of the simpler IPF method.
Presumably, the errors that you see here that are slightly off the diagonal are improvements in accuracy. Because instead of starting from, say, all 1's you are using good information about a seed matrix from ODX. So the accuracy should have been improving. We don't have, again, the ground truth to assert that measure, how close are we about to reality.
And one application of this, here's a chart showing all the origins. So it's like a heat map of London. And a darker color of red shading each cell shows a higher proportion of people originating at that location and going to Oxford Circus, which is right here in the middle.
So for a planner, looking at this and knowing if I want to know where people are coming from to Oxford circle, here's a map. And you can do is by time band, so only for the AM peak or-- there's many applications of this origin destination data. I'm just showing you one here.
And here are some references, so Jay Gordon's thesis, a paper he wrote. The Southwick reference is for the scaling of buses without the transfer demand. So if you want to read more about that, that's the write up. And then I wrote a paper on the inference of destinations using dynamic programming, instead of closest node. That's also published. You can get that.
And then finally, Jay's website has the this visualizations for London, and Boston, and yeah. So you can have fun looking at that. All right. Do we have any questions about this? We can watch the animations again. And maybe now we really know-- now we really appreciate what went into it.
AUDIENCE: So I know there's a number of old systems [AUDIO OUT] a lot of newer systems are just doing proof of payment. So in that case, they only can use--
GABRIEL SANCHEZ-MARTINEZ: So some new systems are proof of payment as well.
AUDIENCE: Right. And in that case, they can only use APC to--
GABRIEL SANCHEZ-MARTINEZ: So right. You can have APC. APC has some issues, especially when the vehicle is crowded. Some people block APC sensors. So you could have-- if it's proof of payment, manual surveys is the other alternative. Yeah. And proof of payment is something we can debate. It has benefits and [AUDIO OUT]. So on the data collections side, that's a clear disadvantage of proof of payment. Yeah.
AUDIENCE: So for the scaling--
GABRIEL SANCHEZ-MARTINEZ: Which scaling method?
AUDIENCE: The slide 41
GABRIEL SANCHEZ-MARTINEZ: 41, OK.
AUDIENCE: You've got an itinerary and an itinerary, so how many itineraries do you have?
GABRIEL SANCHEZ-MARTINEZ: Oh many, many, many, many.
AUDIENCE: [INAUDIBLE]
GABRIEL SANCHEZ-MARTINEZ: Any possible combination of-- I don't know if I have that here.
AUDIENCE: This is only one example of one trip.
GABRIEL SANCHEZ-MARTINEZ: Yeah, so--
AUDIENCE: So you have--
GABRIEL SANCHEZ-MARTINEZ: So we know that there are trillions of solutions that satisfy the control total. I forget how many unique itineraries there are, but many, many, many. It's a large number in a city like London, particularly.
AUDIENCE: So for this method, you--
GABRIEL SANCHEZ-MARTINEZ: So this is a computationally intense activity. Yeah.
AUDIENCE: You need the APC information.
GABRIEL SANCHEZ-MARTINEZ: On bus, you would want to have that. That would be one control total that you could use for bus. This method is flexible, though, because it doesn't require that you have control totals everywhere. You just use whatever control total you trust.
So say, if you didn't have APC on buses, then you would only use the control total on rail. If you have APC on some buses, you could use the control totals on those buses to improve the scaling information. But it doesn't require that all the places have counts.
Does that make sense? Because you have, essentially, a list of count nodes. And that list is not necessarily a complete list of places that people go through. And then you have all the list of itineraries that you see, and you want to associate those.
Again, no proof of convergence here. But [AUDIO OUT]. And on the toy examples we run, we observe these properties of convergence rate and error at the end, which is consistent with normal or more traditional applications of IPF. So we can hypothesize that it behaves very similarly. Question in the back.
AUDIENCE: [INAUDIBLE] generate a list of reasonable itineraries.
GABRIEL SANCHEZ-MARTINEZ: It's not a list of reasonable itineraries. It's a list of inferred itineraries. So it's an area that was inferred because I saw you tapping in here, out there, then transferring, taking this bus, and maybe you took three other buses after that. Maybe one person did that.
AUDIENCE: [INAUDIBLE]
GABRIEL SANCHEZ-MARTINEZ: That's one itinerary that's included here.
AUDIENCE: So do we just ignore the possibility that the people with uninferred destinations have--
GABRIEL SANCHEZ-MARTINEZ: Something different? Yes. You could generate every combination, but that's maybe intractable. So what we're doing is only considering itineraries that were observed, and only scaling those up. But that's a good point. There might be some people that were counted, but didn't have an inference, and their itinerary might be completely different. And this method doesn't handle that.
AUDIENCE: How different is [AUDIO OUT] the scaling matrix, compared with the traditional matrix that you can calculate with the sample [INAUDIBLE] to infer the alighting?
GABRIEL SANCHEZ-MARTINEZ: You mean the traditional IPF?
AUDIENCE: Yes. Are you [INAUDIBLE]? Is it very different? Or is--
GABRIEL SANCHEZ-MARTINEZ: No. Well, it depends on the accuracy of your IPF procedure and the accuracy of your control totals. Yeah, if your seed matrix is very good, then IPF should [AUDIO OUT] well. [AUDIO OUT] any applications of IPF, we just seed it to one and run it.
And you have the issue of the transfer, which would plague that, and it would amplify the error because presumably, your seed matrix-- a lot of people might be getting off here to transfer, and you may not be considering that. If you use IPF to scale up the inferred portion, for example, that would happen.
So I think through our validation, we have seen that there's more reason to trust the inference algorithms scaled up than to just take control totals and scale those up. From an information theory perspective, we're adding information, so you should-- even if we can't provide evidence that absolutely, it is the case, from information theory, we see we're consuming more information to generate this estimate, then that the estimate should be more accurate.
But it depends on whether your inferences were correct or not. That depends on the assumptions you made about people. So many assumptions go into this, and it's hard to say exactly. All right. So do you want to look at Boston? Or--
GABRIEL SANCHEZ-MARTINEZ: Let's see. Turn it off. All right, so here's Boston.
GABRIEL SANCHEZ-MARTINEZ: It's a much smaller city. And this is an earlier video where we still had some issues with ODX. And so some of them, you could perhaps detect by looking at the animation. So that's another application of this animation is to find issues with the algorithm.
In this case, people are being routed through the actual paths. So that's another difference between the video of London, where people were bursting in linearly.
AUDIENCE: Right.
GABRIEL SANCHEZ-MARTINEZ: Here, it looks less bursty, and part of that is that the nodes are moving through paths.
AUDIENCE: You see these thick dots along the red line.
GABRIEL SANCHEZ-MARTINEZ: Yeah.
AUDIENCE: [INAUDIBLE]
GABRIEL SANCHEZ-MARTINEZ: Yeah. Some of the issues are very slowly-moving dots. So you see some green dots that barely move. So those are errors that have been fixed already, and we should run this program again to generate a new animation without those errors.
But yeah, you see the same pattern. This is now late at night. So you can see where people are still at work, or perhaps at restaurants, or bars. And Boston system just shuts down. So it's not as alive at night because we're only looking at people who would take the T.
All right? Thank you. And if you have questions, I'll take them. Otherwise, I'll see you next class.