Flash and JavaScript are required for this feature.
Download the video from Internet Archive.
Andy Coravos gives an overview of US regulatory agencies and their role in overseeing devices (SaMDs) and how to submit public comment to these agencies. Mark Shervy gives an overview of institutional review boards (IRBs) for research with human subjects.
Speakers: Andy Coravos, Mark Shervey
Lecture 22.1: Regulation of ML/AI in the US slides (PDF - 2.9MB)
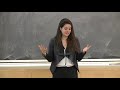
Lecture 22: Regulation of M...
PROFESSOR: All right. Let's get started. Welcome, ladies and gentlemen. Today it's my pleasure to introduce two guest speakers who will talk about the regulation of AI and machine learning and about both the federal FDA level regulation and about IRB issues of regulation within institutions. So the first speaker is Andy Coravos. Andy is the CEO and founder of Elektra Labs, which is a small company that's doing digital biomarkers for health care. And Mark is a data and software engineer for the Institute for Next Generation Healthcare at Mount Sinai in New York and was kind enough to come up to speak to us today.
So with that, I'm going to introduce them and sit back and enjoy.
ANDY CORAVOS: Thank you. Thank you for having us. Yeah, so I am working on digital biomarkers, and I'm also a research collaborator at the Harvard MIT Center for Regulatory Sciences. So you all have a center that is looking just at how regulators should think about some of these problems. And then I'm also an advisor at the Biohacking Village at DEFCON, which we can talk a little bit more about.
My background-- I'm a software engineer, had worked with the FDA formerly as an entrepreneur resident in the digital health unit, and then spent some time in corporate land.
MARK SHERVEY: I'm Mark Shervey. I work at the Institute for Next Generation Healthcare at Mount Sinai. I've been there about three years now. My background is in software and data engineering, coming mostly from banking and media. So this is a new spot. And most of my responsibilities focus around data security and IRB and ethical responsibilities.
ANDY CORAVOS: We also know how much people generally love regulatory conversations, so we will try to make this very fun and exciting for you. If you do have questions, as regulations are weird and they're constantly changing, you can also shoot us a note on Twitter. We'll respond back if you have things that come up. Also, the regulatory community on Twitter, amazing. When somebody comes out with, like, what does real world data actually mean, everybody is talking to one another.
So once you start tapping into-- I'm sure you have your own Twitter communities, but if you tap into the regulatory Twitter community, it is a very good one. The digital health unit tweets a lot at the FDA. OK. Disclaimers-- these are our opinions and the information that you'll see here does not necessarily reflect the United States government or the institutions that we are affiliated with. And policies and regulations are constantly changing. So by the time we have presented this to you, most likely parts of it are wrong.
So you should definitely interact early and often with relevant regulatory institutions. Your lawyers might say not to do that. There are definitely different ways, and we can talk through how you'd want to do that. But especially as a software engineer and developing anything on the data side, if you spend too much time developing a product that is never going to get through, it is really a wasted period of time. So working with the regulators, and given how open they are right now to getting feedback, as you saw with the paper that you read, is going to be important.
And then the last thing, which Mark and I talk a lot about, is many of these definitions and frameworks have not actually happened yet. And so when somebody says a biomarker, they might actually not mean a biomarker, they might mean a measurement. I'm sure you know this. When someone's like, I work in AI, and you're like, what does that actually mean? So you should ask us questions. And if you think about it, the type of knowledge that you have is a very specific, rare set of knowledge compared to almost everybody else in the country. And so as the FDA and other regulators start thinking about how to regulate and oversee these technologies, you can have a really big amount of influence.
And so what we're going to do is a little bit of the dry stuff around regulatory, and then I am going to somewhat plead with you and also teach you how to submit public comments so that you can be part of this regulatory process. And then--
MARK SHERVEY: I will speak about the Institutional Review Board. How many people in here have worked with an IRB or aware of them? OK, good. That's a good mix. So it'll be a quick thing, just kind of reviewing when to involve the IRB, how to involve the IRB, things you need the IRB for and some things that you don't, as an alternative to taking the FDA approach.
ANDY CORAVOS: All right, good. And then I'll go first, and then we'll go through IRBs, and then we'll leave the last part for your impressions of the paper. OK. So before I start, I'll ground us in some ideas around algorithmically-driven health care products. So as you know, these can have wide ranges of what they can do. A general framework that I like to use to think about them is products that measure, that diagnose, or treat. So measurement products might include things like digital biomarkers or clinical decision support.
Diagnostics might take that measurement and then say whether or not somebody has some sort of condition given those metrics. And then treatment are ideas around digital therapeutics. How many people here think that software can treat a person? A few, maybe. OK. And I think one thing that people don't think about always when they have these sorts of tools is-- and you all probably think about this a lot more-- is even something as simple as a step count is an algorithm. So it takes your gyroscope, accelerometer, height, weight, and age, and then it predicts whether or not you've made a step. And if you think about the types of different steps that people make, older people drag their feet a little bit more than younger people.
So an algorithm for a step count looks very different from an algorithm for younger people for step count. And so all of these tools have some level of error, and they're all algorithms, effectively. One of my favorite frameworks as you start thinking about-- a lot of people are very interested in the measurement side around what's called digital biomarkers. And it turns out that the FDA realized that many people, even within their own agency, didn't know what a biomarker was, and everyone was using the term slightly differently, probably how people approach you slightly differently of what machine learning actually is.
And so there is a really good framework around the seven different types of biomarkers that I'd highly recommend you read if you go into this area. A digital biomarker only, in my definition and how other people have started to use this, is the way that that measurement is collected. And so you might have a monitoring biomarker, a diagnostic biomarker, but it is collected in an ambulatory, remote way that is collecting digital data. And this type of data is very tricky. To give you an example of why this is particularly difficult to regulate, so think about a couple of products that just look at something that would be simple, like AFib.
So AFib is an abnormal heart condition. You might have seen in the news that a number of different companies are coming out with AFib products. Put simply, there is obviously a large stack of different types of data, and one person's raw data is another person's processed data. So what you see on this chart is a list of five different companies that are all developing AFib products, from whether or not they develop the product internally, which is the green part, versus whether or not they might use a third party product, so developing an app on top of somebody else's product. And so in a broad way, thinking about going from the operating system to the sensor data.
So somebody might be using something like a PPG sensor and collecting this sort of data from their watch and then doing some sort of signal processing, then making another algorithm that makes some sort of diagnostic. And then you have some sort of user interface on top of that. So if you are the FDA, where would you draw the line? Which part of this product, when somebody says my product is validated, should it be actually validated? And then thinking about what does it actually mean if something is verified versus validated.
