Flash and JavaScript are required for this feature.
Download the video from Internet Archive.
Prof. Szolovits gives an overview of clinical care from the past to the present. The main topics covered are the goals of medicine, the tasks of medicine, public health, and paying for health care.
Speaker: Peter Szolovits
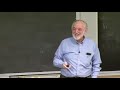
Lecture 2: Overview of Clin...
PETER SZOLOVITS: As David said, I've been at this for a long time. I am not a medical doctor. But I've probably learned enough medicine to be able to play one on television over the years.
And actually, that's relevant to today's lecture because today's lecture is really trying to set the scene for you to say, well, what are the kinds of problems that doctors are interested in by looking at what is it that they do. OK. So that's our goal for today.
So what we're going to do today is to talk about, in a very general way, what are the goals of health care. So how many of you are doctors? A couple. Great. OK. So fix me when I blow it. All right. Please feel free to interrupt.
So that's going to be my first task. And then the second one is going to be what are the things that people actually do in order to try to achieve these goals. What is the practice of medicine like? What is the process that generates the data that we're going to be using to learn from?
And then I can't resist talking a little bit about paying for health care at the end of the lecture because a lot of the problems that come up and a lot of the interest that people show in doing the kind of analysis we're talking about in fact is motivated by money. They want to be able to save money, or spend less money, or something like that. So it's important to know that.
OK. Medicine's been around for a long time. I think from probably the earliest of recorded history, there are discussions of people wondering what the cause of disease is, how to cure it. They came up with some fairly cockamamie theories because they didn't have a lot of scientific, modern approaches to it.
But for example, this is a photo on the left of a shaman I think from a Canadian Indian tribe who's working on the boy lying there who's sick. And this shaman would use his knowledge of experience that he'd had with other patients.
They did know a lot about medicinal plants. They knew something about how to care for injuries and things like that. And so this was an effective form of health care. Not much record keeping. You don't see electronic medical records system in that scene.
On the right is a modern shaman practicing at one of the New York area hospitals. And so there are traditional cultures in which that sort of hands on interaction with the healer is considered a very important part of the practice of medicine.
And if you listen to futurist doctors talking about what medicine is likely to be like, they emphasize the fact that the role of a healer is not just to be a good automaton who figures out the right things but is to persuade a patient to trust them to do the things that he or she is suggesting to the patient. And there are a lot of placebo effects that we know from lots and lots of experiments that say that if you think you're going to get better, you are going to get better, on average. No guarantees.
OK. Now modern medicine actually looks more like this. So this is an intensive care unit. And what you see is a patient who has got all kinds of electrical leads, and tubes, and things going into them and is surrounded by tons and tons of equipment which is monitoring him and perhaps keeping him alive. And so this is the high tech medicine that we think of as the contemporary version of clinical care.
Well, you might say, OK, what does it mean to be healthy? Right. If the goal of medicine is to make people or keep people healthy, what is health? So we turn to the World Health Organization. And they have this lovely, very comprehensive notion of the definition of health.
A state of complete physical, mental, and social well-being, not merely the absence of disease or infirmity. And then they categorize. This and they say, well, there's physical health, there's mental health, and there's social health. Social health is especially hard to measure. And I'll come back to that in a little while.
So what's easiest to measure is how long people live. And so we've had data on survival analysis for a long time. And this is kind of shocking. If you look here, this lower curve is from around 1800. And what it shows you is that if you lived in India around 1800, your life expectancy was about 25 years. It's not very good.
And if you lived in the richest countries, which in those days were typically European, in Belgium, your life expectancy was way up there at 40 years. Right. How many of you knew that? OK. Good. I didn't until I started looking at this.
Now by 1950, which is not that long ago, it was like 69 years ago, in Norway your expectation was that you'd live into your early 70s, in the US that you would live into your late 60s on average. There was still a huge cliff where, if you lived in Bhutan, or Somalia, or something, you were still down around 30.
Today, well in 2012, we're doing a lot better. And the thing that's striking is not only that the people who were doing well have gotten better but that a lot of the people who were doing very poorly have also gotten better. And so we're now up-- India, remember, was at 25 years of life expectancy and now it's in the high 60s. Now of course, these are averages. And so individuals vary a lot. But it's kind of interesting.
So if you look at the numbers, you see that even on a shorter term, there are big changes. So for example, if you're a male living in Rwanda, which is among the worst places in terms of life expectancy, your life expectancy, if you were born today, is about 62 and 1/2 years. Right. If you were born in 2001, it was only 38 years. Now what was going on in Rwanda in 2001?
Genocide. Yeah. They were killing each other. So that's sort of an exceptional situation. And that's gotten much better because they've stopped killing each other as genocidal attacks between the Hutu and Tutsi, I think, if I remember right.
What about South Africa? What was going on in South Africa in 2001. I'm not sure I heard you.
AUDIENCE: Failure to address the HIV crisis.
PETER SZOLOVITS: Yes. The government at the time was claiming that HIV was not the cause of AIDS and therefore there was no point in controlling HIV infections because AIDS was caused by something else. Pretty crazy.
So that was terrible. And they've gotten much better at it. So that's what you tend to see in a lot of African countries. And what you also see is that there has really been improvement everywhere.
So in the US, we went from males expected to live 74 years to almost 78 years, so about a four year increase in life expectancy over a period of just 17 years. You women, by the way, are going to outlive us men, on average. There's some biological thing that seems to work that way.
OK. So a typical way that people look at the survival of a population is to say, well, given a cohort of people born at some instant zero, what fraction of them are still alive after a certain period of time?
