Flash and JavaScript are required for this feature.
Download the video from Internet Archive.
The susceptible-infected-removed (SIR) epidemiological model of disease spreading in a population, which is a system of coupled nonlinear ODEs. An epidemic will occur when the initial reproductive number is greater than unity.
Speaker: Professor Martin Z. Bazant
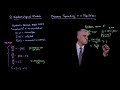
Video 3-1: Epidemiological ...
PROFESSOR: So now, let's begin talking about the spread of disease, initially focusing on a population of individuals.
So the type of modeling, which we'll be discussing, is sometimes referred to as compartmental modeling because a population is divided into different compartments.
Such as, for example, a set of susceptible people that have yet not been infected, a set of infected people, and finally a compartment of recovered people.
Additional compartments could be added, for example, for different age groups or sub-populations, for incubation prior to infection by the disease, and, for example, death and separated from recovery.
This type of modeling based on population compartments was introduced by Kermack and McKendrick in the simplest case of what is called the SIR model, where S is the number of susceptible, I number infected, and R the number of recovered individuals in a population.
And the dynamics take a simple form of a set of coupled nonlinear Ordinary Differential Equations.
So first of all, the rate of change of the number of susceptible people is minus the transmission rate beta times S times I because SI base refers to how many pairs of susceptible and individual, or infected persons there are, and beta is the transmission rate per such individual pair.
And then, next, we look at the dynamics of the number of infected persons.
So that starts by a conversion from susceptible to infected.
So beta*S*I is the rate of producing new infected persons.
And then, we introduce another rate, constant gamma, which is the removal rate.
So this could be people are removed from the infected compartment either by recovering or, potentially, we could lump into that, dying.
So we finally complete the balance here by writing the number of recovered, changing as gamma*I.
So basically, we have a model here with three compartments and two rate constants.
Now the important aspect, really, here is the rate of change of the number of infected individuals.
So I'll write that equation over here, which is dI/dt equals, and let's factor out gamma and write the prefactor here on the rate as (beta*S/gamma-1) times gamma*I.
And if we look now at early times, then the number of susceptible people is approximately equal to the initial number, at t=0.
So that's essentially the size of the entire population, typically.
And so we would then write this as beta*S0/(gamma-1), times gamma*I.
So now this is just a formula that at early times gives you an exponential increase.
So we would then find that I grows like the initial number of infected persons.
It could be, for example, just 1 times e to this factor here times t.
And I'm going to write it in a certain way, which is (R_0-1)*gamma*t.
So this is the early times.
And then we have put a little squiggle here to indicate that's the initial growth rate or the initial dependence, where R_0 is beta*S_0/gamma.
And this is called the reproductive number of the disease, or of the epidemic, because we can see here that if R_0 is bigger than 1, then we have an exponential growth of the number infected persons.
So then we essentially have an epidemic starting from an initial index case, or some set of cases, numbering I_0.
Of course, if R_0 is less than 1, then we have no epidemic.
In other words, there may be an infected person or two, but the number will exponentially decrease, and there won't be any growth.
So the reproductive number is an important concept in epidemiology that comes directly from these models.
Related to that is the concept of herd immunity, which is the point where enough members of the population are immune that the epidemic starts to die out and eventually disappear.
Let's make a plot of the typical predictions of the SIR model.
So as a function of time, the number of susceptible people starts at some value S_0, and it decreases.
Initially, the number of infected persons starts at some small number I_0, which might even just be one index case, and as we showed here, it exponentially increases.
The number of recovered starts at 0 and increases as well, with some delay given by the recovery time.
And what we then see is that as the number of susceptibles comes down-- let's look at this equation here.
We can write this as (beta*S-gamma)*I.
So initially, (beta*S-gamma) is positive.
It starts, in fact, at the value (R_0-1)*gamma.
But eventually, as the number of susceptible people comes down, there's a certain point where this factor goes to 0.
And that would be leading to dI/dt equal to 0.
So, in other words, a maximum of the number of infected people.
So at some point, there's going to be a value where this will turn around.
So dI/dt is equal to zero.
And where that happens will be at a certain value of S_0, which we'll just put-- of S, I should say.
I'll call that S_h for the value of herd immunity in the susceptible number.
And that is when this factor beta*S-gamma is equal to 0.
Or in other words, S_h is gamma/beta.
OK, and once we get to that point, then now dI/dt is going to change sign and will only be negative.
So the number infected will only be decreasing.
Notice S is strictly decreasing because this is a negative rate here.
So S only continues to go down, which means that the prefactor here is always negative.
So the number of infected people now, at this point, must also necessarily continue to go down, and ultimately the number of susceptibles will, from that point, tend towards 0.
The number of recovered will tend, of course, with some lag to S_0.
So this is the number of recovered.
This is the number of susceptible.
And the number of infected, of course, also goes to 0, something like this.
And ultimately, in the long run, the decay-- because S is going to 0, dI/dt is minus gamma*I*t.
So the final rate of drop here is -gamma*t.
So basically, the recovery rate is really dominating how quickly people are being converted, basically, from, to create the recovered and remove the infected population.
So the final result here that comes from these models, which is quite interesting, is to ask, what is this fraction of the population that needs to become immune, or what is this sort of threshold of susceptible in order to achieve herd immunity?
So if we look at what is this S_h over the initial?
So how far do we have to go down?
Well, that would be gamma/(beta*S_0), and you'll recognize that that is nothing more than the inverse of the initial reproductive number.
So essentially, herd immunity is reached at a value of the susceptible fraction becoming 1/R_0.
And this is an interesting prediction of this very simple model, which is that more infectious diseases that have a very high value of R_0, for example, smallpox, leads to a situation that, when the epidemic finally ends, the number of susceptibles when you've reached herd immunity, well, when this starts to turn around, is actually quite low.
So, in other words, you have to go very far in infecting the population to start to end the epidemic.
Conversely, a disease that has a small value of R_0, even COVID-19, might be a value of say 3.5, then this fraction here might not be so [low].
It might be, maybe, only say, 20% or 30%.
Where you can start to see then herd immunity being reached and the number of infected people going down dramatically.