So verified being like, if I walk 100 steps, does this thing measure 100 steps? And then validation being, does 100 steps mean something for my patient population or for my clinical use case? And so one of the things that the FDA has started to think through is how might you decouple the hardware components from the software components, where you think about some of the hardware components as the ways that you would-- effectively, the supply chain for collecting that data, and then you would be using something on top. And so maybe you have certain types of companies that might do some sort of verification or validation lower down the stack, and then you can innovate higher up.
And so these measurements have pretty meaningful impacts. So in the past, a lot of these tools, you really had to go into the clinic. It was very expensive to get these sorts of measurements. And more and more, a number of different companies are getting their products cleared to use in some care settings with a doctor or possibly to inform decisions at home. All right. And so in the last of some examples is around digital therapeutics. So I had worked with a company that was using a technology based out of UCSF that is developing, effectively, a video game for pediatric ADHD. And so when kids play the game, they reduce their ADHD symptoms.
And one of things that's pretty exciting about this game it is a 30-day protocol. And unlike something like Ritalin or Adderall, where you have to take that drug every day for the rest of your life as you reduce the symptoms, this seems to have an effect that, after 30 days, is more long-term and that when you test somebody months down the line, they still retain the effects of the treatment. So this technology was taken out of UCSF and licensed to a company called Akili, who decided, hey, we should just structure ourselves like a drug company. So they raised venture capital like a drug company, they ran clinical trials like a drug company, and they're now submitting to the FDA and might be the first prescription drug.
So anybody who was told that video games might rot your brain, you now have a revenge, maybe. So the FDA has been looking at more and more of these tools. I don't have to tell you, you're probably thinking a lot about it. And the FDA has been clearing a number of these different types of algorithms. And one of the questions that has come up is, what part of the agency should you think about? What are you claiming when you use these sorts of algorithms? And what ones should be cleared and what's not? And how should we really think about the regulatory oversight for them?
And a lot of these technologies enable things that are really quite exciting, too. So it's not just about the measurement, but what you can do with them. So one thing that has a lot of people really excited about is an idea around decentralized clinical trials. No block chains here. You might be able to build it with a blockchain, but not necessary. So on the y-axis, you can think about where are the data collected. So is it collected at a clinical site, or is it collected remotely?
And then the method is how it's collected. So do you need a human to do the interaction, or is it fully virtual? So at the top you can think about somebody doing telemedicine, where they call into somebody at home and then they might ask some questions and fill out a survey. On the bottom, you can imagine in a research facility where I'm using a number of different instruments, and perhaps I'm in a Parkinson's study and you're measuring my tremor with some sort of accelerometer.
And so the challenge that's happening is a lot of people use all of these terms for different things when they mean decentralized trials. Is it telemedicine? Is it somebody who's instrumented with a lot of wearables? How do you know that the data are accurate? But this is, I think, in many instances really exciting because the number one reason why people don't want to enroll in a clinical trial is to get a placebo. I think nobody really wants to participate in research if you're not getting the actual drug. And then the other reason is location. People don't want to have to drive in, find parking, participate. And this allows people to participate from home.
And the FDA has been doing a lot of work around how to rethink the clinical trial design process and incorporate some of this real world data into decision-making. Before I jump into some of the regulatory things, I want to just set a framework of how to think about what these tools can do. So these are three different scenarios of how you might use software in a piece of clinical research. So imagine that somebody has Parkinson's and you want to measure how their Parkinson's is changing over time using a smartphone-based test.
You have a standard Parkinson's drug that they would use, and then you would collect the endpoint data, which is how you see if that drug has performed using a piece of software. Another idea would be, say you have an insulin pump and then you have a CGM that is measuring your blood sugar levels, and you want to dose the insulin based on those readings. You might have software both on the interventional side and on the endpoint side. Or, like the company we talked about, which has a digital product, they said the only thing we want to change in the study is that the intervention is digital, but we want you to compare us like any other intervention for pediatric ADHD. So we want to use standard endpoints and not make that an innovation.
The challenge here is the first one, most likely, would go to the drug side of the FDA. The second one would go to both the drug and the device side of the FDA as a combination product. And the final one would just go to devices, which has been generally handling software. We've never really had products at the FDA, in my opinion, where-- we don't have drugs that can measure, diagnose, and treat and change all the different ways. And so you're now having software hitting multiple different parts of a system, or it might even be the same product, but in one instance it's used as an intervention, another instance it's used as a diagnostic, another it's to inform or expand labeling. And so the lines are not as clean anymore about how you would manage this product.
So how do you manage these? There's a couple agencies that are responsible for thinking through and overseeing health care software. The big one that we'll spend most of our time on is the FDA. But it's also worth thinking about how they interact with some of the other ones, including ONC, FCC, and FTC. So the FDA is responsible for safety and effectiveness and for facilitating medical product innovation and ensuring that patients have access to high quality products.
The ONC is responsible for health information technology. And you can imagine where the lines between storing data and whether or not you're making a diagnosis on that data start to get really vague, and it really might be the exact same product but just the change of what claim you're making on that product. And most of these products have some level of connectivity to them, so they also are working with FCC and have to abide by the ways that these tools are regulated by this agency.
And then finally, and probably most interesting, is around the FTC, which is really focused on informing consumer choice. And if you think about FDA and FTC, they're actually really similar. So both of these agencies are responsible for consumer protection, and the FDA really takes that with a public health perspective. So in many instances, if you've seen some of the penalties around somebody having deceptive practices, it actually wasn't the FDA who stepped in, it was the FTC. And I think some of the agencies are thinking about where do their lines end and where do others begin.
And in many instances, as we've really seen with a lot of probably bad behavior that happens in tech, there's really gaps across multiple places where nobody's stepping in. And then there's some also non-regulatory agencies to think about. An important one is around standards and technology. You probably think about this all the time with interoperability and whether or not you can actually import the data. There are people who spend a lot of time thinking about standards. It is a very painful and very important job to promote innovation.
OK. So the FDA has multiple centers. I'm going to use a lot of acronyms, so you might want to write this down or take a picture. And I'll try to minimize my acronyms. But there are three centers that will be the most interesting for you. So CDER is the one for drugs, and this is the one where you would have a regular drug and possibly use a software product to see how that drug is performing. CDHR is for devices. And CBER is for biological products. I will probably use drugs and biologics in a very similar sort of way. And the distinctions that we'll spend most of our time on are around drugs versus device.