And what you see is that, of course, 2031 we haven't reached yet. And so these are projections based on sort of theoretical extrapolations of actual data. But the older data is real. And what you see is that from 1851 to, you know, 2011 let's say, these numbers have gone way up.
Now where have they gone up the most? Well, it used to be that childhood mortality was enormous. And so if you look at 1851, by age 10 about 30% of children had died. And so we've gotten a lot better at stopping that from happening.
People also look at curves like this. So this is a distribution of death rates by age. This happens to be for Japan a few years ago. And again, females do better than males. The gold curve in the middle is the average of the two.
And this is very typical of almost any country that you look at. The shape of this curve is pretty universal. So what does this say? It says that when you are born, there is a relatively high risk that you're going to die.
So these are kids who have congenital abnormalities, have prenatal problems, have all kinds of difficulties. And they don't make it. So there's a fairly high death rate at birth. But once you make it to, I think, about two years old, the death rate is down to about one in 10,000 per year. Right.
And then it stays quite low until you become a teenager. Now why might the death rate go up when you become a teenager? Well, suicide is the extreme example of that. But teenagers tend to be risk seeking rather than risk averse.
You know, they start driving cars. They go skiing, skydiving, whatever it is that they're doing. And they start dying. But then if you make it to about 20, then there is a relatively flat region where by then you've developed enough sense to know what risks are worth taking and which ones aren't.
And so it's relatively flat until about age 35 or 40 at which point it starts inexorably rising. And of course, as you get older and older, the probability that you're going to die in the next year becomes higher and higher. Right. This is uncomfortable for somebody with my amount of gray hair.
Now there is a peculiarity in Japan which people puzzled over for a while. And that is that weird dip up at age 106. So first of all, that's a very small number of people that that represents.
And it turned out that it was fraud. So there were families who failed to report the death of their ancient grandmother or great-grandmother because they wanted to continue collecting social security payments from the government. So that's an artifact.
OK. Now this is a serious problem which we're going to return to in a more technical way later in the semester, which is this problem of disparities. So if you look at, for example, the difference between white and black female life expectancy in the United States, you see that everybody's life expectancy, as we've shown, is going up gradually in this case from 1975 to 2015.
But there continues to be a gap between black and white females and between black and white males where black patients are more likely to die or less likely to survive longer given the disparities that exist socioeconomically. Maybe medically. We don't know exactly.
And then if you look at Hispanics, however, they do pretty well. So in 2015, you're actually a little bit better off to be Hispanic, either male or female, than you are to be either white or black. But it's still worse to be black than to be white or Hispanic. Right. So these are the kinds of facts that drive some of the issues in what we do in medical care.
Now what do people die of? Well, about a quarter of them die of heart disease. And a little over a fifth of them die of cancer. This is USA data from 2014 so it's not completely up to date but hasn't changed that much.
And then there's a decreasing number of deaths from various other causes. So heart disease, cancer, or chronic lower respiratory disease. So this is like COPD that's caused by smoking, stuff like that. Accidents account for about 5% of deaths.
Stroke, cerebral vascular events, Alzheimer's disease, diabetes, influenza, pneumonia, kidney disease, suicide, and then everything else is about another quarter. OK. Now take a look at those. What kind of diseases are these, the biggies?
Well. They're chronic. Most of them are chronic. They're also not infectious. But except for influenza and pneumonia, nothing else there is infectious as far as we know. I mean, yeah. That asterisk you should put after every statement about current medicine.
So that's interesting, because if you wrote the same table back in 1850, you would find that a lot of people were dying of infections. And they weren't typically living long enough to develop these lovely chronic diseases of the aging. So there have been big changes there.
Now the other thing that's worth looking at is, in addition to the reasons that people die, they start getting sicker. And they are getting sort of less value out of life because they're developing all these other conditions. So if you look at people over 65, about half of them have some form of arthritis.
And about 40%-- yeah. About 40% have hypertension. By the way, if you have trouble with any of the medicalese words, just interrupt. Hypertension is high blood pressure. Hearing impairment. Me, I'm wearing a hearing aid on one side because my ears are going bad.
Heart disease, about a quarter. Orthostatic impairment-- that means people who wobble because their sense of balance is not so good-- 16%. Cataracts, chronic sinusitis, visual impairment, genitourinary problems, diabetes, et cetera. So these are all growing.
Here's the list of the next 10. And varicose veins, hernia, hemorrhoids, psoriasis, hardening of the arteries, tinnitus, corns, calluses, constipation, hay fever, and cerebral vascular problems. All right. So people develop these by the time they're over 65.
So one question we might ask is, well, what is the quality of life? So for example, a lot of the doctors that I started working with in the 1970s were great advocates of the application of decision analysis decision theory to making medical decisions.
And so the problem is how do you evaluate an outcome? And they said, well, the way we evaluate an outcome is we look at your longevity. Obviously, the longer you live, the better typically.
But we also look at your quality of life during that time. And we say that if you're confined to a wheelchair, let's say, your quality of life might not be as good as if you were able to run around, or if you're suffering from chronic pain, your quality of life might not be as good as if you were pain free.
And so we came up with this model that says, well, the value of your life is essentially an integral from time zero to however long you're going to live of a function Q that says this is a measure of how good your quality of life is at that particular point in time and then some discount factor. Right.
So what's the role of the discount factor? Well, it's just like in economics. If I offer you some horribly painful thing today versus 10 years from now, which are you going to choose? Most of us will say later. So that's what the discount factor does.