There's a bunch of policy that is coming out that is both exciting and making things change. So one of the big ones is around the 21st Century Cures. This has accelerated a lot of innovation in health care. It's also changed the definition of what device is, which has a pretty meaningful impact on software. And the FDA has been thinking a lot about how you would actually incorporate these products in. I think there is a lot of people who are really excited about them. There's a lot of innovation, and so how do we create standards both to expand labeling, be able to actually ingest digital data, and have these sorts of digital products that are actually under FDA oversight and not just weird snake oil on the app store?
But what is a medical device? Pretty much, a device is like anything that's not the other centers, which has a big catch-all for all the other components. And so one of the big challenges for people is thinking about what a device is. If you think about generally what the FDA does, it doesn't always make sure that your products are safe and effective. They check whether or not you claim that they are safe and effective. So it's really all about claims management and what you're claiming that this product can do and evaluating that for marketing.
Obviously if your product causes very significant harm, that is an issue. But the challenge really happens to be when somebody makes-- the product can do something that it doesn't necessarily claim to do, but then you are able to imply that it does other things. Most people don't really have a really good understanding of what the difference is between informing a product versus diagnosing a product, and so I think in many instances for the public, it gets a bit confusing.
So as we talked about before, the FDA has been thinking about how do you decouple the hardware from the software, and they've come up with a concept around software as a medical device, so the software that is effectively defined as having no hardware components where you can evaluate just this product, and this is pronounced SaMD. And SaMDs are pretty interesting. This is very hard to read, but I pulled it straight from legal documents, so you know I'm not changing it. So something that's interesting about SaMD-- so if you go all the way to the end-- so if you have electronic health care data that's just storing health data, that is not a SaMD and often can go straight to market and is not regulated by the FDA.
If you have a piece of software that is embedded into a system, so something like a pacemaker or a blood infusion pump, then that is software in a medical device, and that's not a SaMD. So there's a line between these about what the functionality is that the product is doing, and then how serious is it, and that informs how you would be evaluated for that product. And if you haven't noticed, I try not to almost ever use the term device. So when I talked about these connected wearables and other sorts of tools, I will use the word tool and not device because this has a very specific meaning for the FDA.
And so if you're curious whether or not your product is a device or your algorithm is a device, first, you should talk to the regulators and talk to your lawyer. And we'll play a little game. So there are two products here. One is an Apple product and one is a Fitbit product. Which one is a device? I'm going to call on somebody randomly, or someone can raise their hand and offer as tribute. OK, which one?
AUDIENCE: I think Apple received 510(k) clearance, so I'd say the Apple Watch device. I'm not sure about the Fitbit, but if it's one or the other, then it's probably not.
ANDY CORAVOS: That's very sharp. So we'll talk about this. Apple did submit to the FDA for clearance, and they submitted for de novo, which is very similar to a 510(k). And they submitted for two products, two SaMDs. One was based on the signal from their PPG, and the second was on the app. So it has two devices, neither of which are hardware. And the Fitbit has, today, no devices. How about now? Is it a device, or is it not a device? Trick question, obviously, because there are two devices there, and then a number of things that are not devices.
So it really just depends on what you are claiming the product does. And back to that set of modularity, what is actually the product? So is the product a signal processing algorithm? Is the product an app? Is the product the whole entire system? And so people are thinking about, strategically, frankly, which parts are devices because you might want somebody else to be building on your system. So maybe you want to make your hardware a device, and then other people can build off of it. And so there are strategic ways of thinking about it.
So the crazy thing here, if you can imagine this, is that the exact same product can be a device or not a device through just a change of words and no change in hardware or code. So if you think about whether or not my product is a device, it's actually generally not the most useful question. The more useful question is, what is the intended use of the product? And so, are you making a medical device claim with what your product is doing?
Obviously this is a little bit overwhelming, I think, in trying to figure out how to navigate all of this. And the FDA recognizes that, and their goal is to increase innovation. And so particularly for products like software, they're having constant updates. It seems a little bit difficult if you're constantly figuring out all the different words and how you're going to get these products to market. So something that I think is really innovative by the FDA is piloting-- this is one example of a program that they're thinking through, which is working with nine different companies. And the idea is, can you pre-certify an entire company that is developing software as an excellent company across a series of objectives, and then allow them to ship additional updates?
So today, if you had an update and you wanted to make a change, you have to go through an entire 510(k) or de novo process or other type of process, which is pretty wild. If you imagine that we only would let Facebook ship one update a year, that would be crazy. And we don't expect Facebook to maintain or sustain a human life. And so being able to have updates in a more regular fashion is very important. But how do you know that that change is going to have a big impact or not?
And I'll pause on this, but you all read the document. I'm actually very glad that you read this document without talking to us because you were the exact audience of somebody who would not necessarily have the background, and so it needs to be put in a way that is readable for people who are developing these types of products to know how to go into them. We'll save some time at the end of the discussion because I'm curious how you perceived the piece. But you should definitely trust your first reading as a honest, good reading. You also probably read it way more intensely than any other person who is reading it, and so the notes that you took are valid. And I'm curious what you saw.
OK. Another thing to help you be cool at cocktail hour. FDA cleared, not the same thing as FDA approved. OK. So for devices, there are three pathways to think about. One is the 510(k), the next is de novo, the next is a premarket approval, also known as a PMA. They're generally stratified by whether or not something is risky. And the type of data that you have to submit to be able to get one of these clearances varies. So the more risky you are, the more type of data that you have to have.
So de novos are granted, but people often will say cleared. 510(k)s are cleared. Very few products that you've seen go through a PMA process.
AUDIENCE: I have a question.
ANDY CORAVOS: Tell me.
AUDIENCE: Do you know why Apple chose to do a de novo instead of a 510(k)?
ANDY CORAVOS: I am not Apple, but if I had to guess, once you create a de novo, you can then become a predicate for other things. And so if they wanted to create a new class of predicates that they could then build on over time, and they didn't want to get stuck in an old type of predicate system, I think, strategically, the fact that they picked a PPG and their app-- I don't know what they'll eventually do over time, but I think it's part of their long-term strategy. Great question.
OK. So the tools are safe and effective, perhaps, depending on how much data is submitted. But what about the information that's collected from the tools? So today, our health care system has pretty strong protections for biospecimens, your blood, your stool, your genomic data. But we really don't have any protections around digital specimens. You can imagine how many data breaches we constantly have and what ads get served to us on Facebook. A lot of this is considered wellness data, not actually health data. But in many instances, you are finding quite a lot of health information from somebody in that.
And I have a lot more. We can nerd about this forever. But generally, there's a couple of things that are good to know, is that with most of this data, you can't really de-identify it anymore. Who here thinks I could de-identify my genome? You can't, right? My genome's unique to me. Maybe you can strip out personally identifiable information, but you're not really going to de-identify it. I am uniquely identifiable with 30 seconds of walk data. So all of this biometric signatures is pretty specific.
And so there are some agencies today who are thinking about how you might handle these sorts of tools. But in the end, there is, I think, a pretty substantial gap. So in general, the FDA is really focused on safety and efficacy, and safety is considered much more of a body safety and not as a we are very programmable as humans in the type of information that we see or change type of safety. So the data that we collect-- FTC could have a lot of power here, but they're a much smaller agency that isn't as well-resourced. And there's a couple of different organizations that are trying to think through how to do rulemaking for Internet of Things and how that data is being used.
But generally, in my opinion, we probably need some sort of congressional action around non-discrimination of digital specimen data, which would require a Congress that could think through, I think, a really difficult problem of how you would handle data rights and management. OK. So I'll go through a couple examples of how government agencies are interacting with members of the public, which I think you might find interesting. So, many of the government agencies are really thinking through, realizing that they are not necessarily the experts in their field in how do they get the data that they need. So a couple pieces that will be interesting for you, I think.
One is there is a joint group with the FDA and Duke, where they're thinking through what's called novel endpoints. So if you are working on a study today where you realize that you're measuring something better than the quote gold standard, and the gold standard is actually quite a terrible gold standard, how do you create and develop a novel metric that might not have a reference standard or a legacy standard? And this is a way of thinking through that. The second is around selecting a mobile technology. This used to be called mobile devices, and they changed it for the same reason around not calling things a device unless it is a device. And so these are thinking through what type of connected tech would you want to use to generate the patient data that you might use in your study.
All right. Who here knows what DEFCON is? Three of you. OK. So DEFCON is a hacker conference. It is probably one of the biggest hacker conferences. It is a conference that if you do have the joy of going to, you should not bring your phone and you should not bring your computer, and you should definitely not connect to the internet because there is a group called the Wall of Sheep, and they will just straight stream all your Gmail passwords plain text and your account logins and anything that you are putting on the internet.
This group is amazing. You may have also heard about them because they bought a number of voting machines last year, hacked them, found the voting records, and sent them back to Congress and said, hey, you should probably fix this. DEFCON has a number of villages that sit under the main DEFCON. One of them is called Biohacking Village. And there is some biohacking, so, like, doing the RFID chipping, citizen science. But there's also a set of people at Biohacking Village that do what's called white hat hacking.
So for people who know about this, there's black cat, where you might encrypt somebody's website and then hold them for ransom and do things that are disruptive. White hat hackers are considered ethical hackers, where they are doing security research on a product. So the hackers in the Biohacking Village started to do a lot of work on pacemakers, which are connected technologies. A lot of pacemaker companies-- an easy way to think about how they're thinking about this was the pacemaker companies are generally trying to optimize for battery life. They don't want to do anything that's computationally expensive.
Turns out, encrypting things is computationally expensive. They did a relatively trivial exploit where they were able to reverse engineer the protocol. Pacemakers stay in a low power mode as long as they can. If you ping it, it will turn into high power mode, so you can drain a multi-year battery of the pacemaker into a couple days or weeks. They were also able to reverse engineer the shock that a pacemaker can deliver upon a cardiac event. And so this has pretty significant implications for what this exploit can do.
With any normal tech company, when you have an exploit of this type, you can go to Facebook, you can go to Amazon, there is something called a coordinated disclosure, you might have a bug bounty, and then you share the update, you can submit the update, and then you're done. With the device companies, what was generally happening is the researchers were going to the device companies, hey, we found this exploit, and the device companies were saying, thank you, we are going to sue you now. And the security researchers were like, why are you suing us? And they said, you're tampering with our product, we are regulated by agencies, we can't just ship updates whenever we want, and so we have to sue you.
Turns out that is not true. And the FDA found out about this and they're like, you can't just do security researchers. If you have a security issue, you have to fix that. And so the FDA did something that was pretty bold, which was three years ago, they went to DEFCON. And if anyone has actually gone to DEFCON, you would know that you do not go to DEFCON if you are part of the government because there is a game called Find the Fed, and you do not want to be found. And of course, NSA, CIA, a lot of members of the government will go to DEFCON, but it is generally not a particularly friendly environment.
The Biohacking Village said, hey, we will protect you, we will give you a speaker slot, we really want to work together with you. And so over the last three years, the agency has been working closely with security researchers to really think through the best ways of doing cybersecurity, particularly for connected devices. And so if you look at the past couple of guidances, there's a premarket and post-market guidance where they've been collaborating, and they're very good and strong guidances.
So the FDA did something really interesting, which was in January, they announced a new initiative, which I think is quite amazing, called #WeHeartHackers. And if you go to WeHeartHackers.org, the FDA has been encouraging device manufacturers, like Medtronic and BD, and Philips, and Thermo Fisher and others, to bring their devices and work together with security researchers. Another group that is probably worth knowing is that if you think about what a lot of these connected products do, they, in many instances, might augment or change the way that a clinician does their work. And so today, if you are a clinician and you graduate from med school, you would take something like a Hippocratic oath to do no harm.
Should the software engineers and the manufacturers of these products also take some sort of oath to do no harm? And would that oath look similar or different? And that line of thinking helped people realize that there are entire professional communities and societies for people who do this sort of thing for doctors in their specialties, so a society for neuro oncology, society for radiology. But there's really no society for people who practice digital medicine. So there is a group that is starting now, which you all might like to join because I think you would all be part of this type of community, which is the society for-- it's called the DIME Society.
And so if you're thinking through, how do I do informed consent with these sorts of digital products, what are the new ways that I need to think through regulation, how am I going to work with my IRB, this society could be a resource for you. All right. So how do you participate in the rulemaking process? One is, I would highly encourage, if you get a chance to, to serve some time in government. There are more opportunities to do that through organizations like the Presidential Innovation Fellow, to be an entrepreneur resident somewhere, to be part of the US Digital Service.
The payment system of CMS is millions of line of COBOL, and so that obviously needs some fixing. And so if you want to do a service, I think this is a really important way. Another way that you can do it is submitting to a public docket. And so this is something I will be asking you to do, and we'll talk about it after, is how can you take what you learned in that white paper and ways that you can share back with the agency of how you would think about developing rules and laws around AI and machine learning.
There's a much longer resource that you can look at, my friend Mina wrote, which is that-- these are a couple of things to know. So anyone can comment, you will be heard. If you write a very long comment, someone at the agency, probably multiple, will have to read every single thing that you write, so please be judicious in how you do that. But you will be heard. And most of the time comments come from big organizations and people who have come together and not from the people who are experiencing and using a lot of the products. So in my opinion, I think someone like you is a really important comment and voice for the agency to have, and to have a technical perspective.