Now who knows what the right discount rate is? So in some of their work, they were doing crazy things like taking the financial discount factors about bank interest rates and things like that and applying them to these health things just because they didn't have any better numbers to do. That seems a little suspicious. But nevertheless, methodologically, it's a way of doing it.
OK. So how do you measure the quality of life? Well, there is this notion of the activities of daily living. So can you bathe and shower? Can you brush your teeth and groom your hair? Can you get dressed? Can you go to the toilet, clean yourself up? Are you able to walk, get in and out of bed, get in and out of a chair? Can you feed yourself?
And then there are a bunch of instrumental factors, things like are you able to clean your house and can you manage your money and so on. So these are typically for older people. But they are ways of trying to quantify that quality of life by saying how many of these things are you able to do.
And there are a lot of federal regulations, for example, that take advantage of quantification like this. So if you're asking to be put on some sort of disability where the government sends you a check to keep you alive, you have to demonstrate that you are at a certain point on the scale that's derived from these capabilities in order to justifiably get that.
So occupational therapy is one of the things that people try to teach the elderly. My parents died in their late 80s and my dad was 90. And I remember when he would have some medical problem, then he would be put into the clutches of an occupational therapist who would try to make sure that he was able to communicate, and get around, and not fall for tricks where people wanted to get him to send all his money to them, or meal preparation and stuff like that.
So these are sort of the-- occupational is a funny term for it, because this typically applies to people who are retired so it's not really an occupation. But it's the sort of things that you need to do in order to be able to have a decent life.
Well, now there's an interesting valuation question. So if you look at the top right model, we actually don't have very good data on anything other than mortality. So mortality is who dies. So the blue curve there is the curve that you've seen before, which is, of a cohort, how many people are still alive after a certain number of years?
The red curve is a morbidity curve which says how many of those people are still alive and have no sort of problematic chronic diseases. So they're not in constant pain. And they're not immobilized. And they're not unable to do the things that I just listed on the previous slides.
And then disability is when you really become incapable of taking care of yourself. And it typically involves moving into an assisted living facility, or a nursing home, or something like that, which is kind of a nightmare for many people and also very expensive for society.
So as I said, the blue curve there is based on actual data for American females in 1980. The red curve is a hypothetical curve where I just assumed that the rate of developing a morbidity is about twice the rate of dying.
And the green curve I assumed that the rate of developing a disability is-- I can't remember-- I think three times as high as the rate of dying, something like that. So that's why those curves are lower. And they look approximately right. But we don't have good data on those.
Now the question that you have to ask is, how do you want to change this? So for example, suppose that we kept the same situation. We reduced mortality to 20% of its actual rate. But we kept the disability and morbidity rates the same as they are on the top left.
So what would that do? Well, what that would do is create a huge number of people who are disabled because they're going to live longer beyond the point where they're able to function fully. So this is-- yeah.
AUDIENCE: Can I just ask, why does green not just mean healthy? To me, it just seems--
PETER SZOLOVITS: Green just means healthy.
AUDIENCE: OK. It does.
PETER SZOLOVITS: Yeah. So beyond green is what I meant is the morbidity curve. And beyond red is the disability curve. OK. I may have misspoken.
So green is healthy. Red means suffering from some morbidity. And blue means disabled. So if we just extend life but we don't make it any better, then that's not a very attractive picture. So other possibilities are compression of morbidity.
So for example, if we reduce the rate at which people get sick and die but we increase it-- we decrease it initially and then increase it so that, on average, people live about the same length of time that they now do, then we're going to have fewer people who are suffering from morbidity or who are disabled because you last doing well and then you die.
So this is the wonderful one horse shay where everything falls apart at once, which, you know, frankly, as somebody who's a little closer to the end than you guys are, I wouldn't mind that kind of exit. Right. I don't want to be disabled for 20 years. I'd rather live a long time healthy and then drop dead at some point. OK.
My dad used to say that he wanted to die by being hit by a meteor. Right. He wouldn't know it's coming. It's instant. No suffering, no pain. Perfect. He almost got it but not quite.
OK. And then the final story is lifespan extension, which is where we simply lower the mortality rate and all the other rates in proportion. And what happens is that you start having healthy 107-year-olds and not so healthy 120-year-olds in the population in larger numbers than we do now.
OK. Social quality of life. That's a tough one. All right. So here's a naive theory. We take the quality of life of every person on the planet and we sum them up. And we say, OK, that's the social quality of life.
Is that a good idea? Probably not. Right. For one thing, it would say that we ought to have as much population explosion as possible, because then we'd have more people to integrate over, which doesn't seem sensible. Right.
Now obviously if we start packing the world so that it's super crowded, then the quality of life will go down eventually enough that adding more people is probably not optimal. But nevertheless, that doesn't seem like a real satisfying solution.
How about less? It was popular about 10 years ago for people to write these sort of speculative books about how would the world look if half the people died. Right. Other than the trauma of the half the people dying, you know, they were proposing that this would be some wonderful sylvan sort of ideal old fashioned kind of world.
I didn't buy that. And of course, we don't know of a good way to get there, although it is true that in at least developed countries, birth rates keep falling to the point where populations are worried about underpopulation.
The Japanese, for example, have very strict immigration policies so you can't become Japanese if you're not born Japanese. And Japanese people aren't having enough kids to replace themselves. And so the natural population of Japan is falling.
Italy is in the same quandary except that Italy has all these immigrants coming in and trying to become Italians. And of course, this leads to big political fights about who gets to be an Italian and who doesn't. And then, of course, there's the question of money, which as I say we'll return to later.
Now one other important thing to consider is that because of the increase in life expectancy, there has been a big change in timescale in the way people think about medical care. So it used to be long, long ago in the shaman era, you wouldn't go to a shaman to say keep me healthy.