Another way that you can do this, which I'm going to put Irene on the spot, is we need new regulatory paradigms. And so when you are out at beers or ice cream, or whatever you do for fun, you can think through new models. And so we were kicking around an idea of, could you use a clinical trial framework to think about AI in general? So algorithms perform differently on different patient populations and different groups. You need inclusion/exclusion criteria. Should this be something maybe we even expand beyond health care algorithms to how you decide whether or not someone gets bail or teacher benefits?
And then the fun thing about putting your ideas online, if you do that, is then people start coming to you. And we realized there was a group in Italy who had proposed a version of FDA for algorithms, and you start to collect people who are thinking about things that you're thinking about. And now we will dig into the thing that you most likely will spend more time with than the government, which is your IRB.
MARK SHERVEY: Thank you. OK. I could probably not give the rest of this talk if you just follow the thing on the bottom. If you don't know if you're doing human subject research, ask the IRB, ask your professor, ask somebody. I think most of what I'm going to say is going to be a lot softer, squishier than what Andy went around, and it's really just to try to get the thought process going through your head of if we're doing actual human research, if the IRB has to be involved, what actually constitutes human research? And just to be sure that you're aware of what's going on there all the time.
We've done this. So research is systematic investigation to develop or contribute generalizable knowledge. So you can do that on a rock. What's important about human subjects research is that people's lives are on the line. Generally, the easiest thing to know is if there's any sort of identifiable information with the data that you're working with, that is going to fall under human subjects research. Things that won't are publicly available, anonymous data. There's all sorts of imaging training data sets that you can use that are anonymized to what is an acceptable level.
But to Andy's point, there's really no way to truly de-identify a data set. And with the amount of data that we're working with all right now in the world, it's becoming impossible to de-identify any data set if you have any other reference data set. So anytime you're working with any people, you are almost certainly going to have to involve the IRB, again.
So why the IRB is there, it's not specifically to slap you on the wrists. It's not that anything's expected to purposely do anything wrong. Although that has happened, that's such a small amount that it's just unhelpful to think that everybody is malicious. So you're not going to do anything particularly wrong, but there are things that you just may not know. And this is not the IRB's 1,000th rodeo, so if you bring something up to them, they'll know almost immediately.
Participants are giving up their time and information, so the IRB, more than keeping the institution from harm, is really protecting the patients first and the institution at the same time. But the main role is to protect the participants. Specifically, here's something that might not go through everybody's head, research that may be questionable or overly manipulative. That gets into compensation for studies. You can imagine certain places in an impoverished nation that you say, we'll pay $50,000 per person to come participate in this study, you can imagine people want to be in that study and it can become a problem.
So the IRB is also a huge part of making sure that the studies aren't actually affecting anybody negatively in that kind of sense. Now, before I do, this next slide gets dark for a second, so we'll try to move through it. But it talks about how the IRB came about. So we start with the Nuremberg Code, human research conducted on prisoners and others, not participants but subjects of research. Tuskegee experiment, another thing that people were not properly consented into the studies. They didn't know what they were actually being tested for, so they couldn't possibly have consented.
The study went for 40 years instead of six months. And even after a standard of care had been established, the study continued on. That essentially is what began the National Commission for Protection of Human Subjects, which led to the IRB being the requirement for research. And then five years later, the Belmont Report came out, essentially enumerating these three basic principles, respect for participants, beneficence as far as do no harm, don't take extra blood if it just makes it more convenient, don't add extra drug if you just want to see what happens, and then just making sure that participants are safe outside of any other harm that you can do.
So we follow the Belmont Report. That's essentially the state of the art that we have now with modernization moving forward. This is not something to really worry about, but HHS has a great site that has a flow chart for just about any circumstance that you can think of to decide if you're actually doing human subjects research or not. This is pretty much the most basic one. You can go through it on your own. Just to highlight the main thing that I think you guys will all probably be worried about, is you will be collecting identifiable data, which just immediately puts you in IRB land.
So anytime you can identify that that's a thing that's happening, you're just there, so you don't really have to go through any of this. What is health data? So you have names obviously. Most of these are either identifications or some sort of identifying thing. The two, I guess, that a lot of people maybe gloss over that aren't so obvious is zip codes. You have to limit them to the first three numbers of a zip code, which gives a generalizable area without actually dialing in on a person's place.
Dates are an extremely sensitive topic. So anytime you're working with actual dates, which I assume in wearable technologies you're going to be dealing with time series data and that kind of stuff. There are different ways of making that less sensitive. But anytime you're dealing with research, anytime we're dealing with the electronic health records, we deal in years, not in actual dates, which can-- it creates problems if you are trying to do time series analysis for somebody's entire health record, in which case you can get further clearance to work with more identifiable data. But that is progressive as it can be.
There's no reason to start with that kind of data if you don't. So it's always on a need to know. Finally, if you're working with patients older than 90, 90 or older, they are just generalized as a category of greater than 90. The rest of these, I think, are fairly guessable, so we don't have to go through them. But those are the tricky ones that some people don't catch. Again, just limit the collection of PHI as strictly as possible. If you don't need it, don't get it. If you're sharing the data, instead of sharing an entire data set if you do have strong PHI, limit what you're giving or sharing to another researcher.
That's just a hygiene issue, and it's really limiting the amount of errors that can happen. So why is this so important? The IRB, again, is particularly interested in protecting patients and making sure that there's as little harm, if any, done as possible to patients. Just general human decency and respect. There's institutional risk if something is done without an IRB, and you can't publish if you have done human subjects research without an IRB.
Those two are kind of the stick, but the carrot really should be the top two, as far as just human decency and making sure that you've protected any patients or any participants that you have involved in your research. These are a couple of violations. We don't have to get too far into it, but they were both allegedly conducted without any IRB approval. There's possible fraud involved, and it ruined both of their careers. But it put people at huge exposures to unhealthy conditions.
This is probably a much bigger common issue that you're going to have. PHI data breaches, they happen a lot. They're generally not breaches from the outside. They're accidents. Somebody will set up a web server on the machine serving PHI because they found it easier to work at home one day. It could just be they don't know how software is set up. So anytime you're working with PHI, you've really got to overdo it on knowing exactly how you're working with it.
Other breaches are losing unencrypted computers, putting data on a thumb drive and losing it. The gross amount of data breaches happen just from negligence and not being as careful as you want to be. So that's always good to keep in mind. I guess a new thing with the IRB and digital research is things have been changing now from face to face recruitment and research into being able to consent online to be able to reach millions of people across the world and allowing them to consent on their own. So this has become, obviously, a new thing since the Belmont Report, and it's something that we are working closely with our IRB to make sure that we're being as respectful as we can to the patients, but also making sure that we can develop software solutions that are not hurting anybody and develop into swim lanes.