You would go to a shaman saying, you know, I broke my arm, or I have this pain in my leg, or fix me somehow. And so things were focused on the notion of cure. And that was applicable to acute illnesses.
But as we've gotten better at treating acute illnesses-- which, by the way, didn't happen all that long ago. I mean antibiotics were only invented in the early 20th century. And that made a huge difference in stopping people from dying of infections. So then it became more a matter of managing long term chronic illnesses.
And that's pretty much where we are now. The medical world at the moment, most of the action is in trying to understand things like diabetes, and heart disease, and cancer, and things like that that develop over a long time. And they don't kill you instantly like infectious diseases did. But they produce a real burden.
And then, of course, the next step that everybody expects is, well, how do we prevent disease? So how can we change your exposures? How can we change your motivation? How can we change your diet? How can we change whatever it is that we need to change? How can we change your genes to prevent you from developing these diseases in the first place? And that's sort of the future.
OK. So that's about what medicine tries to do. But how does it do it? And so we're going to talk a little bit about the traditional tasks that are attributed to health care practice.
So traditionally, people talk about diagnosis, prognosis, and therapy. So diagnosis-- I go to my doctor. I say, doc, I've got this horrible headache. I've had it for two weeks. What's wrong with me? And his job-- in my case, it happens to be a "he." His job is to come up with an answer. What's wrong with me?
Prognosis is he's supposed to predict what's going to happen to me, at least if he doesn't do anything. So is this headache going to go away or is it going to turn out to be a brain tumor that will kill me, or is it going to be some amoeba that's living in my brain and eat my neurons? All kinds of horrible things are possible. So it's the prospect of recovery as anticipated from the usual course of disease or peculiarities of the case.
And then therapy, of course, is what do you do about it. And prognosis is definitely informed by diagnosis, because if you don't know what's wrong with me, then it's much harder to predict what's going to happen to me. And if you can't predict what's going to happen to me, then it's much harder to figure out what to do to prevent that from happening or to encourage that to happen, right?
So this is kind of a serial process. And the way I look at it is that there's a kind of cyclic process of care. And the process starts with an initial presentation.
So I show up at my doctor's office and I complain about something. And if you ever listen to a doctor interacting with a patient, the first time the patient comes in, the first question is always, what brought you here? Right? And that's called the presenting complaint.
So if I say, you know, my ankle hurts like hell, that's very different than if I say, I stopped being able to hear in my right ear, or I have this horrible skin rash on my arm, or whatever. And that's going to take me in very different directions.
So then what happens is that the doctor examines you and generates a bunch of data. So these are measurements. And of course, it used to be that those measurements were based on observation.
So there are very famous doctors from 100 years ago who were spectacularly good at being able to look at a patient and figure out what's wrong with them by being very astute observers-- the Sherlock Holmes kind of subtle, oh, I see that you have a cut on the inside of your shoe, which means you must have been going through brambles, and you know, whatever. I'm making up a Sherlock Holmes story here. So that generates data.
And then we interpret that data to generate some kind of information or interpreted data about the patient. And based on that, we determine a diagnosis.
Now, do we determine a diagnosis? Maybe not. Maybe we guess a diagnosis. One of the things I learned early on working in this field is that doctors are actually quite willing to make guesses, because it's so useful to believe that you understand what's going on.
If you say, well, there's some probability distribution over a vast number of possible things, that doesn't give you very good guidance on what to do next. Whereas if you can say, oh, I think this patient is developing type 2 diabetes, then you're locked into a set of questions and a set of approaches that you might try.
Now, when we come back to looking at machine learning, machines don't have the same limitations as people. And so for a machine to integrate over a vast number of possibilities is not difficult. But for a human cognition to do that is very hard. And so this is actually an important characteristic of the way doctors think about diagnostic reasoning.
So then, having made a diagnosis or made a guess, they plan some kind of therapy. They apply that therapy, and then they wait awhile and they see what happened. So if your diagnosis led you to a choice of therapy, you gave that therapy to the patient and the patient got better, then you say, well, it must have been the right diagnosis.
If the patient didn't get better, then you say, well, how did what happened to the patient differ from what I expected to happen to the patient? And that drives your revision of this whole process.
So we again examine what happened as a result of the therapy. We gather more data. We interpret it. We come up with the revised diagnostic hypothesis. We come up with a revised therapeutic plan, and we keep going around the cycle.
Now, that cycle happens very quickly if you're a hospitalized patient, because you're there all the time. You're available. They're trying to do things to you constantly. And so this cycle happens on the order of hours or a day, whereas if you were an outpatient, then you're not dealing with some urgent problem. It may happen over a much slower period.
It may be that your doctor says, well, we're going to adjust your drug dose and see if that helps bring down your cholesterol, or manage your pain, or whatever it is that he's trying to do. Or worse yet, we're going to try to convince you to eat more healthy food, and six months later we'll see if your hemoglobin A1C came down, that you're less close to being diabetic.
So the time scale is very different. But that process of continually reinterpreting things is a really critical feature, I think, of all of medical care.
And if you look back, Alan Turing actually talked, in the early 1950s, about health care as being one of the interesting application areas of artificial intelligence. Why? Well, because it was an important topic. And he had the vision that says, as we start getting more data about health care, we're going to be able to build the kinds of models that we're going to be talking about in this class.
But a lot of the early work took a kind of one-shot approach. So they said, well, we're going to solve the diagnostic problem. So we're going to take a snapshot of a patient, all their data at a particular moment. We're going to feed it into an algorithm. It'll come up with a diagnosis. We're done.
And that wasn't very useful, because it didn't obey the cyclic nature of the process of providing health care. And so this was a revolution that started, really, around the 1980s when people realized that you have to be in it for the long-run and not for sort of single interactions.
OK, well, this is just some definitions of these care processes. So here I've listed some ideas that came from a 1976 paper by several of my colleagues, who said, well, here's a cognitive theory of diagnosis. From the initial complaints, guess a suitable hypothesis. Use the current active hypotheses to guide questioning-- so to order more tests, to ask questions of the patient. And it's the failure to satisfy expectations that's the strongest clue to how to develop a better hypothesis.
And then the hypotheses could be in an activated, deactivated, confirmed, or rejected state. They actually built a computer program that implemented this theory of diagnostic reasoning. And these rules, essentially, about whether to activate, deactivate, confirm, or reject something could be based both on logical criteria and on a kind of very bad probabilistic model.
So it was very bad, because what they really needed was Bayesian networks. And those were about a decade in the future at that point. So they and every other system built in the 1970s had really horrible probabilistic models, because we didn't understand how to do it correctly.
Now, what's interesting is somebody noticed that if you strip away the medicine from this, this is kind of like the scientific method, right? If you're trying to understand something, you form a hypothesis. You perform an experiment. If the experiment is consistent with your expectations, then you go on and you've gotten a little bit more confident in your hypothesis.
If your experiment is inconsistent with your expectations, then you have to change your theory, change your hypothesis. You go back and gather more data, and then keep doing this until you're satisfied that you've come up with an adequate theory.
So this was a surprise to doctors, because they thought of themselves more as artists than as scientists. But in a way, they act like scientists, which is kind of cool.
All right, this doesn't stop with caring for a single patient. So we have all these meta-level processes about the acquisition and the application of knowledge about education, quality control and process improvement, cost containment, and developing references.
So this is a picture from David Margulies, who was chief information officer at Children's Hospital here. And the cycle that I described is the one right here. This is the care team taking care of a patient. But of course at some point, that patient is discharged. And then they're in community care and self-care, and then maybe they're in some sort of active health status management.
And then if that goes badly, then there's some episode where they reconnect to the health care system. They get authorized to come back. They get scheduled for a visit, and they're back in the cycle.
And so the process of care involves people going to the hospital, getting taken care of, they get better, they get discharged, they live their lives for a while. Maybe they get sick again. They come back. And so there's this larger cycle around that issue.
And then around that are all kinds of things about health plan design and membership and what coverage you have and so on. And then I would add one more idea, which is that if you have a system like this, you actually want to study, at the next meta-level, that system, make observations about it, analyze it, model it, plan some improvements, and then intervene in the system and observe how it's working and try to make it better.
So in terms of tasks that are important for us in this class, this class of tasks is central, because one of the things we're trying to do is to look at the way health care works and to figure out how to make it better by examining its operation. And that can be done at any of these three levels.
It can be done in the more acute phase, where we're dealing with somebody who's in the middle of an illness. It can be done at the larger phase of somebody who's going through the cycle of being well for a while and then being sick, and then being well again and being sick again. And it can be in terms of the system itself, of how do you design a health care system that works better for the population?
So this notion of a learning health care system is now a journal. So in 2017, Chuck Friedman at the University of Michigan started this new journal. And it's full of articles about this third level of external cycle.
So how does the health care system learn? Well, I'll tell you an anecdote. In the mid-1980s, I was teaching an AI expert systems course. And I had just come back from a conference of medical informatics people, where they were talking about this great new idea called evidence-based medicine.
And I remember mentioning this to a bunch of MIT engineering students. And one of them raised his hand and said, as opposed to what? Right? I mean, to an engineer, it's obvious that evidence is the basis on which you analyze things and make things better. But it wasn't obvious to doctors. And so this was almost a revolutionary change.
And the idea that they fostered was the idea of the randomized controlled clinical trial. So I'm going to sketch what that looks like. Of course, there are many variations. But suppose that I'm one of the drug companies around Kendall Square, and I come up with a new drug and I want to prove that it's more effective for condition X than some existing drug B.
So what do I do? I find some patients who are suffering from X and I try very hard to find patients who are not suffering from anything else. I want the purest case possible.
I then go to my statisticians and I say, let's design an experiment where we're going to collect a standard set of data about all these patients. And then we're going to give some of Drug A and some of them Drug B, and we'll see which of them do better. And we'll pre-define what we mean by do better. So like, not dying is considered doing better, or not suffering some bad thing that people are suffering from is considered doing better.
And then the statisticians also will tell me, given that you expect Drug A to be, let's say, 10% better than Drug B, how many patients do you have to enroll in this trial in order to be likely to get a statistically significant answer to that question? And then they do it.
The statisticians analyze the data. Hopefully you've gotten p less than 0.05. You go to the Food and Drug Administration and say, give me permission to market this drug as the hottest new cure for something or other, and then you make billions of dollars. Right? This is the standard way that pharma works.
Now, there are some problems. So most of the cases to which the results of a trial like this are applied wouldn't have qualified to be in the trial. For example, we talked about morbidities, about the chronic problems that people have.
Well, if you're dealing with one disease, you want to make sure that those populations you're dealing with don't have any of these other diseases. But in the real world, people do. And so we've never actually measured what happens to those people if you give them this drug and they have these comorbidities.
The other problem is that the drug company wants to start making these billions of dollars as soon as possible. And so they want the trial to be as short as possible. And they want it to be as small a sample as they need to get that 0.05 statistical significance.