So what we've come up with a framework for is that there's a project which is-- we're studying all cancers. So you can post reports about different research that's going on, things that seem important. A study is an actual person that's consented to a protocol, which is human research and subject to IRB. Then we'll have a platform that the users will use, and that will be like a website or an iPhone app that they can get literature information about what the project is going on. And then we'll have a participant who is actually part of a study, who's, again, covered under IRB through consent.
So why this kind of development has been important, the old way of software development was the waterfall approach, where you work for three weeks, implement something, work for three weeks, implement something, where we have moved to a Agile approach in software. And so while Agile makes our lives a lot easier as far as development, we can't be sure what we're doing isn't going to affect patients in certain contexts. So within a study, working Agile makes no sense.
We have we want to work with the IRB to approve things, but IRB approval takes between two and four weeks for expedited things. When we talk about projects and stuff, that's where we want to work safely in an Agile environment and try to figure out places where the IRB doesn't necessarily have to be involved or doesn't want to be involved and that there isn't any added patient risk whatsoever in working in that kind of environment. So it's working with software products versus studies, and so working with the IRB to be sure that we can separate those things and make sure that things move on as well as possible without any added harm.
So that's these categories again. So project activity would be social media outreach, sharing content that is relevant to the project and kind of just informing about a general idea. A study activity is what you would generally be used to with consent, data sharing, actually participating in a study, whether it's through a wearable, answering questions, and then withdrawing in the process. And the study activities are 100% IRB, where the project activities that aren't directly dealing with the study can hopefully be separated in most cases.
So the three takeaways really are just if you don't know, ask, limit the collection of PHI as strictly as possible, and working in Agile developments are great but it is unsafe in a lot of human research, so we have to focus on where that can be used and where it can't. And that's it. Thank you.
[APPLAUSE]
Oh.
AUDIENCE: I have a question about how it's actually done. So as the IRB, how do you make sure that your researcher is complying? Is that, like, writing a report, doing a PDF, or is there a third party service?
MARK SHERVEY: Yeah, yeah. So we certify all of our researchers with human research and HIPAA compliance, just blanket. And if you provide that and your certifications are up to date, it's an understanding that the researcher knows what they should be looking out for and that the IRB understands.
AUDIENCE: So is that a third party?
MARK SHERVEY: Oh, yeah, yeah. We use a third party. You can have-- I don't-- we use a third party.
PROFESSOR: Can I just add--
MARK SHERVEY: Oh, yeah.
PROFESSOR: So at MIT, there's something called COUHES, the Committee on Use of Humans as Experimental Subjects, and they are our official IRB. It used to be all paper. Now there's an electronic way where you can apply for a COUHES protocol. And it's a reasonably long document in which you describe the purpose of the experiment, what you're going to do, what kind of people you're going to recruit, what recruiting material you're going to use, how you will handle the data, what security provisions you have.
Of course, if you're doing something like injecting people with toxins, then that's a much more serious kind of thing, and you have to describe the preliminary data on why you think this is safe and so on. And that gets reviewed at, essentially, one of three levels. There is exempt review, which is-- you can't exempt yourself, but they can exempt you. And what they would say is, this is a minimal risk kind of problem. So let's say you're doing a data only study using mimic data, and you've done the city training, you've signed the data use agreement.
You're supposed to get IRB permission for it. There is an exception for students in a classroom, in which case I'm responsible rather than making you responsible. But if you screw it up, I'm responsible. The second level is an expedited approval, which is a low risk kind of approval, typically data only studies. But it may involve things like using limited data sets, where, for example, if you're trying to study the geographical distribution of disease, then you clearly need better geographical identifiers than a three-digit zip code, or if you're trying to study a time series, as Mark was talking about, you need actual dates. And so you can get approval to use that kind of data.
And then there's the full on review, which takes much longer, where they do actually bring in people to evaluate the safety of what you're proposing to do. So far, my experience is that mostly with the kinds of studies that we do that are representative of the material we're studying in class, we don't have to get into that third category because we're not actually doing anything that is likely to harm individual patients, except in a kind of reputational or data-oriented sense, and that doesn't require the full blown review. So that's the local situation.
MARK SHERVEY: Yeah, thank you. Yeah, I think I misunderstood the full range of the question. Yeah, and that's roughly our same thing. So we have-- Eddie Golden is our research project manager, who is my favorite person in the office for this kind of stuff. She keeps on top of everything and makes sure that the right people are listed on research and that people are taken off, that kind of stuff. But it's a good relationship with the IRB on that kind of stuff. Yeah?
AUDIENCE: So I'm somewhat unfamiliar with Agile software development practices. On a high level, it's just more parallelized and we update more frequently?
MARK SHERVEY: Yeah, yeah. I don't know if I took that slide out, but there's something where Amazon will deploy 50 million updates per year or something like that. So it's constantly on an update frequency instead of just building everything up and then dropping it. And that's just been a new development in software.
AUDIENCE: Can we ask questions to both you guys?
ANDY CORAVOS: Yeah.
AUDIENCE: Can you tell us more about Elektra Labs? I couldn't fully understand. Are you guys more consultantancy for all these, we'll call them, tool companies? Or is it more like a lobbying kind of thing? The reason I ask this is also because I wonder what your opinion is on a third party source for determining whether these things are a good or bad kind of thing because it seems like the FDA would have trouble understanding. So if you had some organic certified kind of thing, would that be a useful solution? Or where does that go wrong?
ANDY CORAVOS: Mm-hm, yeah. So what we're building with Elektra is effectively a pharmacy for connecting technologies. So the way that today you have pharmacies that have a formulary of all the different drugs that are available, this is effectively like a digital pharmacy, like a Kelley Blue Book of all the different tools. And then we're building out a label for each of them based on as much objective data as we can, so that we're not scoring whether or not something's good or bad. Because in most instances, things aren't good or bad and absolute, they're good or bad for a purpose.
And so you can imagine something-- maybe you need really high levels of accuracy, so you need to know whether or not that tool has been verified and validated in certain contexts in certain patient populations. Even if the tool's accurate, if you're to recharge it all the time or you can't wear it in the shower, you won't have the usability, or if the APIs are really hard to work with. And then security profile, whether or not they have coordinated disclosure, how they handle things like the tool companies, like a software bill of materials and what kind of software is used. And then even if the tool is accurate, even if it's relatively usable, even if it's secure, that doesn't solve the Cambridge Analytica problem, so how tools are doing a third party transfer.
And so one of the philosophies is we don't score, but we are building out the data set so when you are evaluating a certain tool, it's like a nutrition label. Sometimes you need more sugar, sometimes you need more protein. Maybe you need more security, maybe you really need to think about the data rates. Maybe you can take a leave on some of the accuracy levels. And so we're all building out this ability to evaluate the tools, and then also to deploy them like the way that a pharmacy would deploy them out.
One thing I would like to do with the group, if you all are down for it, out of civic duty-- and I'm serious, though. Voting is very important and submitting your comments to the public register is very important. And I read all your comments because Irene sent them to me, and they were very good. And I know probably people who came here want to polish everything and make them perfect. You can submit them exactly how they are. And I am very much hoping that we get about 95% of you to submit, and the 5% of you that didn't, like, your internet broke or something.
You can submit tonight. I will email Irene because you already have done the work, and you can submit it. But I would like to just hear some of your thoughts. So what I'm going to do is I'm going to use that same framework around, what would you keep? What would you change? And then change can also include, like, what was so confusing in there, that it didn't even really make sense? Part of the confusion might be that it was-- some regulations are confusing. But some of the confusion is that part of that document was not written by people who-- some people have technical backgrounds and some do not. And so sometimes some of the language might not actually be used in the way that industry is using it today, so refining the language. And then what did you see that was missing?
So here's what we're going to do. Keep, change slash confusing, and then start or add. And before I ask you, I want you to look at the person next to you, seriously, and if there's three of you, that's fine, and I want you to tell them when you will be submitting the comment. Is it tonight, tomorrow, or you are choosing not to? Just look at them and talk.
[INDISTINCT CHATTER]
There will be a link. I will send you all links. I will make this very easy. OK. All right. Who wants to start? We got three things on the board. Yes?
AUDIENCE: So one thing that-- I don't know if this is confusing or just intentionally vague, but for things like quality systems and machine learning practices, who sets those standards and how can they be adapted or changed?
ANDY CORAVOS: Mm-hm. I don't also know that answer, and so I would like you to submit-- one of the things that is nice is then that you have to respond, yeah. And I think it's also a little bit confusing, even the language. So people are using different things. People call it GXP, good manufacturing practice, good clinical practice. These are maintained, I think, in some instances by different orgs. I wonder if good algorithm practice gap or good machine learning practice-- yeah, that's a good thing. So who owns GXP? OK. Yes? You didn't have a question? No.
AUDIENCE: Just wanted to share something.
ANDY CORAVOS: Yes.
AUDIENCE: One of the things I found really to keep were the examples in the appendix. I don't know [INAUDIBLE].
--general guidelines, and so it's more that the language itself is more generalized and so the examples are really hopeful for what is a specific situation that's analogous to make.
ANDY CORAVOS: Yep. Like that? Yeah, examples are helpful. Yep?
AUDIENCE: Speaking of specifics, I thought around transparency they could have been much more specific and that we should generally adhere to guidelines as opposed to the exact set of data that is-- this algorithm is exactly what's coming out of it, the exact quality metrics, things like that that hold people accountable as opposed to there are many instances to not be transparent. And so if those aren't as specific, I worry that not that much really would happen there. The analog like I thought of was when Facebook asks for your data, they say here are the things that we need or that we're using, and it's very explicit. And then you can have a choice of whether or not you actually want that.
ANDY CORAVOS: OK.
AUDIENCE: So seeing something like that [INAUDIBLE].
ANDY CORAVOS: So part of it is transparency, but also user choice in data selection or--
AUDIENCE: Yeah, I think that was, for me, more of an analog because choice in the medical setting is a bit more complex. Someone who doesn't have the ability in that case or the knowledge to actually make that choice.
ANDY CORAVOS: Yeah.
AUDIENCE: I think at the very least saying this algorithm is using this, and maybe some sort of choice. So you can work with someone, and maybe there's some parameters around what you would or would not have that choice.
ANDY CORAVOS: Yep. Yes?
AUDIENCE: What if you added something about algorithm bias? Because I know that that's been relevant for a lot of other industries in terms of confidence within the legal system, and then also in terms of facial recognition not working fully across races. So I think that breaking things down by population and ensuring equitable across different populations is important.
ANDY CORAVOS: Yep. I don't know if I slept enough, so if I just gave this example-- but a friend of mine called me last week and asked for PPGs, so the sensor on the back. She was asking me if it works on all skin colors and whether or not it responds differently. And if it responds differently, whether or not somebody has a tattoo. And so for some of the big registries that are doing bring your own device data, you can have unintended biases in the data sets just because of how that it's processing. So yeah.
What do you think? What are ways-- I think Irene's worked with some of this. How do you think about whether or not something is-- what would be a good system for the agency to consider around bias?
AUDIENCE: I think maybe coming into consideration with [INAUDIBLE] system might be part of the GNLP. But I think it would be the responsibility of the designer to assess [INAUDIBLE].
ANDY CORAVOS: OK.
AUDIENCE: As a note, bearing this is our next lecture, so anyone who might be confused or want to talk about it more, we will have plenty material next time.
ANDY CORAVOS: You want to pick someone?
MARK SHERVEY: I'm sorry. Go ahead.
AUDIENCE: Me?
MARK SHERVEY: Yeah.
ANDY CORAVOS: Cold call.
[LAUGHTER]
Yeah?
AUDIENCE: Just adding off at another place, so it looked like there was a period for providing periodic reportings to the FDA on updates and all that. There could also be like a scorecard of bias on subpopulations or something to that effect.
ANDY CORAVOS: Mm-hm. That's cool. Have you seen any places that do something like that?
AUDIENCE: I remember when I read Weapons of Math Destruction from Cathy O'Neil, she mentioned some sort of famous audit. But I don't really remember the details.
ANDY CORAVOS: Yeah. When you do submit your comment, if you have ideas or links, or it can be posts or blogs or whatever, just link them in because one thing that you'll find is that we read a lot of things, probably the same things on Twitter, but other groups don't necessarily see all of that. So I think that Cathy O'Neil is really interesting work, but yeah, just tag stuff. It doesn't have to be formatted amazingly.
PROFESSOR: So in some of the communities that I follow, not on Twitter but email and on the web, there's been a lot of discussion about really terrible design of information systems in hospitals and how these lead to errors. Now, I know from your slide, Andy, that the FDA has defined those to be out of its purview. But it seems to me that there's probably, at the moment, more harm being done by information systems that encourage really bad practice or that allow bad practice than there is by retinopathy, AI, machine learning techniques that make mistakes.
So just this morning, for example, somebody posted a message about a patient who had a heart rate of 12,000, which seems extremely unlikely.