So these are all problematic, and they lead to real problems. So I didn't bring any examples, but there are plenty of stories where the FDA has approved some drug on this basis, and then later they discovered that although the drug works well in the short-term, it has horrible side effects in the long-term, or that it has interactions with other diseases where it doesn't work effectively for people except for these pure cases that it was tested on.
So the other idea, the competing idea is to say, let's build this learning health care system in which progress in science, informatics-- whatever-- generates new knowledge as an ongoing natural byproduct of the care experience and we seamlessly refine and deliver best practices for continuous improvement in health and health care. Wonderful words from the Institute of Medicine, now called the National Academy of Medicine.
But it's hard to do this. And the reason it's hard to do this is mainly for a very profound underlying reason, which is that people are not treated by experimental protocols. So it's very important in that sketch that I gave you of the Drug A versus Drug B that there is a randomization step where I flip a coin to decide which drug any particular individual is going to get.
If I allow that decision to be biased by my expectations or by something else I know about the patient, then I'm no longer doing a fair trial. And of course, when I collect data about how actual patients are being treated, they're being treated according to what their doctors think is best for them, and so there is no randomization.
I mean, if I went to Mass General and said, could you guys please treat everybody randomly so that we collect really good data, they would throw me out properly.
OK, so we also need a whole lot of technical infrastructure. We need to capture all kinds of novel data sources, which we'll talk about in the next lecture. And then we need a technical infrastructure for truly big data.
So just for example, Dana Farber started about five years ago-- it's a cancer hospital. And so for every solid tumor, they would take samples of the tumor and genotype it-- multiple samples, because tumors are not uniform. So just storing that stuff is a technical challenge, and being able to come up with it.
You've got three gigabases, so about over a gigabyte of data-- from each sample, from each tumor, times all the people who come in and have this test. So you buy some big disk drives or you farm it out to Google or something. But then you need to organize it in some way so that it's usefully easy to find that data.
So today's technique, today's prejudice is what I call the meat grinder story, which is you take medical records, genetic data, environmental data, data from wearables, you put them into an old-fashioned meat grinder, and out come bits, which you store on a disk. And then you have all the data from which you can build models. And that's what we do. You're going to see a lot of that in this course.
OK, the other thing that medicine tries to do is not to cure people but to keep them healthy. And this has been pretty much the domain of the public health infrastructure. So if you go across the river to the Harvard Medical area, there are a couple of big buildings, which is the Harvard School of Public Health, and this is what they're all about.
So they do things like tracking disease prevalence and tracking infections, and worrying about quarantining people. They also do a lot of the kind of work we're going to talk about in this class, which is modeling in order to try to understand what's going on in individual's health, in the health of a population, in the operations of a health care system. So they're very much into this.
Now historically, I looked back and it turns out there's something called the London Bills of Mortality in the 17th century, started by a gentleman named John Graunt. And he was interested just in figuring out, how long do people live? And so he came up with these bills of mortality, where he went around to different parts of London, talked to undertakers and hospitals and whatever health care providers existed at the time, and collected data on what people died and how many people were living in that area.
And so for example, he estimated that the mortality before age six in the 17th century-- this was a long time ago, 1600s-- was about 36%. So if you were a kid, your chances of making it to age six were only about 64%, so less than 2/3. Kind of shocking.
In the 18th century, people you've never heard of-- and Linnaeus, who you probably have heard of, because he was one of the early taxonomists for biological and animal species and so on-- made the first attempts at systemic classification. In the mid-1850s, mid-1800s, there was a Congress of the First International Statistical Congress.
And a gentleman named William Farr came up with an interesting categorization that said, well, if we're going to taxonomize the diseases, we should separate epidemic diseases from constitutional diseases from local diseases, whereby "local" he means affecting a particular part of the body from developmental diseases.
So this is things like stunted growth or failure of mental development or speech development, and then diseases that are the direct result of violence. So this is things like broken bones and bar fight results and stuff like that. So that was the first classification of disease in about 1853.
Note, by the way, that this is before Louis Pasteur and his theory of the germ cause of disease. And so this was a pretty early attempt, and obviously could have benefited from what Pasteur later discovered.
So by the 1890s, which is post-Pasteur, they came up with a classification that was a hierarchic classification, 44 top-level hierarchies broken up into 99 lower-level categories and 161 particular titles. And they adopted this as a way of getting, typically, mortality data of what was it that people were dying of.
And by the 1920s, you've heard of ICD-- ICD-9, ICD-10. So this is currently used as a way of classifying diseases and disorders. The International List of the Causes of Death was the first ICD back in, I think, the 1920s. And then it kept developing through multiple versions. In 1975, ICD-9 was adopted. In 2015, ICD-10. And these are under the control of the World Health Organization, which is now a UN agency, although I think it predates the UN actually.
And then we have ICD-9 CM and ICD-10 CM, are US Clinical Modifications that are an extension of the ICD-9 and 10 coding. And they are primarily used for billing. But they're also used for epidemiological research.
And if you look at the Centers for Disease Control, CDC, they collect death certificates like this from all over the country. And so this is a person who died of a cerebral hemorrhage which was due to nephritis, which was due to cirrhosis of the liver. And so you can use this kind of data to say, well, here's the immediate cause of death, here's sort of the proximate cause of death, and here's the underlying cause of death. And so this is the sort of statistical data that we now have available.
Now, do any of you watch the PBS show Victoria? Nobody? You're not television watchers. All right, that's pretty cool. I was stunned, because as I was preparing this lecture and I had the next slide, it turns out this plays a role in one of the episodes that was broadcast about a week and a half ago.