[LAUGHTER]
ANDY CORAVOS: Yep.
PROFESSOR: And the problem is that when you start automating processes that are based on the information that is collected in these systems, things can go really screwy when you get garbage data.
ANDY CORAVOS: Yeah. Have you thought about that with your system?
MARK SHERVEY: We cannot get good data. I mean, you're not going to get good data out of those systems. What you're seeing is across the board, and there's not much you can do about it other than validate good ranges and go from there.
PROFESSOR: Well, I can think of things to do. For example, if FDA were interested in regulating such devices-- oh, sorry, such tools--
ANDY CORAVOS: Yeah. Well, they would regulate devices. So one of the funny things with FDA is-- and I should have mentioned this-- is the FDA does not regulate the practice of medicine. So doctors can do whatever they want. They regulate-- well, you should look up exactly-- the way I interpret it is they regulate the marketing that a manufacturer would do. So I actually wonder if the EHRs would be considered practice of medicine or if it would be a marketing from the EHR company, and maybe that's how it could be under their purview.
PROFESSOR: Yeah.
ANDY CORAVOS: Yeah. Yes?
AUDIENCE: I guess something that I was surprised not to see as much about were privacy issues in this. I know there's ways where you can train machine learning models and extract information that the data was trained on. At least I'm pretty sure that exists. It's not my expertise. But I was wondering if anything like that [INAUDIBLE] have someone try to extract the data that you can't. But you talked about that a lot in your section of the talk, but I don't remember it as much [INAUDIBLE].
ANDY CORAVOS: OK. Yep. Realistically, how many of you do think you'll actually submit a comment? A couple. So if you're thinking you wouldn't submit a comment, just out of curiosity, I won't argue with you, I'm just curious, what would hold you back from submitting a comment? If you didn't raise your hand now, I get to cold call you. Yes?
AUDIENCE: I raised my hand before. We were just talking. Most of us have our computers open now. If you really want us to submit it as is, if you put it up, we could all submit.
ANDY CORAVOS: OK. OK, OK. Wow.
AUDIENCE: We are 95% [INAUDIBLE].
ANDY CORAVOS: All right.
PROFESSOR: So while Andy is looking that up, I should say when the HIPAA regulations, the privacy regulations were first proposed, the initial version got 70,000 public comments about it. And it is really true that the regulatory agency, in that case, it was Health and Human Services, had to respond to every one of those by law. And so they published reams of paper about responding to all those requests. So they will take your comments seriously because they have to.
AUDIENCE: I was going to say, is there any way of anonymously commenting? Or does it have to be tied to us, out of curiosity?
ANDY CORAVOS: I don't know. I think it's generally-- I don't know, I'd have to look at it again. I think most of them are public comments. I mean, I guess if you wanted to, maybe you could coordinate your comments and you could-- yeah, OK. Irene is willing to group comment. So you can also send if you'd like to do it that way, and it can be a set of class comments, if you would prefer. The Bitly is capital MIT all lowercase loves FDA, will send you over to the docket.
I'm amazed that that Bitly has not been taken already.
PROFESSOR: [INAUDIBLE] has been asleep on the job.
ANDY CORAVOS: What other questions do you all have? Yes?
AUDIENCE: So what is the line between an EHR and a SaMD? Because it said it earlier that EHR is exempted, but then it also says, oh, for example, with SaMD, it could be collecting physiological signals, and then they might send an audible alarm to indicate [INAUDIBLE]. And my understanding is some of EHRs do that.
ANDY CORAVOS: Mm-hm.
AUDIENCE: And so would they need to be retroactively approved and partially SaMD-ified? Or how's that work?
ANDY CORAVOS: So I'm not a regulator, so you should ask your regulator. A couple resources that could help you decide this is, again, it's about what you're claiming the product does, perhaps not what it actually does. The next thing, which I don't think-- I mean, I think if it really does that, you should also claim that it does what it does, especially if it's confusing for people. There's a couple of regulations that might be helpful. One is called Clinical Decision Support. And if you read any FDA things, they love their algorithms-- I mean, they love their algorithms, but they also love their acronyms.
So Clinical Decision Support is CDS, and then also Patient Decision Support. There's a guidance that just came out around the two types of decision support tools, and I would guess maybe that is supporting a decision, that EHR. So it might actually be considered something that would be regulated. There's also a lot of weird-- we didn't go into it, but there are many instances where something might actually be a device and the FDA says it's a device, but it will do something called enforcement discretion, which says it's a device but we will not regulate it as such. Which is actually a little bit risky for a manufacturer because you are device, but you can now go straight to market.
In some instances, you still have to register and list the product, but you don't have to necessarily get reviewed. And it also could eventually be reviewed. So the line of, is it a device, is it a device and you have to register, is it a device and you have to get cleared or approved, is why you should early and often-- yes?
AUDIENCE: I enjoyed your game with regards to Fitbit and Apple. And I have a question about the app. I know that you're not Apple either, but why do you think that Apple went for FDA approval versus Fitbit who didn't? What were the motivations for the companies to do that?
ANDY CORAVOS: I would say, in public documents, Fitbit has expressed an interest in working with the FDA. I don't know at what point they have decided what they submitted or had their package. They're also working with the pre-cert program. So I don't know what's happening behind the scenes. Yeah?
AUDIENCE: Does it give them a business edge, perhaps, to get FDA approval?
ANDY CORAVOS: I cannot comment on that.
AUDIENCE: OK, no worries.
ANDY CORAVOS: Yeah. I would say, generally, people want to use tools that are trustworthy, and developing more tools that have somebody of evidence is a really important thing. I think the FDA is one way of having evidence. I think there are other ways that tools and devices can continue to build evidence. My hope is over time, that a lot of these things that we consider to be wellness tools also have evidence around them. Maybe in some instances we don't always regulate vitamins, but you want to still trust that your vitamin doesn't have sawdust in it, right, and that it's a real product. And so the more that we, I think, push companies to have evidence and that we use products that do that, I hope over time this helps us.
PROFESSOR: Does it give them any legal protection to have it be classified as an FDA device?
ANDY CORAVOS: I'm not sure about that. Historically, it has helped with reimbursement. So a class two product has been easier to reimburse. That also is generally changing, but that helps with the business model around that.
PROFESSOR: Yeah. Well, I want to thank you both very much. That was really interesting. And I do encourage all of you to participate in this regulatory process by submitting your comments. And I enjoyed the presentations. Thank you.
ANDY CORAVOS: Yeah.
MARK SHERVEY: Thank you.
ANDY CORAVOS: Thank you.
[APPLAUSE]