So in the 1850s, there was a big outbreak of cholera in London. And John Snow was a doctor who did this amazing epidemiological study to try to figure out what was causing cholera. The accepted opinion was that cholera was caused by miasma. What's a miasma?
STUDENT: Bad air.
PETER SZOLOVITS: Bad air, OK? So it's bad air. Somehow, bad air was causing people to get sick and die. And several hundred people died. Whereas Snow started plotting on a map of the area in London where these were concentrated where everybody lived.
And what he discovered, interestingly enough, is that right in the middle of Broad Street, which is pretty much at the epicenter of all these people dying, was a water pump that everybody in the neighborhood used. And that water pump, its supply had become infected by cholera. And so people were pumping water, taking it home, drinking it, and then dying, or at least getting very sick.
And he looked at this and he said, well, if we turn off that pump, the epidemic will stop. And he actually went to the queen, Queen Victoria-- hence the tie-in to the television show-- and convinced her that this was worth trying, because they didn't have any better ideas. And they took the pump handle off the pump, and sure enough the cholera epidemic abated.
Now, of course the underlying problem was sanitation. And they didn't fix that. That took longer.
But what's interesting is here is a 2003 study of the spread of West Nile virus. So this is mosquitoes that are biting people and infecting them with this nasty disease. And they actually used very similar techniques to figure out that maybe this was coming in on airplanes through JFK. So mosquitoes were hitching a ride on an airplane and coming into the US. We need to build a border wall against mosquitoes.
There is also a very controversial practice that used to be used a lot by public health officials, which was to quarantine people. And so there are lots of examples. Anybody's relatives come through Ellis Island? Must be a few.
OK, so they were subject to quarantine. If you were sick when you arrived at Ellis Island and they didn't know exactly how sick you were, they would put you in a building and wait a month and see if you got better or if you got worse, and then decide whether to send you back or to let you in. So that was a pretty common practice.
There's a famous story of Typhoid Mary, who was a carrier of typhoid fever but was not herself affected. Unfortunately, she was a cook. And wherever she was employed, people got really sick. And eventually, the New York Department of Health forcibly essentially jailed her in some sanatorium in order to keep her from continuing to infect people-- it was a very controversial case, as you might imagine.
You don't have to go that far back. Here's a 1987 article from UPI from The Chicago Tribune. So Jesse Helms, who was a senator, was calling for everybody who has AIDS to be quarantined. So fortunately, that didn't happen. But the idea is still around, to say, well, we're going to stop this infection by quarantining people.
And then here's a recent report about the Ebola response in Africa over the past few years, when Ebola was ravaging parts of that continent. And their conclusions are it's controversial, a debated issue, significant risks related to human rights. Quarantine should be used as a last resort. Quarantines in urban areas are really hard. Mobile populations make it hard.
And this is the most technical conclusion, that if you're going to quarantine a bunch of people, you have a huge waste disposal problem on your hands. Because if you have people who might have Ebola, you can't just take their garbage and throw it out somewhere. You have to dispose of it properly.
OK, so the last thing I wanted to talk about-- hopefully mercifully short-- will be paying for health care. So I remember reading about 20 years ago that if you bought a Chevy from General Motors, they spent more money on health insurance and health care for their employees than they did on the steel that went into your car. That's pretty amazing.
So why is this? Well, essentially, there's an insatiable demand for health care, right? Nobody wants to die, except for the suicidal people. And so if I'm sick, I want the best care possible, and I want as much of it as possible. Because you know, what is more important in life than continuing to live?
So we also have gotten better at making drugs and better tests, and so on. I remember one MRI machines became popular about 30 years ago. The state of Massachusetts, for example, had a commission that you had to convince them to be allowed to buy an MRI machine for your hospital, because MRI machines were very expensive and MRIs were, at that time, hugely expensive. And so they wanted to contain the cost of health care by limiting the number of such machines.
Well, eventually the costs came down. And so we're doing better. But if you read the newspapers, you see that drug therapy is very expensive. We have these wonder drugs for rare diseases or cancers that cost $1 million a year to pay for your dosage.
And so there is a high human motivation to do this, and not much pushback-- except from insurance companies. But they just pass the cost on in insurance contracts.
There's also waste. So there are lots of stories about half of health care expenses are spent in the last year of somebody's life.
Although, Ingelfinger, who was the editor of The New England Journal, gave a talk here about 25 years ago. And he said, you know, when I was a practicing doctor, no patient ever came into my office saying, doc, I'm in the last year of my life. And so that was a difficult criterion to try to operationalize.
There are marginally useful procedures. The IOM estimated that there are sort of 40,000 to 100,000 quote unquote unnecessary deaths per year-- in other words, deaths that could be avoided by just being more careful and a little bit smarter.
Well, so the result of this is that if you look at health care spending as a percentage of gross domestic product from 1970 to I think 2017, I believe, on this graph, what you see is one real outlier. And that's the United States on top.
So I've selected a few of these just to look at. There's the US on top. France, Germany, a lot of the European countries are roughly at that highest of the crowd level. Canada is down there. The UK is a little lower. Spain is a little lower. Israel is a little lower. Turkey is the lowest of the OECD countries in terms of percentage of GDP that they spend on health care.
So well, that's OK. But maybe we're getting more for our money than these other countries. And so there are a lot of analyses that look at stuff like this. They say, well, if we spend so much money per patient per year, what do we get in terms of the simplest thing to measure, which is life expectancy? How long do people live?
And what we discover is that in the United States, we're spending $9,000 a year on patients and we're getting a life span of somewhere in the high 70s. So this is 2015 data. Whereas in Switzerland, they're spending about $6,000-- so about 2/3-- and they're getting 83 years or something of life for the same price, and so on for all these different countries.
By the way, this is all from Gapminder, which is a wonderful data visualization tool. And I don't have time to show you, but you can click on individual lines there and slide the slider about what euro you're talking about, and the data moves. And it's beautiful, and it's a wonderful way of understanding it.
So there's an important thing to remember, which was taught to me by my friend Chris Dede at the Harvard Education School. And that is that it's not even good enough to stand still. So he suggested the following scenario.
If you look at the growth of productivity over this period of 10 years by industry, you discover that productivity went up by seven point something percent for durable goods and went down by something like 2% for mining. About 1% for construction. Information technologies grew by 5 and 1/2% or something.
So if you ask the question, what happens if the demand for these goods remains constant over a period of time? And what you discover is that because the more productive things become cheaper, they wind up occupying a smaller fraction of the total amount of money that's being spent.
So your computer, your laptop, is a lot cheaper today than it was 30 years ago. And so that means that the amount of spending that people do on things like information technology, at least per item, is much lower than it used to be. And if in the aggregate it's also lower than it used to be, that means that something else must be higher, right? Because it sums to 100%.
And so what this shows is that if you spend 30 years at the same rates of productivity growth, mining grows from whatever fraction of the economy it was to something that's about three times as big a fraction. Right? And if productivity growth is better, then that sector of the economy shrinks.
So I think something like this is happening in health care, which is that there's infinite demand. And health care is not terribly productive. We're not getting better as fast as electronics is getting better, for example.
So people have tried doing various things. "Managed care" was the buzzword of the 1980s and '90s. And they said, well, what we're going to do is to prevent people from overusing medical services by requiring pre-admission review, continued stay review, second surgical opinion. We're going to have post-care management, where if you're released from the hospital and you're in that second of the cycles, people will call you at home and try to help you out and make sure that you're doing whatever is best to keep you out of the hospital. And
We're going to try various experiments, like institutional arrangements, that say, well, if I as a doctor agree to refer all my patients to Mass General rather than to the Brigham or the BI, they'll pay me extra. And they'll get some kind of efficiencies from aggregation. And so maybe that's one way of controlling costs. So leakage is the idea of keeping people in-system.
And capitation is an interesting idea, which says that instead of paying for what the hospital does for me or what the doctor does for me, we simply pay him a flat fee for the year to take care of me. And that takes away the incentive for him to do more and more and more to get paid more.
But of course, it gives them an incentive to do less and less and less so that he doesn't have to spend as much money. And so it's sort of a knife balance to figure out how to do this. But that's an important component.
So if you look at the evaluation of managed care a long time ago, what they said was it helped reduce inpatient costs by increasing outpatient costs. So what it's done is it's pushed people from going to the hospital into going to their doctor's office. But it's been pretty much a wash in terms of overall spending.
Doctors also hated managed care. I was sitting with one of my colleagues at a Boston hospital, and an insurance company clerk called him to dun him for having ordered a certain test on a heart patient. And so he was furious. And he turns to her and says, so which medical school do you have your diploma from? And of course, she doesn't have a medical degree. She's following some rules on a sheet about how to harass doctors not to order expensive tests.
And so we have Edward Annis, the past president of the AMA, who says, well, in the glory days there was no bureaucratic regimentation, no forms, no blah, blah, blah. And all my patients were happy, and I was happy, and things were ideal.
If you actually look back at those days, it wasn't as good as the cracks it up to be. And some of those issues of disparity that we were talking about were horrible. So for his patients who were rich and who could afford to see him, life was pretty good. But not so much for underserved populations.
So you've seen ObamaCare come and partly go, and continues to be controversial. But it's trying to foster better information technology as a basis for getting doctors to make better decisions. It's trying to foster these accountable care organizations, which is a version of capitation, to put the pressure on to reduce the amount of health services that people are asking for.
There is a hospital readmission reduction program now that says if you are a Medicare patient, for example, and you're discharged from the hospital and you're readmitted within 30 days of your discharge, then they're going to dun you and not pay you for that readmission, or not pay you for part of that readmission. But if you look at the statistics, which I just did, that's the distribution of the payment adjustment factors. So it turns out the lowest number is 97%.
So a 3% decrease in reimbursement is something that the CFO of your hospital would really care about. But it's not like a 25% reduction in reimbursements. So this has had a fairly minor effect.
Let me just finish by saying that money determines much. From our point of view, one of the problems we face is that IT traditionally gets about 1% to 2% of spending in medical centers, whereas it gets about 6% or 7% in business overall and about 10% to 12% for banking.
And a lot of these systems are managed by accountants, although that is slowly changing. So in the 1990s, HST with Harvard started a training program for medical doctors to become medical informaticians-- so practicing this sort of witchcraft. And our first two graduates, one of them is now CIO at the Beth Israel Deaconess. The second one is CIO at Children's Hospital. And so one of my big successes personally has been to displace some accountants by doctors who actually understand this technology to some extent.
OK, I think that's all I'm going to say. There's a funny last slide here, which has a pointer that I would want you to remember. The slides will be up on our website. You can follow it.
MIT has a program called GEMS. It's the General Education in Medical Sciences, intended as a minor program for people in the PhD program in some other field. And if you're serious about really concentrating on health care, developing at least the kind of understanding of the health care process that I've tried to give you today and that would allow you to play a doctor on television is really important. And there is a program that helps you achieve that, which I commend to people.
OK, see you next week.